Add your promotional text...
AI Media Monitoring: Advanced Crisis Management Techniques
Discover advanced AI media monitoring techniques for crisis management. Learn how artificial intelligence transforms real-time threat detection, sentiment analysis, and automated response systems for modern businesses.
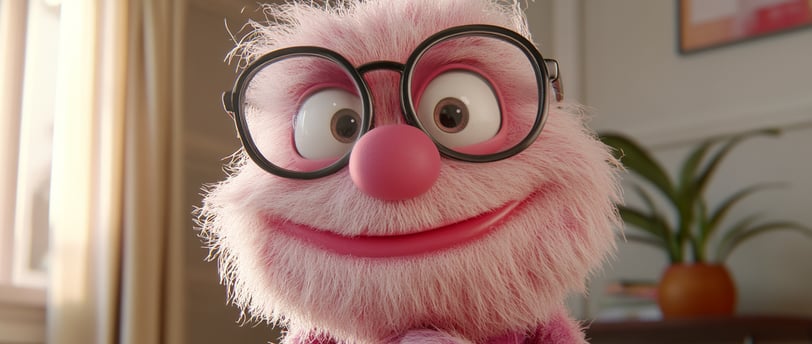
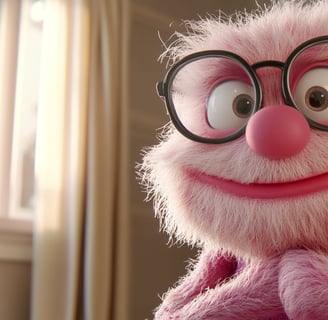
A single social media post can ignite a crisis that spreads across the globe in minutes. Traditional media monitoring methods simply cannot keep pace with the velocity and volume of modern information flow. This is where artificial intelligence steps in as a game-changer, offering sophisticated capabilities that transform how organizations detect, assess, and respond to potential crises.
The integration of AI into media monitoring represents a fundamental shift from reactive to proactive crisis management. While human analysts might catch major stories hours after they break, AI systems can identify emerging threats in real-time, analyze sentiment patterns across millions of data points, and even predict crisis trajectories before they fully materialize. This technological evolution isn't just about speedโit's about precision, scalability, and the ability to navigate the complex nuances of human communication across diverse digital platforms.
Throughout this comprehensive guide, we'll explore the cutting-edge techniques that are reshaping crisis management. From natural language processing algorithms that understand context and emotion to machine learning models that can predict viral content patterns, we'll delve into the practical applications that are helping organizations stay ahead of potential reputation disasters. We'll also examine real-world case studies, discuss implementation strategies, and provide actionable insights for businesses looking to harness the power of AI in their crisis management frameworks.
Understanding AI-Powered Media Monitoring
The Evolution from Traditional to Intelligent Monitoring
Traditional media monitoring relied heavily on keyword-based alerts and human analysis, creating significant blind spots in crisis detection. These systems often generated false positives, missed contextual nuances, and struggled with the sheer volume of content across multiple platforms. The limitations became particularly evident during high-stakes situations where timing and accuracy were critical.
Modern AI-powered monitoring systems represent a quantum leap forward in capability and sophistication. These systems employ advanced natural language processing (NLP) to understand context, sentiment, and intent behind communications. Rather than simply flagging mentions of specific terms, AI systems can distinguish between neutral product discussions and emerging complaints that might escalate into crisis situations. They analyze linguistic patterns, emotional undertones, and even visual content to provide comprehensive threat assessment.
The transformation extends beyond detection to include predictive analytics capabilities. Machine learning algorithms trained on historical crisis data can identify patterns that precede major reputation events. These systems learn from past incidents, continuously refining their ability to recognize early warning signals that human analysts might overlook. This predictive capability allows organizations to implement preventive measures before issues reach critical mass.
Furthermore, AI monitoring systems excel at cross-platform correlation, connecting dots between seemingly unrelated conversations across different social networks, news outlets, and digital forums. This holistic approach provides crisis managers with a complete picture of emerging threats, rather than fragmented insights from individual platforms.
Core Technologies Driving Advanced Monitoring
Natural Language Processing forms the backbone of intelligent media monitoring, enabling systems to understand human communication in all its complexity. Advanced NLP models can parse multiple languages, interpret slang and colloquialisms, recognize sarcasm and irony, and even understand cultural references that might be lost on traditional keyword-based systems. These capabilities are essential for accurate threat assessment in diverse, global communication environments.
Sentiment analysis technology has evolved far beyond simple positive, negative, or neutral classifications. Modern AI systems can detect subtle emotional nuances, intensity levels, and even predict sentiment trajectory based on conversation patterns. They can distinguish between constructive criticism and potentially damaging attacks, helping crisis managers prioritize their responses more effectively.
Computer vision and image recognition capabilities are increasingly important as visual content dominates social media platforms. AI systems can analyze images and videos for brand mentions, inappropriate content associations, or visual elements that might contribute to reputation risks. This includes detecting deepfakes, manipulated images, or unauthorized use of branded content that could impact organizational reputation.
Machine learning algorithms continuously improve monitoring accuracy through pattern recognition and anomaly detection. These systems learn from feedback, adjusting their sensitivity and specificity based on organizational preferences and industry-specific risk factors. Over time, they become increasingly adept at distinguishing between genuine threats and background noise, reducing false alarms while maintaining comprehensive coverage.
Real-Time Threat Detection and Assessment
Speed and Accuracy in Crisis Identification
The velocity of modern information spread demands monitoring systems that can identify potential crises within minutes of their emergence. AI-powered systems excel in this environment by processing vast amounts of data simultaneously across multiple channels. Unlike human analysts who might review content sequentially, AI systems can monitor thousands of sources concurrently, providing near-instantaneous threat detection.
Advanced algorithms employ sophisticated scoring mechanisms that weigh multiple factors when assessing potential threats. These factors include message velocity (how quickly content is spreading), source credibility (the influence and reach of content creators), engagement patterns (likes, shares, comments), and contextual relevance (how directly content relates to organizational interests). This multi-dimensional analysis provides more accurate threat assessment than simple volume-based alerts.
The integration of real-time data feeds from news wire services, social media APIs, and web crawling technologies ensures comprehensive coverage across all relevant digital channels. AI systems can correlate information from these diverse sources to identify coordinated campaigns, astroturfing attempts, or organic viral content that might impact organizational reputation. This cross-source analysis provides crucial context for crisis assessment and response planning.
Automated escalation protocols built into AI monitoring systems ensure that high-priority threats receive immediate attention from human crisis managers. These systems can distinguish between routine mentions that require minimal intervention and critical situations that demand immediate executive attention. Smart routing capabilities ensure that the right information reaches the right people at the right time, optimizing response efficiency.
