AI Agents in Customer Service: Beyond Simple Chatbots
Discover how AI agents are revolutionizing customer service beyond basic chatbots, delivering personalized experiences with advanced capabilities in natural language processing, sentiment analysis, and complex problem-solving.
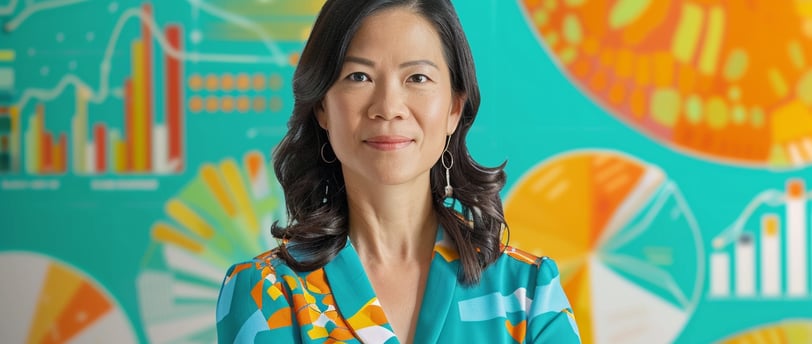
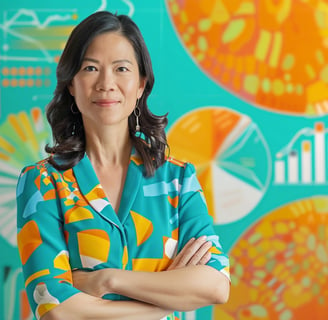
We've all been there—trapped in a frustrating loop with a chatbot that simply doesn't understand our question. "I'm sorry, I don't understand. Could you rephrase that?" The repetitive, unhelpful responses that make us long for human interaction. But what if the future of customer service isn't a choice between limited bots and human agents? What if it's something far more sophisticated? Advanced AI agents are fundamentally transforming the customer service landscape, moving well beyond the rudimentary capabilities of first-generation chatbots. These intelligent systems combine complex reasoning, emotional intelligence, and specialized knowledge to create experiences that customers actually appreciate rather than tolerate. In this comprehensive guide, we'll explore how AI agents are reshaping customer service operations, examine implementation strategies for businesses of all sizes, and look at real-world examples of companies reaping the benefits of this technological evolution.
The Evolution of Customer Service Technology
The journey from traditional call centers to today's AI-powered customer service hubs represents one of the most dramatic technological transformations in business operations. Just two decades ago, customer service primarily meant staffing large call centers with representatives handling inquiries one at a time via telephone. The introduction of email support and basic online FAQ systems marked the first step toward digital customer service, though these systems still relied heavily on human intervention to function effectively. The true revolution began with the advent of rule-based chatbots around 2010, which could respond to simple, predictable queries using predefined scripts and decision trees. These early chatbots represented a significant advancement but were notoriously limited in their capabilities and often led to customer frustration when conversations deviated from anticipated patterns.
The introduction of machine learning to customer service around 2016-2017 marked a critical inflection point in this evolutionary journey. Rather than relying solely on predetermined scripts, these systems could analyze vast datasets of previous customer interactions to improve their responses over time. This shift from purely rule-based to learning-based systems allowed for greater flexibility and accuracy in addressing customer needs. Today's most advanced AI customer service systems harness the power of sophisticated large language models (LLMs), neural networks, and specialized AI technologies that can understand context, recognize sentiment, and even anticipate customer needs before they're explicitly stated.
The transition hasn't been merely technological but has fundamentally shifted the customer service paradigm from reactive problem-solving to proactive experience enhancement. Modern AI agents don't just respond to issues; they actively work to improve customer journeys, predict potential problems, and provide personalized recommendations based on individual preferences and behavior patterns. This evolution continues at a rapid pace, with each advancement bringing us closer to truly seamless, intelligent customer service experiences that combine the best aspects of human empathy with the efficiency and scalability of technology.
