AI-Driven Agriculture: Sustainable Farming and Maxing Yield Potential
Discover how artificial intelligence is transforming agriculture with innovative solutions for sustainable farming practices and yield optimisation. Explore real-world applications, ROI analysis, and the future of smart farming in this comprehensive guide.
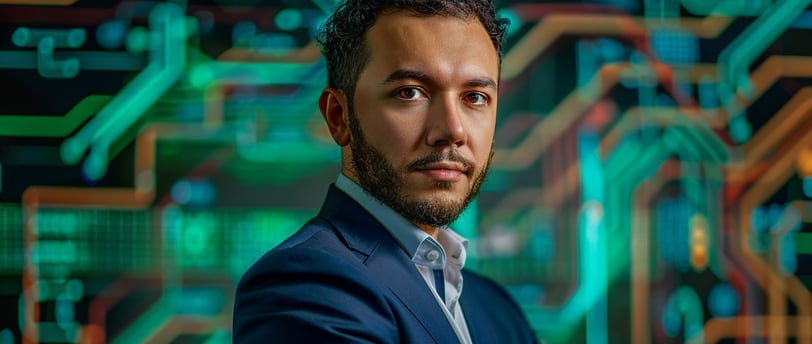
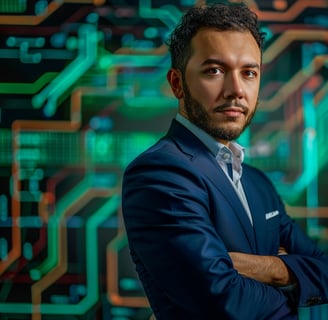
Picture a farm where sensors monitor soil conditions in real-time, drones sweep across fields collecting multispectral imagery, and algorithms predict crop yields with astonishing accuracy. This isn't science fiction—it's the reality of modern agriculture transformed by artificial intelligence. The global agricultural sector faces unprecedented challenges: feeding a growing population projected to reach 9.7 billion by 2050, while contending with climate change, diminishing arable land, and resource scarcity. Traditional farming methods alone cannot meet these demands. Fortunately, AI-driven solutions are emerging as powerful tools to revolutionize farming practices, optimize yields, and promote sustainability. In this article, we'll explore how artificial intelligence is reshaping agriculture, examine real-world applications driving sustainable farming, and analyze the economic and environmental benefits of these technological innovations.
The Evolution of Agricultural Technology
Agriculture has always evolved alongside human technological advancement, from the invention of the plow thousands of years ago to the mechanization of farming in the 20th century. The Green Revolution of the 1950s and 1960s marked a significant turning point, introducing high-yielding crop varieties, synthetic fertilizers, and modern irrigation techniques that dramatically increased global food production. Despite these advances, conventional farming methods often rely on blanket applications of resources like water, fertilizers, and pesticides across entire fields, regardless of variability in soil conditions or crop needs. This approach not only leads to resource wastage but also contributes to environmental degradation through chemical runoff, soil erosion, and greenhouse gas emissions. The emergence of precision agriculture in the 1990s began addressing these issues by using GPS technology to tailor resource application to specific field locations. Now, AI is taking precision farming to unprecedented levels of sophistication and effectiveness.
The integration of artificial intelligence into agriculture represents the next significant leap in farming technology, often called Agriculture 4.0 or smart farming. Unlike previous agricultural revolutions that primarily focused on mechanization and chemistry, this new paradigm harnesses data, connectivity, and intelligent algorithms to optimize every aspect of the farming process. AI systems can analyze vast amounts of data from diverse sources—soil sensors, weather stations, satellite imagery, equipment monitors, and historical records—to generate actionable insights for farmers. These technologies enable more precise decision-making about when to plant, irrigate, fertilize, and harvest crops, helping farmers produce more food with fewer resources. The transition to AI-driven agriculture isn't merely about adopting new tools; it represents a fundamental shift in how we approach food production, prioritizing efficiency, sustainability, and resilience in an increasingly uncertain world.