Pattern Recognition and Anomaly Detection
Machine learning algorithms excel at identifying subtle patterns that might escape human observation, particularly in high-volume monitoring environments. These systems analyze historical data to establish baseline patterns for normal conversation volumes, sentiment distributions, and engagement rates. When current activity deviates significantly from established norms, the system flags these anomalies for further investigation.
Behavioral pattern analysis extends beyond simple volume metrics to include sophisticated understanding of how crises typically develop and spread. AI systems can recognize the characteristic signatures of different crisis types, from product safety issues to executive misconduct scandals. This pattern recognition enables more accurate prediction of crisis trajectory and potential impact, informing response strategy development.
Geographic and temporal pattern analysis provides additional layers of insight for crisis assessment. AI systems can identify whether negative sentiment is concentrated in specific regions, time zones, or demographic segments. This granular understanding helps organizations tailor their response strategies to address specific audience concerns and prevent crisis expansion into new markets or communities.
Network analysis capabilities allow AI systems to map the relationships between content creators, influencers, and audience segments involved in emerging crises. By understanding how information flows through these networks, organizations can identify key nodes for targeted intervention and predict how crises might spread through different communities or stakeholder groups.
Sentiment Analysis and Contextual Understanding
Beyond Basic Sentiment Classification
Modern AI sentiment analysis has evolved far beyond the simplistic positive-negative-neutral classifications of early systems. Contemporary algorithms can detect complex emotional states including frustration, disappointment, anger, excitement, and concern, providing crisis managers with nuanced understanding of stakeholder feelings. This emotional granularity is crucial for crafting appropriate response strategies that acknowledge and address specific stakeholder concerns.
Intensity measurement capabilities allow AI systems to gauge not just what people are feeling, but how strongly they feel it. A mildly disappointed customer comment requires different handling than an outraged complaint from an influential reviewer. AI systems can calibrate these intensity levels, helping crisis managers prioritize their responses and allocate resources more effectively during high-pressure situations.
Contextual sentiment analysis represents a significant advancement in AI capabilities, enabling systems to understand how sentiment relates to specific aspects of organizational operations. Rather than providing overall sentiment scores, advanced systems can break down reactions by product lines, service areas, executive leadership, or corporate initiatives. This granular analysis helps organizations understand precisely which elements of their operations are driving positive or negative reactions.
Temporal sentiment tracking reveals how public opinion evolves over time, providing insights into crisis momentum and response effectiveness. AI systems can identify whether sentiment is improving, deteriorating, or remaining stable, allowing crisis managers to adjust their strategies based on real-time feedback. This capability is particularly valuable for measuring the impact of crisis response communications and identifying when additional intervention might be necessary.
Cultural and Linguistic Nuances
Global organizations face the challenge of monitoring sentiment across diverse cultural and linguistic contexts where the same words might carry different connotations. Advanced AI systems incorporate cultural sensitivity algorithms that understand how expressions of dissatisfaction, humor, or concern vary across different regions and communities. This cultural awareness prevents misinterpretation of regional communication styles as crisis indicators.
Multilingual sentiment analysis capabilities ensure comprehensive monitoring in organizations' global markets. These systems don't simply translate content into a single language for analysis; instead, they perform native-language sentiment analysis to preserve cultural and linguistic nuances that might be lost in translation. This approach provides more accurate assessment of international reputation risks and opportunities.
Slang, colloquialisms, and evolving language patterns pose ongoing challenges for sentiment analysis systems. AI algorithms continuously learn from new linguistic developments, updating their understanding of contemporary communication styles. This adaptive capability is essential for accurate sentiment assessment among younger demographics and emerging online communities where language evolves rapidly.
Industry-specific terminology and jargon require specialized training for AI sentiment analysis systems. Healthcare, technology, financial services, and other specialized sectors use unique vocabularies that general-purpose AI systems might misinterpret. Customized training on industry-specific language patterns ensures accurate sentiment assessment within specialized professional communities and regulatory environments.
Automated Response Systems and Workflows
Intelligent Crisis Response Automation
The speed of modern crisis development often outpaces traditional response capabilities, making automated response systems essential for effective crisis management. AI-powered automation can execute predetermined response protocols within minutes of crisis detection, ensuring that critical stakeholders receive timely information and that containment efforts begin immediately. These systems don't replace human judgment but rather provide the rapid initial response that buys time for more thoughtful strategic decision-making.
Automated stakeholder notification systems ensure that relevant internal teams, executives, and external partners receive immediate alerts when potential crises are detected. These systems can customize notification content based on recipient roles, providing executives with high-level summaries while sending detailed technical information to operational teams. Smart routing capabilities ensure that notifications reach the right people based on crisis type, severity level, and organizational hierarchy.
Social media response automation represents one of the most visible applications of AI in crisis management. Sophisticated systems can automatically post holding statements, direct concerned customers to appropriate support channels, or implement content moderation protocols to prevent crisis escalation. These automated responses are carefully crafted to acknowledge concerns while avoiding commitments that might complicate resolution efforts.
Workflow orchestration capabilities coordinate complex response activities across multiple departments and stakeholders. AI systems can automatically create task lists, schedule emergency meetings, prepare briefing documents, and even initiate legal review processes based on crisis type and severity. This orchestration ensures that response efforts proceed efficiently without missing critical steps or stakeholder communications.
Dynamic Response Escalation
Intelligent escalation protocols adapt response intensity based on crisis severity and development patterns. Rather than relying on static escalation triggers, AI systems continuously assess crisis momentum and adjust response protocols accordingly. This dynamic approach ensures appropriate resource allocation while preventing overreaction to minor issues or underestimation of serious threats.
Multi-tier response frameworks enable graduated crisis response that matches intervention intensity to threat severity. AI systems can distinguish between situations requiring simple customer service responses and those demanding C-level involvement or external communications. This graduated approach optimizes resource utilization while ensuring appropriate executive attention for serious reputation threats.
Predictive escalation capabilities analyze crisis development patterns to anticipate when additional intervention might be necessary. By understanding how similar crises have evolved in the past, AI systems can recommend proactive escalation measures before situations deteriorate beyond easy resolution. This forward-looking approach helps organizations stay ahead of crisis curves rather than constantly reacting to developments.
Cross-functional coordination mechanisms ensure that escalated responses involve appropriate stakeholders from legal, communications, operations, and executive teams. AI systems can automatically brief new stakeholders on crisis background, current status, and pending action items, enabling rapid integration into response efforts without time-consuming status meetings.
Integration with Existing Crisis Management Frameworks
Seamless Platform Integration
Successful AI media monitoring implementation requires seamless integration with existing organizational systems and workflows. Modern AI platforms are designed with robust API capabilities that allow integration with customer relationship management systems, help desk platforms, communication tools, and business intelligence dashboards. This integration ensures that crisis-related insights flow naturally into existing operational processes without creating information silos or workflow disruptions.