From Simple Chatbots to Advanced AI Agents: Defining the Difference
The distinction between simple chatbots and advanced AI agents extends far beyond superficial differences in capability. At their core, traditional chatbots operate on a relatively straightforward premise: match customer inputs to predefined patterns and respond with corresponding scripts. These systems function like digital decision trees, following if-then logic paths to determine how to respond to user queries. While such chatbots can effectively handle basic, frequently asked questions, they quickly reach their limits when faced with complex inquiries, unusual phrasings, or scenarios that weren't explicitly programmed into their response database. The rigid architecture of traditional chatbots means they have no genuine understanding of language or context—they simply perform pattern matching exercises.
Advanced AI agents, by contrast, represent a fundamentally different approach to customer interaction built on sophisticated artificial intelligence technologies. These systems leverage natural language processing (NLP) and natural language understanding (NLU) capabilities to comprehend the true meaning behind customer inquiries rather than simply matching keywords. They can parse complex sentences, understand linguistic nuances, and maintain context throughout multifaceted conversations spanning multiple topics. AI agents also employ sentiment analysis to detect emotional cues in customer communications, allowing them to adapt their tone and responses accordingly—showing empathy when a customer is frustrated or congratulatory when they express satisfaction.
Perhaps most significantly, advanced AI agents possess learning capabilities that allow them to continuously improve through each interaction. Unlike static chatbots that can only be improved through manual updates to their programming, AI agents incorporate machine learning algorithms that analyze successful and unsuccessful interactions to refine their approach over time. Many leading systems now incorporate generative AI techniques that enable them to create original, contextually appropriate responses rather than selecting from pre-written templates. This fundamental capacity for growth and adaptation means that AI agents become more valuable assets with each customer interaction, creating a virtuous cycle of continuous improvement that static chatbots simply cannot match.
Key Capabilities of Modern AI Agents
The transformative power of modern AI agents stems from a sophisticated suite of capabilities that extend far beyond simple query-response functionality. Natural language processing (NLP) and understanding (NLU) form the foundation of these systems, enabling them to comprehend customer inquiries with remarkable accuracy regardless of how they're phrased. Unlike rule-based systems that require exact keyword matches, advanced AI agents can interpret the intent behind various expressions of the same question—understanding that "How do I reset my password?" and "Can't log in, need to change password" represent essentially the same inquiry. This linguistic flexibility dramatically reduces customer frustration by eliminating the need to carefully phrase questions according to the system's expectations.
Emotional intelligence represents another critical dimension of advanced AI agents' capabilities. Through sentiment analysis technologies, these systems can detect frustration, satisfaction, confusion, or urgency in customer communications. More sophisticated agents can adapt their communication style based on these emotional signals—becoming more empathetic when customers express distress, more celebratory when they share positive news, and more direct when they indicate time sensitivity. Some cutting-edge systems can even detect subtle emotional shifts throughout a conversation and adjust their approach accordingly. This emotional responsiveness helps bridge the empathy gap that has traditionally differentiated human from automated customer service.
The problem-solving capabilities of advanced AI agents have expanded dramatically in recent years. Today's systems can handle remarkably complex customer service scenarios by drawing on extensive knowledge bases, analyzing previous similar cases, and applying sophisticated reasoning algorithms. When faced with unusual or challenging situations, leading AI agents can break problems down into manageable components, request specific information to clarify ambiguities, and synthesize solutions that address the full complexity of customer needs. Many systems now incorporate decision-making frameworks that allow them to take initiative in resolving issues—proactively offering refunds when appropriate, suggesting alternative products when first choices are unavailable, or escalating to human agents when they recognize scenarios beyond their capabilities.
Personalization capabilities distinguish truly advanced AI agents from their more basic counterparts. By integrating with customer relationship management (CRM) systems and analyzing interaction history, these agents can tailor responses to individual customer preferences, purchase history, and previous service experiences. An AI agent might recognize a returning customer, acknowledge their previous interactions, and customize product recommendations based on their established preferences. Some systems can even adjust their communication style to match customer preferences—being more technical with customers who demonstrate expertise and more explanatory with those who may need additional guidance.