The journey toward widespread adoption of AI in agriculture has accelerated dramatically in recent years, driven by advances in computing power, sensor technology, data analytics, and machine learning algorithms. Early precision agriculture relied primarily on GPS guidance for tractors and basic variable-rate applications of inputs. Today's AI systems can perform complex tasks like identifying plant diseases from images, predicting weather patterns at the microclimate level, optimizing irrigation schedules based on soil moisture sensors, and even automating harvesting with robotic systems. According to a recent market analysis, the global AI in agriculture market was valued at $1.5 billion in 2022 and is projected to reach $8.4 billion by 2030, growing at a CAGR of 24.1%. This rapid growth reflects both the increasing technological capabilities and the urgent need for more sustainable farming solutions in the face of climate change and resource constraints.
Key AI Technologies Transforming Agriculture
Machine Learning and Predictive Analytics
Machine learning algorithms form the backbone of AI applications in agriculture, enabling systems to identify patterns, make predictions, and continuously improve their performance through experience. These algorithms analyze historical and real-time data to forecast crop yields, predict pest outbreaks, optimize resource use, and support countless other farming decisions. Predictive analytics models can process information about soil conditions, weather patterns, crop health, and market trends to help farmers make more informed choices about planting dates, crop varieties, irrigation schedules, and harvest timing. For example, advanced predictive models developed by companies like Climate Corporation and Farmers Edge integrate multiple data streams to forecast yields months before harvest with remarkable accuracy. These predictions allow farmers to better plan their operations, secure appropriate financing, and make strategic marketing decisions well in advance.
The power of machine learning in agriculture lies in its ability to identify complex relationships between variables that human analysis might miss. By analyzing thousands of data points across multiple growing seasons, these systems can detect subtle patterns that influence crop performance and recommend specific interventions to address potential problems before they affect yields. Supervised learning algorithms can classify plant diseases based on leaf images, identify weed species for targeted treatment, or determine optimal harvesting times based on crop maturity indicators. Unsupervised learning methods excel at finding unknown patterns in agricultural data, helping researchers discover new relationships between growing conditions and crop outcomes. Reinforcement learning, meanwhile, enables systems to optimize sequential decision-making processes like irrigation scheduling or robotic harvesting by learning from the outcomes of previous actions and adapting accordingly.
As machine learning algorithms become more sophisticated and accessible, their applications in agriculture continue to expand. Deep learning, a subset of machine learning using neural networks with multiple layers, has shown particular promise for complex agricultural tasks requiring image recognition or natural language processing. Convolutional neural networks (CNNs) can analyze drone or satellite imagery to assess crop health across vast areas, identifying subtle signs of stress that would be impossible to detect through manual inspection. Recurrent neural networks (RNNs) excel at time-series analysis, making them valuable for predicting seasonal patterns or detecting anomalies in sensor readings from soil monitoring systems. Transfer learning techniques allow models trained on one agricultural problem to be adapted for related tasks, accelerating the development of new applications and making AI more accessible to farmers with limited data resources.
Computer Vision and Image Recognition
Computer vision technology has revolutionized how farmers monitor crop health, detect pests and diseases, and assess field conditions. Using cameras mounted on drones, tractors, or even smartphones, computer vision systems can capture images of crops and analyze them for signs of stress, nutrient deficiencies, pest infestations, or disease outbreaks. Advanced image recognition algorithms can identify specific plant diseases with accuracy rivaling that of experienced agronomists, often detecting problems before they become visible to the human eye. This early detection capability allows for targeted interventions that minimize crop damage while reducing the need for broad-spectrum pesticide applications. According to research published in Plant Pathology Journal, AI-powered disease detection systems can identify common crop diseases with over 98% accuracy, significantly outperforming manual inspection methods.
Drone-based imaging has become particularly valuable for monitoring large agricultural operations, providing high-resolution views of entire fields in a fraction of the time required for ground-based scouting. These aerial systems can capture multispectral and hyperspectral images that reveal information invisible to the human eye, such as variations in chlorophyll content, water stress, or nitrogen levels across a field. Specialized vegetation indices derived from these images, like the Normalized Difference Vegetation Index (NDVI), provide quantitative measures of crop health and vigor. The temporal resolution offered by regular drone flights allows farmers to track changes over time, creating "time-lapse" views of crop development throughout the growing season. This comprehensive monitoring enables more precise management decisions and helps identify problem areas requiring immediate attention before they impact overall yield.