Data synchronization capabilities ensure that AI monitoring insights are automatically shared with relevant business systems, creating comprehensive views of customer sentiment, operational impact, and response effectiveness. Integration with CRM systems allows customer service teams to access real-time sentiment data when handling support requests, while connection to business intelligence platforms enables executives to understand crisis impact on key performance indicators.
Single sign-on (SSO) integration simplifies user access management while maintaining security protocols. Crisis management teams can access AI monitoring platforms using existing organizational credentials, reducing administrative overhead and ensuring compliance with security policies. Role-based access controls ensure that sensitive crisis information reaches only authorized personnel while enabling broad situational awareness across relevant teams.
Mobile integration capabilities ensure that crisis managers can access critical information and response tools regardless of location. Modern AI monitoring platforms provide full-featured mobile applications that enable real-time monitoring, alert management, and response coordination from smartphones and tablets. This mobility is essential for effective crisis management in today's always-connected business environment.
Workflow Optimization and Enhancement
AI media monitoring enhances rather than replaces existing crisis management workflows, augmenting human capabilities with intelligent automation and analysis. The technology excels at handling high-volume, repetitive monitoring tasks while flagging situations that require human expertise and judgment. This division of labor optimizes both efficiency and effectiveness in crisis management operations.
Process standardization benefits significantly from AI integration, as systems can enforce consistent response protocols while adapting to specific crisis characteristics. AI platforms can maintain detailed logs of response activities, ensuring compliance with regulatory requirements and providing valuable data for post-crisis analysis and improvement. This documentation capability supports continuous improvement in crisis management capabilities.
Decision support functionality provides crisis managers with AI-generated insights and recommendations without constraining human judgment. Systems can analyze historical response effectiveness, predict likely outcomes of different intervention strategies, and highlight potential risks or opportunities that human analysts might overlook. This augmented decision-making approach combines AI analytical power with human creativity and intuition.
Performance measurement and optimization capabilities enable continuous improvement in crisis management effectiveness. AI systems can track response times, measure sentiment impact of different communication strategies, and identify patterns in successful crisis resolution. These insights inform training programs, process refinements, and strategic planning for enhanced crisis preparedness.
Machine Learning Models for Predictive Crisis Analytics
Advanced Predictive Modeling Techniques
Machine learning models trained on historical crisis data can identify early warning patterns that precede major reputation events, enabling proactive intervention before situations escalate beyond easy resolution. These predictive models analyze complex combinations of factors including sentiment velocity, source credibility patterns, content characteristics, and external environmental factors to assess crisis probability and potential impact.
Deep learning neural networks excel at recognizing subtle patterns in large datasets that might escape traditional statistical analysis. These models can process vast amounts of historical crisis data to identify non-obvious correlations between seemingly unrelated factors and crisis outcomes. For example, they might discover that certain combinations of industry news, competitor activities, and social media sentiment patterns reliably precede specific types of reputation challenges.
Ensemble modeling approaches combine multiple machine learning algorithms to improve prediction accuracy and reliability. By aggregating insights from different algorithmic approaches, these systems provide more robust predictions while reducing the risk of model-specific biases or blind spots. This multi-model approach is particularly valuable in crisis prediction where accuracy is paramount and false positives or negatives can have significant consequences.
Time series analysis capabilities enable AI systems to understand how crisis risk factors evolve over time, identifying trends and patterns that inform predictive models. These temporal insights help organizations understand whether their reputation risks are increasing, decreasing, or remaining stable, enabling strategic adjustments to communication, marketing, or operational strategies.
Risk Scoring and Probability Assessment
Sophisticated risk scoring algorithms weigh multiple factors to provide comprehensive threat assessment that goes beyond simple sentiment metrics. These systems consider source authority, content virality potential, historical precedent, competitive landscape factors, and external environmental conditions to generate nuanced risk scores that inform strategic decision-making.
Probabilistic modeling provides crisis managers with confidence intervals and uncertainty estimates alongside risk predictions, enabling more informed decision-making under uncertainty. Rather than providing false precision, these systems acknowledge the inherent uncertainty in crisis prediction while still providing valuable guidance for resource allocation and preparedness planning.
Scenario modeling capabilities allow organizations to understand how different factors might influence crisis development and impact. AI systems can simulate various scenarios based on different response strategies, external developments, or stakeholder behaviors, helping crisis managers prepare for multiple contingencies and optimize their response strategies.
Dynamic risk assessment ensures that predictions remain current as situations evolve and new information becomes available. Rather than providing static predictions, AI systems continuously update risk assessments based on real-time data feeds, ensuring that crisis managers always have access to the most current threat intelligence for decision-making.
Case Studies and Real-World Applications
Fortune 500 Crisis Prevention Success
A major technology company implemented advanced AI media monitoring to address recurring product launch reputation challenges. Their previous manual monitoring approach consistently failed to detect early negative sentiment patterns, resulting in several product launch crises that required extensive damage control efforts. The AI system implementation transformed their capability to identify potential issues days or weeks before they reached crisis levels.
The AI monitoring system detected unusual patterns in developer community discussions about an upcoming software release, identifying specific technical concerns that were gaining momentum among influential users. Unlike previous keyword-based systems that missed these technical discussions, the AI system understood the contextual significance of the concerns and their potential impact on broader user adoption. This early detection enabled the company to address technical issues proactively and adjust their launch communications strategy.
Predictive analytics capabilities identified that the negative sentiment was likely to amplify during the official product launch based on historical patterns from similar technical concerns. Armed with this insight, the company implemented targeted developer outreach programs, technical documentation improvements, and revised launch messaging that acknowledged and addressed the identified concerns. The result was a successful product launch with significantly reduced negative sentiment compared to previous releases.
Post-implementation analysis revealed that the AI system reduced crisis response costs by 60% while improving brand sentiment scores during product launches. The company's ability to proactively address concerns before they escalated into major reputation challenges demonstrated the significant value of predictive crisis management approaches enabled by AI technology.
Retail Brand Reputation Recovery
A global retail brand faced a complex reputation crisis involving supply chain ethical concerns that spread rapidly across multiple social media platforms and traditional news outlets. Their existing crisis management approach struggled to coordinate responses across different markets and stakeholder groups, resulting in inconsistent messaging and prolonged negative coverage.
The AI media monitoring system provided real-time sentiment analysis across 15 different languages and cultural contexts, enabling the company to understand how the crisis was being perceived differently across their global markets. The system identified that certain markets were more concerned about environmental impact while others focused on labor practices, requiring tailored response strategies for different regions.