Implementation Strategies for Businesses
Successfully implementing AI agents requires a strategic approach that begins with carefully defining your customer service objectives and identifying the specific pain points that AI can address. Businesses should conduct a thorough audit of their existing customer service infrastructure, analyzing common customer queries, average resolution times, satisfaction metrics, and friction points in the current process. This assessment allows organizations to prioritize AI implementation in areas where it can deliver the greatest immediate impact while identifying aspects of customer service that may still benefit from a human touch. Rather than viewing AI implementation as an all-or-nothing proposition, successful organizations typically adopt a phased approach, starting with specific use cases where AI can demonstrably improve customer experiences.
Selecting the right AI agent technology represents a critical decision point in the implementation journey. This choice involves evaluating whether to build custom solutions, leverage existing platforms, or adopt a hybrid approach. Larger enterprises with unique requirements and sufficient technical resources may benefit from developing proprietary AI systems tailored to their specific needs. However, most organizations find greater success utilizing established AI platforms that offer pre-built capabilities while allowing for customization. The landscape of AI platforms has matured significantly, with options ranging from comprehensive customer service suites to specialized solutions for specific industries or use cases. Evaluation criteria should include natural language processing capabilities, integration options with existing systems, scalability, customization flexibility, and the vendor's track record for continuous improvement.
Integration with existing customer service infrastructure presents both technical and operational challenges that must be addressed for successful implementation. From a technical perspective, AI agents must seamlessly connect with CRM systems, knowledge bases, e-commerce platforms, and other business systems to access the information needed to serve customers effectively. This integration often requires developing APIs, establishing data pipelines, and ensuring security protocols protect sensitive customer information. From an operational standpoint, businesses must define clear workflows governing how AI agents interact with human representatives, including when and how to escalate issues, how customer information passes between systems, and how to maintain consistent service quality across all channels.
Training and optimization represent ongoing commitments rather than one-time implementation tasks. Even the most sophisticated AI agents require initial training with company-specific information, including product details, policies, common customer issues, and brand voice guidelines. This training process typically involves feeding the system with relevant documents, conversation logs, FAQ materials, and other resources that help it understand the business context. Once deployed, continuous optimization becomes essential as businesses analyze AI performance metrics, identify areas for improvement, and regularly update the system with new information. Organizations that achieve the greatest success with AI implementation establish dedicated teams responsible for monitoring agent performance, analyzing conversation logs to identify improvement opportunities, and continuously refining the system to enhance customer experiences.
Measuring ROI and Success Metrics
Quantifying the return on investment from AI agent implementation requires a multidimensional approach that captures both direct cost savings and broader business impacts. The most immediately measurable benefit typically comes from operational efficiency, where businesses can track metrics such as cost per interaction, average handling time, first-contact resolution rates, and agent productivity improvements. Leading organizations have reported cost reductions of 15-70% depending on implementation scope, with the most significant savings coming from reduced staffing requirements and increased throughput of customer inquiries. Beyond these direct savings, AI agents can significantly reduce training costs, as new human agents require less extensive training when AI handles routine inquiries, allowing them to focus on developing skills for complex cases. Infrastructure savings may also accrue as businesses reduce their reliance on extensive physical call centers and associated equipment.
Customer experience metrics provide crucial insight into the effectiveness of AI implementation beyond pure cost considerations. Key indicators include customer satisfaction scores (CSAT), Net Promoter Score (NPS), customer effort scores, and sentiment analysis from interaction transcripts. The most successful implementations show improvements in these metrics over time as AI systems become more sophisticated at addressing customer needs. Organizations should establish baseline measurements before implementation and track changes across different customer segments and interaction types. Leading companies have found that well-implemented AI can increase customer satisfaction by 10-25% while simultaneously reducing customer effort. Particularly telling are measurements of channel switching behavior—effective AI implementations reduce the frequency with which customers abandon automated channels to seek human assistance.
Revenue impact metrics help capture how AI agents contribute to business growth rather than merely cost reduction. These measurements include conversion rates for sales-oriented interactions, cross-sell and upsell effectiveness, customer retention improvements, and lifetime value increases. Advanced analytics can attribute revenue outcomes directly to AI agent interactions, demonstrating how effective automated service influences purchasing decisions. Some organizations have documented 5-15% increases in conversion rates and average order values through personalized AI-driven recommendations. Particularly valuable is measuring the impact on customer churn, as effective service automation can significantly improve retention rates for customers who might otherwise have been lost following negative service experiences.