The integration of computer vision with other AI technologies has created powerful new tools for automated farming operations. Vision-guided robotic systems can perform precise tasks like selective harvesting, detecting and picking only fruits that meet specific ripeness criteria while leaving others to mature. Automated weed detection systems combine computer vision with precision sprayers to target herbicide applications only where weeds are present, potentially reducing chemical use by up to 90% compared to conventional broadcast spraying. Similarly, intelligent thinning robots can identify and remove excess seedlings to maintain optimal plant spacing, a task traditionally requiring extensive manual labor. As these technologies mature and become more affordable, they promise to address critical challenges in agriculture, including labor shortages, input costs, and environmental impacts, while improving overall productivity and sustainability.
IoT and Sensor Networks
The Internet of Things (IoT) has created unprecedented opportunities for data collection and real-time monitoring in agricultural settings. Networks of connected sensors can measure soil moisture, temperature, humidity, light levels, CO2 concentration, and numerous other parameters critical to crop growth. These sensors transmit data wirelessly to central management systems where AI algorithms analyze the information and generate recommendations or automatically adjust farm equipment settings. Modern soil moisture sensors, for instance, can be deployed at multiple depths throughout a field to provide detailed information about water availability in the root zone, enabling precision irrigation systems to deliver exactly the right amount of water to each area. According to a study by the University of California, such precision irrigation systems have demonstrated water savings of 20-40% while maintaining or improving crop yields compared to conventional methods.
Weather stations equipped with IoT connectivity offer another valuable data source for AI-driven agriculture. These systems monitor local conditions with greater precision than regional forecasts, accounting for microclimates that can significantly impact growing conditions. When integrated with predictive analytics platforms, farm-specific weather data helps optimize planting schedules, anticipate frost or heat stress events, and plan field operations around favorable conditions. Some advanced weather monitoring networks can even predict localized disease pressure based on temperature and humidity patterns, alerting farmers when environmental conditions favor the development of specific crop diseases. This early warning capability allows for preventative treatments that can be more effective and require lower chemical inputs than reactive approaches after symptoms appear.
The true power of agricultural IoT emerges when multiple sensor systems work together as an integrated network, creating a comprehensive digital representation of farm conditions. Soil sensors, weather stations, equipment monitors, and irrigation controllers can all feed data into unified management platforms that provide farmers with holistic views of their operations. These systems enable sophisticated decision support tools that consider complex interactions between variables—for example, adjusting irrigation schedules based not only on current soil moisture readings but also on weather forecasts, crop growth stage, and soil type variations across fields. As sensor technology becomes more affordable and power-efficient, deployment density continues to increase, providing ever more granular data for AI analysis. The latest developments include biodegradable sensors that can be distributed throughout fields without creating waste and self-powered devices that harvest energy from their environment, eliminating battery replacement needs and enabling truly maintenance-free monitoring networks.
Sustainable Farming Applications
Precision Resource Management
At the heart of AI-driven sustainable agriculture lies precision resource management—the practice of applying water, fertilizers, pesticides, and other inputs at the right time, in the right place, and in the right amounts. Traditional farming often relies on uniform application rates across entire fields, leading to overuse in some areas and insufficient application in others. AI-powered precision farming addresses this inefficiency by creating detailed prescription maps based on soil tests, crop health indicators, yield history, and other variables. These maps guide variable-rate application equipment to adjust input rates continuously as they move across the field, ensuring each area receives optimal treatment. Studies from the University of Nebraska's precision agriculture program have shown that variable-rate fertilizer application can reduce nitrogen use by 15-30% while maintaining or improving yields, significantly reducing both input costs and environmental impacts.
Water management represents another critical area where AI delivers substantial sustainability benefits. Smart irrigation systems use soil moisture sensors, weather data, crop water requirement models, and machine learning algorithms to determine precisely when and how much to irrigate. These systems can account for spatial variability within fields, adjusting water application rates based on soil type, topography, and crop needs at specific locations. Some advanced platforms even incorporate evapotranspiration data and crop growth models to predict water requirements days in advance, optimizing irrigation scheduling around rainfall forecasts and electricity pricing. In regions facing water scarcity, such precision irrigation approaches have become essential for maintaining agricultural productivity while conserving limited water resources. According to field trials conducted across multiple crops and regions, AI-optimized irrigation can reduce water use by 30-50% compared to conventional scheduling methods, while often improving crop quality through more consistent soil moisture levels.