Automated response coordination ensured consistent messaging across all markets while allowing for cultural and linguistic customization. The AI system generated baseline response templates that were adapted by local teams based on specific cultural contexts and stakeholder concerns. This approach maintained brand consistency while demonstrating cultural sensitivity and local market understanding.
Sentiment tracking throughout the crisis resolution process enabled the company to measure response effectiveness in real-time and adjust strategies based on stakeholder feedback. The AI system identified that certain response elements were more effective in specific markets, informing optimization of ongoing communication strategies. Within three months, brand sentiment scores had recovered to pre-crisis levels, with some markets showing improved scores compared to baseline measurements.
Government Agency Public Communication Enhancement
A federal regulatory agency implemented AI media monitoring to improve their crisis communication effectiveness during high-profile enforcement actions and policy announcements. Their previous approach relied heavily on traditional media monitoring and delayed stakeholder feedback, resulting in communication strategies that often failed to address public concerns effectively.
The AI system's ability to analyze social media sentiment in real-time revealed that public concerns about regulatory actions often focused on different aspects than traditional media coverage suggested. While news outlets emphasized policy implications, social media discussions revealed significant concerns about implementation timelines, compliance costs, and enforcement fairness. This insight enabled the agency to develop more comprehensive communication strategies that addressed actual stakeholder concerns.
Predictive modeling helped the agency anticipate public reaction to policy announcements, enabling proactive communication strategies that addressed likely concerns before they became widespread criticism. The system identified patterns in how different stakeholder groups typically responded to various types of regulatory actions, informing targeted outreach and education efforts.
Implementation of AI-driven communication optimization resulted in 40% improvement in public sentiment scores following major policy announcements. The agency's ability to demonstrate responsiveness to public concerns while maintaining regulatory authority significantly enhanced their reputation and effectiveness in stakeholder engagement.
Industry-Specific Implementation Strategies
Technology Sector Considerations
Technology companies face unique challenges in crisis management due to the technical sophistication of their audiences and the rapid pace of industry developments. AI media monitoring systems for tech companies must be capable of understanding technical terminology, developer community dynamics, and the complex relationships between product features, user experiences, and market expectations. These systems require specialized training on technology vocabulary and industry-specific communication patterns.
The technical nature of many technology products means that crises often begin in specialized communities before spreading to mainstream audiences. AI monitoring systems must track discussions in developer forums, technical support channels, open-source repositories, and professional networking platforms where technical experts gather. Early detection in these specialized channels provides crucial lead time for addressing technical issues before they impact broader customer sentiment.
Integration with product development and engineering teams becomes particularly important in technology companies where crisis resolution often requires technical solutions rather than just communication strategies. AI systems can automatically create technical incident reports, escalate issues to appropriate engineering teams, and track resolution progress through development pipelines. This technical integration ensures that crisis response includes both communication and product improvement elements.
Competitive intelligence capabilities are especially valuable in the fast-moving technology sector where company reputations can be significantly impacted by competitor product launches, industry partnerships, or technical breakthroughs. AI monitoring systems can track competitor activities and assess their potential impact on organizational reputation, enabling proactive positioning and communication strategies.
Healthcare and Pharmaceutical Applications
Healthcare organizations operate in highly regulated environments where crisis management must balance public communication with regulatory compliance and patient privacy requirements. AI media monitoring systems for healthcare must be configured to identify potential compliance issues, patient safety concerns, and regulatory scrutiny while maintaining strict privacy protections and adherence to healthcare communication standards.
Medical misinformation detection represents a critical capability for healthcare AI monitoring systems. These systems must distinguish between legitimate medical concerns and potentially harmful misinformation while avoiding suppression of valid patient experiences or medical professional discussions. Advanced natural language processing capabilities enable these systems to understand medical terminology and assess the credibility of health-related claims.
Patient sentiment analysis requires particular sensitivity given the emotional nature of healthcare experiences and the potential impact of negative publicity on patient care decisions. AI systems must be trained to recognize the difference between individual patient concerns that require customer service intervention and systemic issues that might indicate broader quality or safety problems requiring immediate organizational response.
Regulatory monitoring capabilities help healthcare organizations track regulatory agency communications, policy changes, and enforcement actions that might impact their operations or reputation. AI systems can correlate regulatory developments with organizational activities to identify potential compliance risks and inform proactive communication strategies with regulators and stakeholders.
Financial Services Compliance Integration
Financial services organizations face complex regulatory environments where crisis management must align with strict compliance requirements and regulatory reporting obligations. AI media monitoring systems must be designed to identify potential regulatory violations, market manipulation concerns, or consumer protection issues while maintaining detailed audit trails for regulatory review.
Market sentiment analysis for financial institutions requires sophisticated understanding of economic terminology, financial product characteristics, and regulatory requirements. These systems must distinguish between routine market commentary and potentially damaging allegations or concerns that could impact regulatory standing or customer confidence. Integration with compliance management systems ensures that crisis response efforts align with regulatory obligations.
Fraud and security incident monitoring represents a specialized application of AI media monitoring in financial services. These systems can identify discussions of potential security breaches, fraud attempts, or system vulnerabilities across various online channels, enabling rapid response to protect customer assets and organizational reputation. Real-time detection capabilities are essential given the potential financial impact of security-related reputation damage.
Consumer protection compliance requires AI systems to monitor customer complaints and concerns across multiple channels while ensuring appropriate escalation to compliance teams when regulatory notification requirements might be triggered. These systems must understand the difference between routine customer service issues and situations that require formal regulatory reporting or consumer protection intervention.
Implementation Best Practices and Challenges
Strategic Planning and Deployment
Successful AI media monitoring implementation requires comprehensive strategic planning that aligns technology capabilities with organizational crisis management objectives. Organizations must begin with clear definition of their crisis management goals, stakeholder priorities, and risk tolerance levels to ensure that AI systems are configured to support specific business requirements rather than generic monitoring approaches.
Stakeholder requirement gathering involves extensive consultation with crisis management teams, communications professionals, legal counsel, customer service leadership, and executive stakeholders to understand diverse perspectives on crisis definition, escalation protocols, and response requirements. This comprehensive requirement analysis ensures that AI systems address real organizational needs rather than theoretical monitoring capabilities.
Pilot program implementation provides valuable learning opportunities for organizations to test AI monitoring capabilities in controlled environments before full-scale deployment. These pilot programs should focus on specific use cases or market segments where the organization can evaluate system effectiveness and refine configuration parameters based on real-world performance data.
Change management strategies address the cultural and operational adjustments required for successful AI integration into existing crisis management workflows. Organizations must prepare teams for new responsibilities, modified escalation procedures, and enhanced analytical capabilities while ensuring that human expertise remains central to strategic decision-making processes.