Operational impact extends beyond financial considerations to encompass how AI agents influence overall business functioning. Metrics in this category include service availability improvements (as AI enables 24/7 support), scalability during peak periods, consistency in policy application, and error reduction rates. Organizations should also measure the impact on human agents, tracking employee satisfaction, retention rates, and productivity metrics. Successful AI implementations typically show improvements in human agent satisfaction as repetitive tasks are automated, allowing staff to focus on more complex and rewarding interactions. Another key operational metric is knowledge utilization—tracking how effectively the organization's collective knowledge is deployed through AI to resolve customer issues consistently.
Real-World Case Studies
Financial services giant Capital One has emerged as a leader in deploying sophisticated AI agents to transform customer service operations. Their virtual assistant, Eno, transcends simple chatbot functionality by combining natural language processing with advanced financial security features and personalized financial guidance. Unlike basic chatbots limited to answering frequent questions, Eno can proactively alert customers to unusual account activity, help users understand complex transactions, and provide tailored financial advice based on spending patterns. The system integrates seamlessly with Capital One's mobile and web platforms while maintaining consistent context across channels. According to company reports, Eno successfully handles over 60% of customer inquiries without human intervention, leading to estimated annual savings exceeding $25 million. More impressively, customer satisfaction scores for AI-resolved inquiries have reached 85%, approaching the rates for human agent interactions. Capital One attributes this success to their methodical implementation approach, which involved extensive testing with focus groups, gradual feature rollout, and continuous refinement based on interaction analysis.
In the e-commerce sector, Sephora's Virtual Artist represents a compelling example of AI agents enhancing customer experience beyond traditional support functions. This sophisticated system combines computer vision, augmented reality, and personalized recommendation algorithms to help customers virtually "try on" makeup products and receive customized beauty advice. The Virtual Artist moves beyond answering questions to actively guiding customers through product discovery, essentially functioning as a digital beauty consultant. Since implementation, Sephora has reported a 11% increase in mobile application engagement time and a 17% lift in conversion rates for customers who use the AI assistant. Perhaps most significantly, the system has generated valuable data insights that inform Sephora's broader business strategy, including product development and inventory management decisions. The company's phased implementation approach involved starting with basic virtual try-on capabilities before gradually expanding to include skincare analysis, personalized beauty routines, and integration with in-store experiences.
Telecommunications provider T-Mobile has successfully deployed an advanced AI agent framework that manages customer interactions across multiple channels while maintaining conversation context. Unlike siloed chatbots that operate independently on different platforms, T-Mobile's system provides consistent experiences whether customers engage via text message, website chat, mobile app, or social media. The system can handle complex troubleshooting scenarios by analyzing device diagnostics, account information, and network status simultaneously. When technical issues are detected, the AI can guide customers through troubleshooting procedures using interactive diagrams and video demonstrations. T-Mobile reports that their AI implementation has reduced call center volume by approximately 30% while decreasing average resolution time by 18 minutes per interaction. Customer feedback indicates particular appreciation for the system's ability to maintain conversation history across channels, eliminating the frustration of repeating information when switching from one platform to another.
Healthcare provider Cleveland Clinic has pioneered the use of AI agents to enhance patient communication and care coordination. Their system helps patients navigate appointment scheduling, insurance verification, medication management, and pre-procedure preparation. What distinguishes their implementation is the sophisticated integration with electronic health records and clinical protocols, allowing their AI to provide personalized guidance based on individual medical histories and treatment plans. The system employs sophisticated natural language understanding to interpret complex medical questions and strict compliance features to ensure all information sharing adheres to HIPAA regulations. Since implementation, Cleveland Clinic has documented a 23% reduction in appointment no-shows and a 15% decrease in readmissions for certain procedures, attributed to improved pre and post-procedure communication. Patient surveys indicate satisfaction rates of 82% for AI-managed interactions, with particularly high ratings for appointment management and medication reminders. The clinic employed a careful implementation strategy involving extensive clinical oversight, gradual feature rollout, and continuous monitoring by medical professionals.