Pesticide and herbicide applications represent both significant costs for farmers and potential environmental concerns. AI-driven approaches to pest and weed management focus on early detection, precise targeting, and minimal chemical use. Instead of calendar-based spraying schedules covering entire fields, smart spraying systems use computer vision to identify specific problem areas requiring treatment. Robotic spot-sprayers can target individual weeds while leaving crops untouched, reducing herbicide use by up to 90% in some applications. For insect pests, AI systems analyze trap data, weather conditions, and historical patterns to predict outbreaks and recommend preemptive measures or optimal treatment timing. Biological control methods can be integrated into these systems, with AI helping to determine when and where to release beneficial organisms based on pest population dynamics. These approaches not only reduce chemical inputs but also help prevent resistance development by using targeted interventions rather than broad-spectrum applications, preserving the long-term efficacy of important crop protection tools.
Soil Health Management
Soil health forms the foundation of sustainable agriculture, and AI technologies offer powerful new approaches to monitoring and improving this vital resource. Advanced soil sensors can now measure not only basic parameters like moisture, temperature, and pH, but also nutrient levels, organic matter content, microbial activity, and compaction. When deployed across fields and integrated with GPS mapping, these sensors create detailed soil health maps that guide variable-rate applications of amendments like lime, gypsum, or compost precisely where needed. Machine learning algorithms analyze these soil data alongside crop performance metrics to identify which soil parameters most strongly influence yields in specific fields, helping farmers prioritize improvement efforts. Some cutting-edge systems even incorporate soil biology assessments, measuring factors like microbial diversity and enzymatic activity that indicate overall soil ecosystem health but were previously difficult to quantify at the field scale.
Cover cropping and crop rotation represent time-tested practices for building soil health, and AI can optimize these approaches for maximum benefit. Advanced planning tools analyze soil conditions, weather patterns, production goals, and historical performance to recommend ideal cover crop species or mixes for specific fields and seasons. These systems can balance multiple objectives simultaneously—like nitrogen fixation, erosion control, weed suppression, and building organic matter—while accounting for practical constraints such as planting windows and equipment limitations. For main crop rotations, AI models can simulate long-term outcomes of different sequences, predicting effects on soil properties, pest and disease cycles, and economic returns over multiple years. According to research from sustainable agriculture institutes, AI-optimized cover cropping strategies have demonstrated 20-35% greater soil organic matter increases compared to standard approaches, accelerating the transition to more resilient and productive soils.
Carbon sequestration in agricultural soils has gained attention as both an environmental benefit and a potential revenue stream through carbon credit markets. AI systems help quantify and optimize this process by modeling how different management practices affect soil carbon levels over time. Machine learning algorithms analyze data from soil samples, remote sensing, weather records, and management histories to estimate current carbon stocks and predict future sequestration rates under various scenarios. These models guide farmers in selecting practices that maximize carbon storage while maintaining profitability, such as reduced tillage, cover cropping, or agroforestry integration. As carbon markets develop, AI-powered measurement, reporting, and verification (MRV) systems are becoming essential for credible carbon accounting at scale. These platforms combine field sampling with predictive modeling to monitor carbon sequestration more efficiently than traditional methods, reducing verification costs while maintaining scientific rigor. By making soil carbon programs more accessible and trustworthy, these technologies help align environmental goals with economic incentives, accelerating adoption of climate-friendly farming practices.
Biodiversity and Ecosystem Services
Agricultural biodiversity provides critical services including pollination, pest control, soil health, and resilience to environmental stresses. AI technologies are creating new opportunities to monitor, protect, and enhance biodiversity within farming systems. Computer vision systems deployed on drones or tractors can identify and map beneficial insect populations, weed species diversity, and wildlife habitat across agricultural landscapes. These biodiversity assessments help farmers understand how their management practices affect ecosystem health and identify opportunities for improvement. Machine learning algorithms can analyze these biodiversity data alongside productivity metrics to identify synergistic relationships—areas where increased biodiversity correlates with improved crop performance—challenging the conventional wisdom that nature conservation and agricultural production must conflict. According to studies from the International Biodiversity Partnership, farms implementing AI-guided biodiversity management have seen pollinator populations increase by 40-70% and natural pest control services improve by 30-50%, often reducing the need for insecticide applications.