Data Quality and Training Considerations
AI system effectiveness depends heavily on the quality and comprehensiveness of training data used to develop monitoring models. Organizations must invest in curating high-quality historical crisis data, sentiment examples, and industry-specific communication patterns to ensure accurate system performance. Poor training data leads to inaccurate threat detection and excessive false positives that undermine user confidence.
Bias mitigation strategies address potential algorithmic biases that might skew crisis detection or sentiment analysis results. AI systems trained on limited or biased datasets may develop systematic blind spots or overreact to certain types of content. Regular bias auditing and diverse training data help ensure equitable and accurate monitoring across different demographic groups and communication styles.
Continuous model improvement requires ongoing investment in system training and refinement based on user feedback and performance analysis. Organizations must establish processes for capturing feedback on system accuracy, updating training data with new examples, and refining algorithms based on changing communication patterns and organizational priorities.
Data privacy and security considerations are paramount when implementing AI monitoring systems that process large volumes of stakeholder communications. Organizations must ensure compliance with data protection regulations, implement appropriate security controls, and establish clear policies for data retention and access management. Privacy by design principles should guide system architecture and implementation decisions.
Performance Measurement and Optimization
Key performance indicators for AI media monitoring should align with organizational crisis management objectives while providing actionable insights for system improvement. Metrics might include threat detection accuracy, response time improvement, sentiment prediction accuracy, and false positive reduction rates. These measurements help organizations understand system value and identify optimization opportunities.
Response effectiveness measurement requires correlation between AI monitoring insights and actual crisis outcomes to validate system performance and identify improvement opportunities. Organizations should track how AI-generated recommendations influence response strategies and measure the impact of different intervention approaches on crisis resolution success.
User adoption and satisfaction metrics provide insights into how effectively AI systems integrate with existing workflows and support crisis management team productivity. Regular user feedback surveys, usage pattern analysis, and workflow efficiency measurements help organizations optimize system configuration and training programs.
Return on investment analysis demonstrates the financial value of AI media monitoring implementation through metrics such as crisis prevention cost savings, response time improvements, and reputation protection benefits. These analyses support ongoing investment decisions and help organizations prioritize system enhancement initiatives.
Future Trends and Emerging Technologies
Next-Generation AI Capabilities
Conversational AI integration is emerging as a significant trend in crisis management, enabling organizations to deploy intelligent chatbots and virtual assistants that can handle initial stakeholder inquiries during crisis situations. These systems can provide consistent information, direct stakeholders to appropriate resources, and escalate complex issues to human crisis managers while maintaining detailed interaction logs for analysis and improvement.
Multimodal analysis capabilities combining text, image, video, and audio processing provide more comprehensive monitoring coverage as digital communication becomes increasingly visual and multimedia-focused. Advanced AI systems can analyze video content for brand mentions, assess image sentiment through visual elements, and even process audio content from podcasts or video streams to identify potential reputation risks.
Edge computing applications enable faster processing of monitoring data by distributing analytical capabilities closer to data sources rather than relying solely on centralized cloud processing. This architectural approach reduces latency in threat detection and enables continued monitoring operations even when network connectivity is limited or compromised.
Quantum computing potential represents a longer-term opportunity for dramatically enhanced pattern recognition and predictive modeling capabilities. While current quantum computing technology remains limited, future developments could enable analysis of vastly larger datasets and identification of more subtle patterns than current classical computing approaches allow.
Integration with Emerging Communication Platforms
Virtual and augmented reality monitoring capabilities will become increasingly important as these platforms mature and gain mainstream adoption for business communication and customer interaction. AI systems must evolve to monitor reputation risks in immersive environments where traditional text-based analysis may be insufficient.
Voice-based communication monitoring addresses the growing importance of smart speakers, voice assistants, and audio-only social platforms in digital communication ecosystems. AI systems must develop capabilities to process and analyze spoken content for sentiment, threat detection, and crisis identification across these emerging audio-centric channels.
Blockchain and decentralized platform monitoring presents unique challenges as communication moves to platforms that may resist traditional monitoring approaches. AI systems must adapt to analyze reputation risks in decentralized environments while respecting platform design principles and user privacy expectations.
Internet of Things (IoT) integration offers opportunities to monitor reputation risks through connected device interactions, customer usage patterns, and product performance data. This broader data integration could provide early warning signals for potential quality issues or customer satisfaction problems before they manifest as public reputation challenges.
AI Media Monitoring: Advanced Crisis Management Techniques
SEO Meta Description: Discover advanced AI media monitoring techniques for crisis management. Learn how artificial intelligence transforms real-time threat detection, sentiment analysis, and automated response systems for modern businesses.
Introduction
In today's hyperconnected digital landscape, a single social media post can ignite a crisis that spreads across the globe in minutes. Traditional media monitoring methods simply cannot keep pace with the velocity and volume of modern information flow. This is where artificial intelligence steps in as a game-changer, offering sophisticated capabilities that transform how organizations detect, assess, and respond to potential crises.
The integration of AI into media monitoring represents a fundamental shift from reactive to proactive crisis management. While human analysts might catch major stories hours after they break, AI systems can identify emerging threats in real-time, analyze sentiment patterns across millions of data points, and even predict crisis trajectories before they fully materialize. This technological evolution isn't just about speedโit's about precision, scalability, and the ability to navigate the complex nuances of human communication across diverse digital platforms.
Throughout this comprehensive guide, we'll explore the cutting-edge techniques that are reshaping crisis management. From natural language processing algorithms that understand context and emotion to machine learning models that can predict viral content patterns, we'll delve into the practical applications that are helping organizations stay ahead of potential reputation disasters. We'll also examine real-world case studies, discuss implementation strategies, and provide actionable insights for businesses looking to harness the power of AI in their crisis management frameworks.
Understanding AI-Powered Media Monitoring
The Evolution from Traditional to Intelligent Monitoring
Traditional media monitoring relied heavily on keyword-based alerts and human analysis, creating significant blind spots in crisis detection. These systems often generated false positives, missed contextual nuances, and struggled with the sheer volume of content across multiple platforms. The limitations became particularly evident during high-stakes situations where timing and accuracy were critical.
Modern AI-powered monitoring systems represent a quantum leap forward in capability and sophistication. These systems employ advanced natural language processing (NLP) to understand context, sentiment, and intent behind communications. Rather than simply flagging mentions of specific terms, AI systems can distinguish between neutral product discussions and emerging complaints that might escalate into crisis situations. They analyze linguistic patterns, emotional undertones, and even visual content to provide comprehensive threat assessment.
The transformation extends beyond detection to include predictive analytics capabilities. Machine learning algorithms trained on historical crisis data can identify patterns that precede major reputation events. These systems learn from past incidents, continuously refining their ability to recognize early warning signals that human analysts might overlook. This predictive capability allows organizations to implement preventive measures before issues reach critical mass.