Ethical Considerations and Best Practices
Transparency and setting appropriate customer expectations represent fundamental ethical principles when deploying AI agents in customer service contexts. Customers have a right to know when they're interacting with automated systems rather than human agents, and organizations must be forthright about both the capabilities and limitations of their AI technologies. This transparency extends beyond simply disclosing the presence of AI to include clear communication about how customer data is being used, what information the system can access, and how decisions are made. Leading organizations implement this principle by designing clear introductions when conversations begin, providing straightforward explanations of what the AI can help with, and being honest about when human intervention might be necessary. Businesses should avoid creating false impressions of AI capabilities through overly humanized language or making promises about service levels that the technology cannot consistently deliver.
Data privacy and security considerations take on heightened importance with AI customer service implementations, as these systems necessarily process large volumes of potentially sensitive customer information. Organizations must implement robust data protection frameworks that govern what information AI agents can access, how long data is retained, and how it can be used for system improvement. Particularly important is establishing clear policies regarding conversation transcripts, which may contain personally identifiable information, financial details, or other sensitive data. Best practices include implementing strict data minimization principles (collecting only information essential for service delivery), robust encryption protocols, and clear audit trails documenting data access. Organizations should also provide customers with transparent options to request data deletion and understand how their interaction information may be used for system training or improvement.
Bias mitigation demands rigorous attention throughout the development and operation of AI customer service systems. Without careful design and monitoring, AI agents may inadvertently perpetuate or amplify biases present in training data or decision algorithms. These biases can manifest in various ways, from providing different quality of service to certain demographic groups to recommending products unequally or using language that reflects societal prejudices. Leading organizations address this challenge through diverse training data selection, regular bias audits of system outputs, and establishing specific metrics to track service equality across customer segments. Some implement bias detection algorithms that continuously monitor for problematic patterns in AI responses and flag potential issues for human review. Equally important is creating diverse AI development teams that bring varied perspectives to identifying potential bias problems before they reach customers.
Human oversight and escalation protocols serve as critical guardrails ensuring AI systems enhance rather than degrade the customer experience. Even the most sophisticated AI agents will encounter scenarios beyond their capabilities, and organizations must design thoughtful processes for transitioning these interactions to human representatives. Best practices include establishing clear thresholds for when AI should escalate issues, creating seamless handoff procedures that preserve conversation context, and designing feedback loops that allow human agents to improve AI performance. Progressive organizations implement "human in the loop" approaches where AI systems can request human guidance on complex decisions without fully transferring the interaction. This approach combines the efficiency of automation with human judgment for critical decision points. Equally important is establishing monitoring procedures where quality assurance teams regularly review AI interactions to identify improvement opportunities and address potential issues before they impact large numbers of customers.
Future Trends in AI Customer Service
Multimodal AI represents one of the most promising frontiers in customer service technology, moving beyond text-based interactions to encompass voice, visual, and even gestural communication channels. Advanced systems are increasingly capable of processing and generating multiple forms of media simultaneously—understanding images customers share, analyzing voice for both content and emotional cues, and responding with appropriate combinations of text, images, videos, and voice. This evolution enables dramatically more natural customer interactions, particularly for complex issues that benefit from visual demonstration or where customers struggle to describe problems textually. Forward-thinking companies are already implementing systems that can analyze photos of damaged products, guide customers through repairs using annotated images, or demonstrate product features through automatically generated video clips. As these capabilities mature, the distinction between different customer service channels will increasingly blur, creating seamless experiences regardless of how customers choose to communicate.
Proactive and predictive service delivery represents a fundamental paradigm shift from reactive customer support to anticipatory problem resolution. Next-generation AI agents are increasingly capable of identifying potential issues before customers even experience them by analyzing usage patterns, monitoring product performance data, and recognizing early warning signals. These systems can proactively reach out to customers with solutions rather than waiting for problems to emerge fully. For example, an AI might detect unusual patterns in a customer's internet connectivity and contact them with troubleshooting steps before service degrades completely, or identify that a customer has been struggling with a particular software feature based on usage analytics and offer targeted guidance. This transition from problem-solving to problem prevention dramatically enhances customer experience while reducing overall support volumes. The most sophisticated implementations combine predictive analytics with personalization to determine not just what issues might arise, but how and when to approach different customers about potential solutions.