Precision conservation represents an emerging application of AI in agricultural landscapes, targeting environmental protection efforts where they deliver maximum benefits. Sophisticated modeling tools analyze topography, hydrology, soil properties, and habitat connectivity to identify critical areas for conservation interventions within and around farmland. These might include riparian buffer zones to filter runoff, wildlife corridors connecting habitat fragments, or flower strips supporting beneficial insects. By focusing conservation efforts on high-impact locations, these approaches maximize environmental benefits while minimizing the land taken out of production. Some advanced platforms even integrate economic models to identify win-win opportunities—areas where conservation provides valuable ecosystem services like erosion control or water purification that directly benefit farm operations. This precision approach helps reconcile the sometimes competing goals of production and conservation, creating multifunctional landscapes that sustain both agriculture and biodiversity.
Agroforestry systems that integrate trees with crops or livestock represent another promising approach to enhancing agricultural biodiversity, and AI tools are making these complex systems more manageable. Machine learning models can predict interactions between tree species and crops under various climate and soil conditions, helping farmers design optimal agroforestry arrangements for their specific contexts. These models account for factors like shade patterns, root competition, nutrient cycling, and microclimate effects to maximize beneficial interactions while minimizing competition. Monitoring systems combining satellite imagery with ground-based sensors track the development of agroforestry plantings over time, providing early warning of any problems and guiding adaptive management. As these systems mature, AI platforms can optimize harvest schedules and management interventions across the multiple components, balancing short-term crop yields with long-term tree growth and ecosystem development. By making agroforestry more predictable and manageable, these technologies are helping scale up this inherently sustainable approach to agriculture that combines productivity with biodiversity conservation, carbon sequestration, and climate resilience.
Yield Optimization Strategies
Predictive Yield Modeling
Advanced predictive modeling represents one of AI's most valuable contributions to agricultural yield optimization. These systems integrate diverse data streams—including historical yields, soil properties, weather conditions, management practices, and remote sensing—to forecast crop performance with increasing accuracy. Modern yield prediction models employ sophisticated machine learning algorithms that continuously improve as they process more seasons of data, learning the complex interactions between variables that influence crop growth and development. Early-season yield forecasts help farmers make informed decisions about resource allocation, marketing strategies, and risk management months before harvest. As the growing season progresses, these models update their predictions based on actual conditions, providing increasingly precise estimates. According to validation studies by agricultural research institutions, the latest AI yield models can predict final harvest outcomes within 5-10% accuracy as early as mid-season for major row crops, giving farmers unprecedented visibility into likely outcomes.
Scenario analysis capabilities built into advanced yield modeling platforms allow farmers to explore "what-if" questions about different management strategies. These tools simulate how changes in planting dates, seeding rates, fertilizer programs, irrigation schedules, or pest management approaches might affect yields under various weather scenarios. By running these virtual experiments before committing to actual field operations, farmers can identify optimal strategies that maximize yield potential while managing risk. Some platforms even incorporate economic models to estimate profitability under different scenarios, accounting for input costs, expected crop prices, and operational expenses. This integrated approach helps farmers optimize not just for maximum yield but for maximum return on investment—a critical distinction in modern agriculture where the highest-yielding approach isn't always the most profitable due to diminishing returns on some inputs.
Field-level yield variability represents a persistent challenge in agriculture, with productivity often differing significantly across a single field due to variations in soil properties, topography, drainage, and other factors. AI-powered yield analysis tools help farmers understand and address this variability by creating detailed maps showing how yields change across their fields over multiple seasons. Machine learning algorithms analyze these spatial patterns alongside other field data to identify the root causes of low-yielding areas—whether soil compaction, pH imbalances, drainage issues, or other factors. Once these underlying causes are understood, targeted interventions can address specific limitations in each field zone. According to case studies from precision agriculture services, this systematic approach to yield gap analysis typically identifies 15-30% yield improvement opportunities even in well-managed operations, often with minimal additional input costs once underlying limitations are addressed.
Crop and Variety Selection
Selecting the optimal crops and varieties for specific growing conditions represents a fundamental decision with enormous impact on agricultural productivity and sustainability. AI-powered crop selection tools analyze detailed soil data, climate records, water availability, market projections, and farm resource constraints to recommend the most suitable crops for each field and season. These systems can account for complex rotational requirements, considering how different crop sequences affect soil health, pest pressure, and long-term productivity. For specialty crop growers facing hundreds of potential options, machine learning algorithms can narrow down choices based on compatibility with local growing conditions, market potential, and farm capabilities, helping farmers identify promising opportunities they might otherwise overlook. According to agricultural extension research, farms using AI-guided crop selection strategies report 12-25% higher net returns compared to traditional approaches based primarily on historical patterns and local convention.