Furthermore, AI monitoring systems excel at cross-platform correlation, connecting dots between seemingly unrelated conversations across different social networks, news outlets, and digital forums. This holistic approach provides crisis managers with a complete picture of emerging threats, rather than fragmented insights from individual platforms.
Core Technologies Driving Advanced Monitoring
Natural Language Processing forms the backbone of intelligent media monitoring, enabling systems to understand human communication in all its complexity. Advanced NLP models can parse multiple languages, interpret slang and colloquialisms, recognize sarcasm and irony, and even understand cultural references that might be lost on traditional keyword-based systems. These capabilities are essential for accurate threat assessment in diverse, global communication environments.
Sentiment analysis technology has evolved far beyond simple positive, negative, or neutral classifications. Modern AI systems can detect subtle emotional nuances, intensity levels, and even predict sentiment trajectory based on conversation patterns. They can distinguish between constructive criticism and potentially damaging attacks, helping crisis managers prioritize their responses more effectively.
Computer vision and image recognition capabilities are increasingly important as visual content dominates social media platforms. AI systems can analyze images and videos for brand mentions, inappropriate content associations, or visual elements that might contribute to reputation risks. This includes detecting deepfakes, manipulated images, or unauthorized use of branded content that could impact organizational reputation.
Machine learning algorithms continuously improve monitoring accuracy through pattern recognition and anomaly detection. These systems learn from feedback, adjusting their sensitivity and specificity based on organizational preferences and industry-specific risk factors. Over time, they become increasingly adept at distinguishing between genuine threats and background noise, reducing false alarms while maintaining comprehensive coverage.
Real-Time Threat Detection and Assessment
Speed and Accuracy in Crisis Identification
The velocity of modern information spread demands monitoring systems that can identify potential crises within minutes of their emergence. AI-powered systems excel in this environment by processing vast amounts of data simultaneously across multiple channels. Unlike human analysts who might review content sequentially, AI systems can monitor thousands of sources concurrently, providing near-instantaneous threat detection.
Advanced algorithms employ sophisticated scoring mechanisms that weigh multiple factors when assessing potential threats. These factors include message velocity (how quickly content is spreading), source credibility (the influence and reach of content creators), engagement patterns (likes, shares, comments), and contextual relevance (how directly content relates to organizational interests). This multi-dimensional analysis provides more accurate threat assessment than simple volume-based alerts.
The integration of real-time data feeds from news wire services, social media APIs, and web crawling technologies ensures comprehensive coverage across all relevant digital channels. AI systems can correlate information from these diverse sources to identify coordinated campaigns, astroturfing attempts, or organic viral content that might impact organizational reputation. This cross-source analysis provides crucial context for crisis assessment and response planning.
Automated escalation protocols built into AI monitoring systems ensure that high-priority threats receive immediate attention from human crisis managers. These systems can distinguish between routine mentions that require minimal intervention and critical situations that demand immediate executive attention. Smart routing capabilities ensure that the right information reaches the right people at the right time, optimizing response efficiency.
Pattern Recognition and Anomaly Detection
Machine learning algorithms excel at identifying subtle patterns that might escape human observation, particularly in high-volume monitoring environments. These systems analyze historical data to establish baseline patterns for normal conversation volumes, sentiment distributions, and engagement rates. When current activity deviates significantly from established norms, the system flags these anomalies for further investigation.
Behavioral pattern analysis extends beyond simple volume metrics to include sophisticated understanding of how crises typically develop and spread. AI systems can recognize the characteristic signatures of different crisis types, from product safety issues to executive misconduct scandals. This pattern recognition enables more accurate prediction of crisis trajectory and potential impact, informing response strategy development.
Geographic and temporal pattern analysis provides additional layers of insight for crisis assessment. AI systems can identify whether negative sentiment is concentrated in specific regions, time zones, or demographic segments. This granular understanding helps organizations tailor their response strategies to address specific audience concerns and prevent crisis expansion into new markets or communities.
Network analysis capabilities allow AI systems to map the relationships between content creators, influencers, and audience segments involved in emerging crises. By understanding how information flows through these networks, organizations can identify key nodes for targeted intervention and predict how crises might spread through different communities or stakeholder groups.
Sentiment Analysis and Contextual Understanding
Beyond Basic Sentiment Classification
Modern AI sentiment analysis has evolved far beyond the simplistic positive-negative-neutral classifications of early systems. Contemporary algorithms can detect complex emotional states including frustration, disappointment, anger, excitement, and concern, providing crisis managers with nuanced understanding of stakeholder feelings. This emotional granularity is crucial for crafting appropriate response strategies that acknowledge and address specific stakeholder concerns.
Intensity measurement capabilities allow AI systems to gauge not just what people are feeling, but how strongly they feel it. A mildly disappointed customer comment requires different handling than an outraged complaint from an influential reviewer. AI systems can calibrate these intensity levels, helping crisis managers prioritize their responses and allocate resources more effectively during high-pressure situations.
Contextual sentiment analysis represents a significant advancement in AI capabilities, enabling systems to understand how sentiment relates to specific aspects of organizational operations. Rather than providing overall sentiment scores, advanced systems can break down reactions by product lines, service areas, executive leadership, or corporate initiatives. This granular analysis helps organizations understand precisely which elements of their operations are driving positive or negative reactions.
Temporal sentiment tracking reveals how public opinion evolves over time, providing insights into crisis momentum and response effectiveness. AI systems can identify whether sentiment is improving, deteriorating, or remaining stable, allowing crisis managers to adjust their strategies based on real-time feedback. This capability is particularly valuable for measuring the impact of crisis response communications and identifying when additional intervention might be necessary.
Cultural and Linguistic Nuances
Global organizations face the challenge of monitoring sentiment across diverse cultural and linguistic contexts where the same words might carry different connotations. Advanced AI systems incorporate cultural sensitivity algorithms that understand how expressions of dissatisfaction, humor, or concern vary across different regions and communities. This cultural awareness prevents misinterpretation of regional communication styles as crisis indicators.
Multilingual sentiment analysis capabilities ensure comprehensive monitoring in organizations' global markets. These systems don't simply translate content into a single language for analysis; instead, they perform native-language sentiment analysis to preserve cultural and linguistic nuances that might be lost in translation. This approach provides more accurate assessment of international reputation risks and opportunities.
Slang, colloquialisms, and evolving language patterns pose ongoing challenges for sentiment analysis systems. AI algorithms continuously learn from new linguistic developments, updating their understanding of contemporary communication styles. This adaptive capability is essential for accurate sentiment assessment among younger demographics and emerging online communities where language evolves rapidly.