Specialized domain expertise in AI agents is rapidly advancing beyond general-purpose customer service to deeply knowledgeable systems for specific industries and functions. Rather than broad shallow knowledge, organizations are developing AI with profound expertise in particular domains—financial advisory AI that understands complex investment products, healthcare AI with detailed medical knowledge, or technical support AI with deep understanding of specific software ecosystems. These specialized systems combine general language capabilities with extensive domain-specific knowledge bases, allowing them to engage in expert-level conversations within their area of expertise. The development trajectory suggests AI agents will increasingly resemble specialized professionals rather than general-purpose assistants, enabling automated handling of even highly complex customer scenarios that previously required human experts. This specialization trend also manifests in AI that understands specific customer segments deeply—for instance, systems optimized to serve the unique needs of small business owners versus individual consumers.
Human-AI collaboration models are evolving beyond simple handoffs to create sophisticated partnerships between automated systems and human representatives. In emerging models, AI and humans work simultaneously rather than sequentially, with AI handling information retrieval, initial analysis, and routine components while humans focus on judgment, creativity, and emotional intelligence. These collaborative approaches include AI systems that actively support human agents during live conversations by suggesting responses, retrieving relevant information, and handling administrative tasks while the human maintains the primary customer relationship. Some organizations are implementing "AI co-pilot" models where artificial intelligence works alongside human agents in real-time, transcribing conversations, identifying customer sentiment shifts, suggesting next best actions, and even drafting follow-up communications. This collaborative approach combines the efficiency and consistency of automation with the empathy and judgment of human interaction, potentially offering the best of both worlds for complex customer service scenarios.
Statistics & Tables
As AI customer service continues to advance, understanding the data behind implementation success becomes increasingly important. The following interactive table provides key statistics on AI agent deployment across different industries.
Conclusion
The evolution from simple chatbots to sophisticated AI agents represents one of the most significant transformations in customer service history. As we've explored throughout this article, these advanced systems transcend the limitations of their predecessors by combining natural language understanding, emotional intelligence, personalization capabilities, and sophisticated problem-solving abilities to create genuinely helpful customer interactions. The benefits of successful implementation extend beyond mere cost reduction to encompass improved customer satisfaction, enhanced operational efficiency, and even new revenue generation opportunities. Companies across industries—from financial services and telecommunications to retail and healthcare—are demonstrating that properly deployed AI agents can simultaneously reduce expenses and enhance customer experiences.
The implementation journey, while complex, follows increasingly well-established patterns that organizations can leverage to accelerate their success. By starting with clearly defined objectives, selecting appropriate technologies, ensuring seamless integration with existing systems, and committing to ongoing optimization, businesses of all sizes can realize significant returns on their AI investments. The case studies we've examined illustrate how diverse organizations have navigated this journey successfully, adapting AI capabilities to their specific industry requirements and customer expectations. Perhaps most importantly, responsible implementation that prioritizes ethical considerations—including transparency, data privacy, bias mitigation, and appropriate human oversight—ensures that these technologies enhance rather than undermine customer trust.
Looking ahead, the continued advancement of AI capabilities promises even more sophisticated customer service experiences. Multimodal interactions that combine text, voice, and visual elements will create more natural engagement experiences. Proactive service delivery will increasingly anticipate and address customer needs before problems fully materialize. Specialized domain expertise will enable AI agents to handle increasingly complex scenarios that once required human experts. And refined collaboration models between humans and AI will leverage the unique strengths of each, creating service experiences that are simultaneously efficient and empathetic. As organizations navigate this rapidly evolving landscape, those that thoughtfully implement AI agents while maintaining focus on genuine customer needs will differentiate themselves through service experiences that build lasting customer relationships and drive sustainable business growth.
Frequently Asked Questions
What is the difference between a chatbot and an AI agent?