Variety selection within a chosen crop represents another critical decision where AI delivers substantial value. Modern breeding programs release numerous new varieties annually, each with different yield potential, disease resistance profiles, maturity characteristics, and quality attributes. AI-powered variety selection tools analyze performance data from official trials, on-farm testing networks, and seed company research to predict how different varieties will perform under specific growing conditions. Advanced platforms incorporate genetic information alongside phenotypic data, using genomic prediction methods to estimate performance even for newer varieties with limited field testing. These systems can recommend not just individual varieties but complementary portfolios that spread risk across different maturity groups and genetic backgrounds. By matching genetics to environment with unprecedented precision, AI variety selection tools have demonstrated yield advantages of 7-15% over conventional selection methods relying on regional recommendations and limited local experience.
Climate adaptation represents an increasingly important factor in crop and variety selection as weather patterns become more variable and extreme events more frequent. AI models examining how different crops and varieties respond to specific stresses—like heat, drought, excessive rainfall, or frost—help farmers select genetics with resilience to the most relevant threats in their region. These systems analyze historical weather data to identify changing patterns and emerging risks, then match crop genetics to these evolving conditions. Some advanced platforms simulate crop growth under projected future climate scenarios, helping farmers prepare for longer-term changes by gradually shifting their production systems. This forward-looking approach is especially valuable for perennial crops like orchards and vineyards, where today's planting decisions determine production potential for decades to come. As climate uncertainty increases, the predictive power of AI provides a valuable tool for maintaining productivity through thoughtful adaptation rather than reactive crisis management.
Harvest Optimization
Determining the optimal harvest timing balances multiple factors: crop maturity, weather conditions, equipment availability, and downstream logistics. AI harvest optimization systems analyze crop maturity indicators from field sensors, satellite imagery, and ground sampling alongside weather forecasts and operational constraints to recommend ideal harvest windows for each field. For crops where quality parameters significantly impact value—like protein content in wheat or sugar levels in fruits—these systems can prioritize harvest sequences to maximize quality-based premiums. In regions where weather risks like rainfall or frost threaten harvest operations, AI models incorporate probability forecasts to balance the risks of waiting for ideal maturity against the potential for weather-related losses. According to case studies from major grain-producing regions, farms using AI-optimized harvest scheduling report 5-8% higher crop value through quality improvements and weather loss reduction, with particularly significant benefits in challenging harvest seasons.
Harvest logistics coordination represents another area where AI delivers substantial value, especially for large operations managing multiple fields, crops, and equipment sets simultaneously. Advanced planning systems optimize the movement of harvesters, transport vehicles, and support equipment across farms, minimizing idle time and maximizing daily throughput. These platforms account for field readiness, travel distances, equipment capacities, and processing facility scheduling to create integrated harvest plans that coordinate all elements of the operation. Real-time adjustments automatically recalculate plans when equipment breakdowns, weather delays, or other disruptions occur, maintaining overall efficiency despite inevitable complications. For operations delivering to processing facilities with limited receiving capacity, AI systems can coordinate harvest timing and delivery scheduling to avoid bottlenecks and rejection risks. By streamlining these complex logistics, harvest optimization platforms typically reduce equipment operating hours by 15-25% while harvesting the same acreage, significantly decreasing fuel consumption, labor costs, and equipment wear.
Post-harvest handling decisions significantly impact both crop marketability and storage stability, and AI systems help optimize these critical processes. Sensor systems measuring moisture, temperature, and quality parameters during harvest feed into decision support tools that direct crops to appropriate storage facilities based on condition and intended marketing windows. For crops requiring drying, AI platforms optimize this energy-intensive process by balancing drying costs against quality preservation and market timing. Machine learning algorithms analyzing historical storage data help predict how different crop conditions will affect storage stability, identifying high-risk lots requiring priority marketing or specialized storage conditions. For operations with multiple marketing options—like direct sales, contract fulfillment, or commodity markets—AI systems recommend optimal allocation strategies based on current quality measurements, market conditions, and forward price projections. This integrated approach to post-harvest management helps maximize the value realized from each crop while minimizing losses during storage and handling, extending the benefits of precision agriculture beyond the growing season through the entire value chain.