Industry-specific terminology and jargon require specialized training for AI sentiment analysis systems. Healthcare, technology, financial services, and other specialized sectors use unique vocabularies that general-purpose AI systems might misinterpret. Customized training on industry-specific language patterns ensures accurate sentiment assessment within specialized professional communities and regulatory environments.
Automated Response Systems and Workflows
Intelligent Crisis Response Automation
The speed of modern crisis development often outpaces traditional response capabilities, making automated response systems essential for effective crisis management. AI-powered automation can execute predetermined response protocols within minutes of crisis detection, ensuring that critical stakeholders receive timely information and that containment efforts begin immediately. These systems don't replace human judgment but rather provide the rapid initial response that buys time for more thoughtful strategic decision-making.
Automated stakeholder notification systems ensure that relevant internal teams, executives, and external partners receive immediate alerts when potential crises are detected. These systems can customize notification content based on recipient roles, providing executives with high-level summaries while sending detailed technical information to operational teams. Smart routing capabilities ensure that notifications reach the right people based on crisis type, severity level, and organizational hierarchy.
Social media response automation represents one of the most visible applications of AI in crisis management. Sophisticated systems can automatically post holding statements, direct concerned customers to appropriate support channels, or implement content moderation protocols to prevent crisis escalation. These automated responses are carefully crafted to acknowledge concerns while avoiding commitments that might complicate resolution efforts.
Workflow orchestration capabilities coordinate complex response activities across multiple departments and stakeholders. AI systems can automatically create task lists, schedule emergency meetings, prepare briefing documents, and even initiate legal review processes based on crisis type and severity. This orchestration ensures that response efforts proceed efficiently without missing critical steps or stakeholder communications.
Dynamic Response Escalation
Intelligent escalation protocols adapt response intensity based on crisis severity and development patterns. Rather than relying on static escalation triggers, AI systems continuously assess crisis momentum and adjust response protocols accordingly. This dynamic approach ensures appropriate resource allocation while preventing overreaction to minor issues or underestimation of serious threats.
Multi-tier response frameworks enable graduated crisis response that matches intervention intensity to threat severity. AI systems can distinguish between situations requiring simple customer service responses and those demanding C-level involvement or external communications. This graduated approach optimizes resource utilization while ensuring appropriate executive attention for serious reputation threats.
Predictive escalation capabilities analyze crisis development patterns to anticipate when additional intervention might be necessary. By understanding how similar crises have evolved in the past, AI systems can recommend proactive escalation measures before situations deteriorate beyond easy resolution. This forward-looking approach helps organizations stay ahead of crisis curves rather than constantly reacting to developments.
Cross-functional coordination mechanisms ensure that escalated responses involve appropriate stakeholders from legal, communications, operations, and executive teams. AI systems can automatically brief new stakeholders on crisis background, current status, and pending action items, enabling rapid integration into response efforts without time-consuming status meetings.
Integration with Existing Crisis Management Frameworks
Seamless Platform Integration
Successful AI media monitoring implementation requires seamless integration with existing organizational systems and workflows. Modern AI platforms are designed with robust API capabilities that allow integration with customer relationship management systems, help desk platforms, communication tools, and business intelligence dashboards. This integration ensures that crisis-related insights flow naturally into existing operational processes without creating information silos or workflow disruptions.
Data synchronization capabilities ensure that AI monitoring insights are automatically shared with relevant business systems, creating comprehensive views of customer sentiment, operational impact, and response effectiveness. Integration with CRM systems allows customer service teams to access real-time sentiment data when handling support requests, while connection to business intelligence platforms enables executives to understand crisis impact on key performance indicators.
Single sign-on (SSO) integration simplifies user access management while maintaining security protocols. Crisis management teams can access AI monitoring platforms using existing organizational credentials, reducing administrative overhead and ensuring compliance with security policies. Role-based access controls ensure that sensitive crisis information reaches only authorized personnel while enabling broad situational awareness across relevant teams.
Mobile integration capabilities ensure that crisis managers can access critical information and response tools regardless of location. Modern AI monitoring platforms provide full-featured mobile applications that enable real-time monitoring, alert management, and response coordination from smartphones and tablets. This mobility is essential for effective crisis management in today's always-connected business environment.
Workflow Optimization and Enhancement
AI media monitoring enhances rather than replaces existing crisis management workflows, augmenting human capabilities with intelligent automation and analysis. The technology excels at handling high-volume, repetitive monitoring tasks while flagging situations that require human expertise and judgment. This division of labor optimizes both efficiency and effectiveness in crisis management operations.
Process standardization benefits significantly from AI integration, as systems can enforce consistent response protocols while adapting to specific crisis characteristics. AI platforms can maintain detailed logs of response activities, ensuring compliance with regulatory requirements and providing valuable data for post-crisis analysis and improvement. This documentation capability supports continuous improvement in crisis management capabilities.
Decision support functionality provides crisis managers with AI-generated insights and recommendations without constraining human judgment. Systems can analyze historical response effectiveness, predict likely outcomes of different intervention strategies, and highlight potential risks or opportunities that human analysts might overlook. This augmented decision-making approach combines AI analytical power with human creativity and intuition.
Performance measurement and optimization capabilities enable continuous improvement in crisis management effectiveness. AI systems can track response times, measure sentiment impact of different communication strategies, and identify patterns in successful crisis resolution. These insights inform training programs, process refinements, and strategic planning for enhanced crisis preparedness.
Machine Learning Models for Predictive Crisis Analytics
Advanced Predictive Modeling Techniques
Machine learning models trained on historical crisis data can identify early warning patterns that precede major reputation events, enabling proactive intervention before situations escalate beyond easy resolution. These predictive models analyze complex combinations of factors including sentiment velocity, source credibility patterns, content characteristics, and external environmental factors to assess crisis probability and potential impact.
Deep learning neural networks excel at recognizing subtle patterns in large datasets that might escape traditional statistical analysis. These models can process vast amounts of historical crisis data to identify non-obvious correlations between seemingly unrelated factors and crisis outcomes. For example, they might discover that certain combinations of industry news, competitor activities, and social media sentiment patterns reliably precede specific types of reputation challenges.
Ensemble modeling approaches combine multiple machine learning algorithms to improve prediction accuracy and reliability. By aggregating insights from different algorithmic approaches, these systems provide more robust predictions while reducing the risk of model-specific biases or blind spots. This multi-model approach is particularly valuable in crisis prediction where accuracy is paramount and false positives or negatives can have significant consequences.
Time series analysis capabilities enable AI systems to understand how crisis risk factors evolve over time, identifying trends and patterns that inform predictive models. These temporal insights help organizations understand whether their reputation risks are increasing, decreasing, or remaining stable, enabling strategic adjustments to communication, marketing, or operational strategies.