Chatbots are rule-based systems that follow predefined scripts and decision trees, while AI agents use advanced technologies like natural language processing, machine learning, and sentiment analysis to understand context, learn from interactions, and provide personalized responses. AI agents can handle complex queries, maintain conversation context, and continuously improve their performance.
How much can AI agents reduce customer service costs?
Based on industry data, AI agents can reduce customer service costs by 15-70%, with an average of around 50% for enterprise implementations and 30-40% for small to mid-sized businesses. These savings come from reduced staffing requirements, shorter handling times, higher first-contact resolution rates, and decreased training costs for human agents.
How long does it take to see ROI from AI customer service implementation?
Most businesses achieve ROI within 4-14 months, with enterprise solutions typically seeing returns in 5-9 months and smaller implementations taking 9-14 months. Technology companies tend to see the fastest ROI, often within 4-6 months, while regulated industries like healthcare may take longer due to compliance requirements.
What industries benefit most from AI customer service agents?
While all industries can benefit, telecommunications, technology, and retail have seen the highest ROI from AI agent implementation, with cost reductions of 56-70% and CSAT improvements of 41-53%. This is likely due to these industries handling high volumes of repetitive queries that AI can efficiently address, combined with customers who are generally comfortable with digital interactions.
How does AI customer service impact customer satisfaction?
Well-implemented AI agents have been shown to increase customer satisfaction by 20-53%, with the highest improvements seen in technology and telecommunications sectors. Key factors contributing to satisfaction include faster resolution times, 24/7 availability, consistent service quality, and the ability to handle multiple queries simultaneously without wait times.
What are the main challenges in implementing AI customer service?
Common challenges include integration with existing systems, data preparation and quality issues, employee resistance, setting appropriate customer expectations, and ensuring proper handling of complex or sensitive cases. Organizations also frequently struggle with determining the right balance between automation and human intervention.
How can small businesses implement AI customer service with limited budgets?
Small businesses can start with focused implementations that address specific high-volume query types, utilize cloud-based AI platforms with consumption-based pricing models, implement phased rollouts that prioritize high-impact areas, and leverage pre-trained models that require less customization. Many vendors now offer SMB-specific packages with simplified implementation requirements.
What ethical considerations should businesses address when implementing AI customer service?
Key ethical considerations include transparency about AI use, maintaining data privacy and security, preventing and mitigating algorithmic bias, implementing appropriate human oversight, and ensuring accessibility for all customer segments. Organizations should develop clear guidelines for responsible AI use that align with brand values and regulatory requirements.
How are privacy regulations like GDPR and CCPA affecting AI customer service implementation?
Privacy regulations require careful attention to data collection, storage, processing, and customer consent practices. Organizations must implement data minimization, secure storage, clear consent mechanisms, and processes for handling data access and deletion requests. These regulations have accelerated the development of privacy-preserving AI techniques and comprehensive governance frameworks.
What skills do customer service teams need in an AI-augmented environment?
As AI handles routine queries, human agents increasingly need complex problem-solving abilities, emotional intelligence, technical troubleshooting skills, and the ability to collaborate effectively with AI systems. Teams also need individuals with AI oversight capabilities who can identify improvement opportunities, recognize when systems are underperforming, and help refine AI responses over time.
Additional Resources
The Ultimate Guide to AI in Customer Service - A comprehensive resource covering everything from basic concepts to advanced implementation strategies for organizations at any stage of their AI journey.
Measuring ROI: The Business Case for AI in Customer Experience - Detailed frameworks for calculating the financial impact of AI customer service implementations across different metrics and business models.
Ethical AI in Customer Service: Guidelines for Responsible Implementation - Best practices for addressing transparency, privacy, bias, and human oversight concerns when deploying customer-facing AI systems.
The Future of Work in Customer Service: Human-AI Collaboration Models - Exploration of emerging approaches to combining human and artificial intelligence for optimal customer service outcomes.
Technical Implementation Guide: Integrating AI Agents with Existing Customer Service Infrastructure - Technical considerations and step-by-step guidance for successfully connecting AI systems with CRM, knowledge bases, and other critical systems.