Economic and Implementation Considerations
ROI Analysis and Cost Effectiveness
Implementing AI technologies in agriculture requires significant investment, making thorough return-on-investment analysis essential for farmers considering these systems. The economics of AI adoption vary considerably depending on farm size, crop value, existing technology infrastructure, and specific applications being considered. High-value specialty crops typically offer faster returns on AI investments due to the substantial revenue impact of even modest yield or quality improvements. For commodity crops with tighter margins, careful cost-benefit analysis must consider both direct benefits like input savings and yield increases alongside indirect advantages such as labor efficiency, risk reduction, and sustainability improvements. According to comprehensive studies from agricultural economics departments, typical payback periods for major AI system investments range from 1-2 years for large specialty crop operations to 3-5 years for mid-sized commodity producers, though specific results vary widely based on implementation quality and how effectively insights are converted into management actions.
The economics of AI adoption in agriculture are evolving rapidly as technology costs decrease while capabilities expand. Early precision agriculture systems often required substantial upfront capital investment in proprietary hardware, software, and services. Today's market increasingly offers scalable solutions with lower entry barriers, including subscription-based software platforms, retrofit kits for existing equipment, and service models where providers handle data collection and analysis for a fee. These flexible approaches allow farmers to start with targeted applications addressing their most pressing challenges, then expand as they build confidence and verify returns. Cloud computing and smartphone integration have dramatically reduced hardware requirements for many applications, making advanced analytics accessible even to smaller operations. According to market research from AgTech Investment Analysis, average implementation costs for comprehensive farm management platforms have declined 45-60% over the past five years while processing capabilities have increased exponentially, significantly improving the cost-benefit equation for agricultural AI adoption.
Beyond direct financial returns, comprehensive economic analysis must consider risk management benefits and sustainability advantages that may not appear in simple cash flow calculations. AI systems often deliver their greatest value by reducing variability and preventing losses rather than just increasing average returns—helping farms maintain profitability through challenging seasons when unprepared operations suffer significant losses. Early disease detection systems, for example, may show modest benefits in typical years but prevent catastrophic crop failures during high-pressure seasons. Similarly, precision irrigation delivers dramatic advantages during drought years compared to normal rainfall patterns. Long-term soil health improvements from AI-optimized management may not show immediate yield impacts but build production resilience and reduce input requirements over multiple seasons. Some sustainability benefits may eventually translate into financial advantages through carbon credit programs, certification premiums, or reduced regulatory compliance costs. Forward-thinking economic analysis accounts for these diverse benefits across multiple time horizons, recognizing that the full value of agricultural AI extends beyond immediate productivity gains to include risk reduction, resource efficiency, and long-term sustainability that positions operations for success in an increasingly challenging production environment.
Conclusion
Artificial intelligence is transforming agriculture from an industry often characterized by tradition and intuition into one driven increasingly by data and analytics. The technologies and approaches described in this article represent not just incremental improvements to existing systems but a fundamental reimagining of how we produce food, manage natural resources, and maintain agricultural landscapes. By enabling more precise, targeted interventions—applying inputs exactly when and where they're needed—AI helps reconcile the sometimes competing goals of productivity and sustainability. The environmental benefits are substantial: reduced chemical usage, more efficient water consumption, decreased soil erosion, and enhanced biodiversity. Equally important are the economic advantages: higher yields, improved quality, reduced input costs, and greater resilience to climate variability and market fluctuations.
As these technologies continue to mature and become more accessible, their adoption will likely accelerate across farm sizes and production systems. The barriers that once limited precision agriculture to the largest, most technologically advanced operations are steadily falling as costs decrease, user interfaces become more intuitive, and service models evolve to accommodate diverse needs. This democratization of agricultural AI promises to extend its benefits to a broader range of farmers worldwide. However, realizing the full potential of these technologies requires not just technical development but supportive policy environments, educational initiatives, infrastructure improvements, and business models that ensure equitable access. The most successful agricultural systems of the future will likely be those that effectively integrate AI capabilities with human expertise, combining the data-processing power of algorithms with the contextual understanding and adaptive decision-making of experienced farmers.
Perhaps most importantly, AI-driven agriculture offers a pathway to address the central challenge facing global food systems: producing enough nutritious food for a growing population while reducing environmental impacts and adapting to climate change. By enabling more efficient resource use and more resilient production systems, these technologies can help secure food supplies while preserving the natural capital upon which agriculture ultimately depends. As we navigate the complex challenges of 21st-century food production, the intelligent application of AI will be not just an advantage but a necessity for sustainable agricultural systems capable of feeding humanity while maintaining the health of our planet.
FAQ Section
What is AI-driven agriculture? AI-driven agriculture refers to the application of artificial intelligence technologies like machine learning, computer vision, and predictive analytics to optimize farming operations, increase yields, and improve sustainability. These systems analyze data from various sources to provide insights and automate decisions that were previously based primarily on human experience and intuition.
How does AI help in sustainable farming? AI enables sustainable farming by optimizing resource use (reducing water, fertilizer, and pesticide usage), improving soil health monitoring, enhancing biodiversity management, and reducing environmental impacts while maintaining or improving productivity. By targeting inputs precisely where and when they're needed, AI helps minimize waste and environmental contamination.
What are the main AI technologies used in agriculture? The main AI technologies in agriculture include machine learning for predictive analytics, computer vision for crop monitoring and disease detection, IoT sensor networks for real-time data collection, and robotics for automation of farming tasks. These technologies work together in integrated systems that collect, analyze, and act upon agricultural data.
How much can AI technologies reduce water usage in farming? AI-powered precision irrigation systems can reduce water usage by 30-50% compared to conventional methods by delivering water exactly when and where crops need it based on soil moisture sensors, weather data, and crop water requirement models. These savings are particularly significant in water-scarce regions facing increasing drought pressure.
What yield improvements can farmers expect from implementing AI? Yield improvements from AI implementation typically range from 7-20% depending on the specific technologies deployed, crop types, and existing management practices, with the greatest gains often seen in operations transitioning from conventional to precision methods. Yield stability across varying weather conditions also typically improves.
What is the typical ROI timeline for agricultural AI investments? ROI timelines for agricultural AI investments generally range from 1-2 years for high-value specialty crops to 3-5 years for commodity crops, with precision irrigation and variable rate fertilization often delivering the fastest returns. As technology costs continue to decrease, these payback periods are becoming shorter.
How can small farms benefit from AI agricultural technologies? Small farms can benefit from AI through scalable solutions like smartphone-based apps for disease identification, subscription services for satellite imagery analysis, equipment-sharing programs for advanced machinery, and cooperative data-sharing initiatives that pool resources for greater insights. Many AI providers now offer entry-level solutions designed specifically for smaller operations.
What data is needed to implement AI in farming operations? Implementing AI in farming typically requires soil data (composition, nutrients, moisture), crop information (variety, growth stage, health), weather data (current and historical), equipment performance logs, and historical yield records to establish baselines and train predictive models. The value of AI systems generally increases with data quality and quantity.
How does AI help with carbon sequestration in agriculture? AI helps with carbon sequestration by modeling how different practices affect soil carbon levels, optimizing cover crop selection and management, monitoring changes in soil organic matter, and providing measurement and verification systems necessary for carbon credit programs. These capabilities help farmers participate in emerging carbon markets while improving soil health.
What challenges exist in adopting AI for agriculture? Challenges in adopting agricultural AI include initial investment costs, technical knowledge requirements, data ownership and privacy concerns, connectivity issues in rural areas, integration with existing equipment, and ensuring the accuracy and reliability of AI recommendations. Addressing these barriers requires both technological solutions and supportive policy frameworks.
Additional Resources
Agricultural Data Coalition - A collaborative initiative providing guidelines for agricultural data ownership, privacy, and security, along with best practices for implementing data-driven farming systems.
National Precision Agriculture Demonstration Network - A network of demonstration farms showcasing different AI agricultural technologies in real-world settings, with case studies, economic analyses, and implementation guides.
Sustainable Smart Farming Initiative - Research and resources on integrating AI technologies with sustainable farming principles, including carbon sequestration, biodiversity enhancement, and resource conservation.
AgTech Investment Analysis Report 2023 - A comprehensive annual report analyzing investment trends, technology adoption rates, and economic impacts of AI and other technologies in global agriculture.
AI in Agriculture: Technical Implementation Guide - A practical guide for farmers and agricultural professionals covering hardware requirements, software platforms, data management, and integration strategies for AI agricultural systems.