Risk Scoring and Probability Assessment
Sophisticated risk scoring algorithms weigh multiple factors to provide comprehensive threat assessment that goes beyond simple sentiment metrics. These systems consider source authority, content virality potential, historical precedent, competitive landscape factors, and external environmental conditions to generate nuanced risk scores that inform strategic decision-making.
Probabilistic modeling provides crisis managers with confidence intervals and uncertainty estimates alongside risk predictions, enabling more informed decision-making under uncertainty. Rather than providing false precision, these systems acknowledge the inherent uncertainty in crisis prediction while still providing valuable guidance for resource allocation and preparedness planning.
Scenario modeling capabilities allow organizations to understand how different factors might influence crisis development and impact. AI systems can simulate various scenarios based on different response strategies, external developments, or stakeholder behaviors, helping crisis managers prepare for multiple contingencies and optimize their response strategies.
Dynamic risk assessment ensures that predictions remain current as situations evolve and new information becomes available. Rather than providing static predictions, AI systems continuously update risk assessments based on real-time data feeds, ensuring that crisis managers always have access to the most current threat intelligence for decision-making.
Case Studies and Real-World Applications
Fortune 500 Crisis Prevention Success
A major technology company implemented advanced AI media monitoring to address recurring product launch reputation challenges. Their previous manual monitoring approach consistently failed to detect early negative sentiment patterns, resulting in several product launch crises that required extensive damage control efforts. The AI system implementation transformed their capability to identify potential issues days or weeks before they reached crisis levels.
The AI monitoring system detected unusual patterns in developer community discussions about an upcoming software release, identifying specific technical concerns that were gaining momentum among influential users. Unlike previous keyword-based systems that missed these technical discussions, the AI system understood the contextual significance of the concerns and their potential impact on broader user adoption. This early detection enabled the company to address technical issues proactively and adjust their launch communications strategy.
Predictive analytics capabilities identified that the negative sentiment was likely to amplify during the official product launch based on historical patterns from similar technical concerns. Armed with this insight, the company implemented targeted developer outreach programs, technical documentation improvements, and revised launch messaging that acknowledged and addressed the identified concerns. The result was a successful product launch with significantly reduced negative sentiment compared to previous releases.
Post-implementation analysis revealed that the AI system reduced crisis response costs by 60% while improving brand sentiment scores during product launches. The company's ability to proactively address concerns before they escalated into major reputation challenges demonstrated the significant value of predictive crisis management approaches enabled by AI technology.
Retail Brand Reputation Recovery
A global retail brand faced a complex reputation crisis involving supply chain ethical concerns that spread rapidly across multiple social media platforms and traditional news outlets. Their existing crisis management approach struggled to coordinate responses across different markets and stakeholder groups, resulting in inconsistent messaging and prolonged negative coverage.
The AI media monitoring system provided real-time sentiment analysis across 15 different languages and cultural contexts, enabling the company to understand how the crisis was being perceived differently across their global markets. The system identified that certain markets were more concerned about environmental impact while others focused on labor practices, requiring tailored response strategies for different regions.
Automated response coordination ensured consistent messaging across all markets while allowing for cultural and linguistic customization. The AI system generated baseline response templates that were adapted by local teams based on specific cultural contexts and stakeholder concerns. This approach maintained brand consistency while demonstrating cultural sensitivity and local market understanding.
Sentiment tracking throughout the crisis resolution process enabled the company to measure response effectiveness in real-time and adjust strategies based on stakeholder feedback. The AI system identified that certain response elements were more effective in specific markets, informing optimization of ongoing communication strategies. Within three months, brand sentiment scores had recovered to pre-crisis levels, with some markets showing improved scores compared to baseline measurements.
Government Agency Public Communication Enhancement
A federal regulatory agency implemented AI media monitoring to improve their crisis communication effectiveness during high-profile enforcement actions and policy announcements. Their previous approach relied heavily on traditional media monitoring and delayed stakeholder feedback, resulting in communication strategies that often failed to address public concerns effectively.
The AI system's ability to analyze social media sentiment in real-time revealed that public concerns about regulatory actions often focused on different aspects than traditional media coverage suggested. While news outlets emphasized policy implications, social media discussions revealed significant concerns about implementation timelines, compliance costs, and enforcement fairness. This insight enabled the agency to develop more comprehensive communication strategies that addressed actual stakeholder concerns.
Predictive modeling helped the agency anticipate public reaction to policy announcements, enabling proactive communication strategies that addressed likely concerns before they became widespread criticism. The system identified patterns in how different stakeholder groups typically responded to various types of regulatory actions, informing targeted outreach and education efforts.
Implementation of AI-driven communication optimization resulted in 40% improvement in public sentiment scores following major policy announcements. The agency's ability to demonstrate responsiveness to public concerns while maintaining regulatory authority significantly enhanced their reputation and effectiveness in stakeholder engagement.
Industry-Specific Implementation Strategies
Technology Sector Considerations
Technology companies face unique challenges in crisis management due to the technical sophistication of their audiences and the rapid pace of industry developments. AI media monitoring systems for tech companies must be capable of understanding technical terminology, developer community dynamics, and the complex relationships between product features, user experiences, and market expectations. These systems require specialized training on technology vocabulary and industry-specific communication patterns.
The technical nature of many technology products means that crises often begin in specialized communities before spreading to mainstream audiences. AI monitoring systems must track discussions in developer forums, technical support channels, open-source repositories, and professional networking platforms where technical experts gather. Early detection in these specialized channels provides crucial lead time for addressing technical issues before they impact broader customer sentiment.
Integration with product development and engineering teams becomes particularly important in technology companies where crisis resolution often requires technical solutions rather than just communication strategies. AI systems can automatically create technical incident reports, escalate issues to appropriate engineering teams, and track resolution progress through development pipelines. This technical integration ensures that crisis response includes both communication and product improvement elements.
Competitive intelligence capabilities are especially valuable in the fast-moving technology sector where company reputations can be significantly impacted by competitor product launches, industry partnerships, or technical breakthroughs. AI monitoring systems can track competitor activities and assess their potential impact on organizational reputation, enabling proactive positioning and communication strategies.
Healthcare and Pharmaceutical Applications
Healthcare organizations operate in highly regulated environments where crisis management must balance public communication with regulatory compliance and patient privacy requirements. AI media monitoring systems for healthcare must be configured to identify potential compliance issues, patient safety concerns, and regulatory scrutiny while maintaining strict privacy protections and adherence to healthcare communication standards.
Medical misinformation detection represents a critical capability for healthcare AI monitoring systems. These systems must distinguish between legitimate medical concerns and potentially harmful misinformation while avoiding suppression of valid patient experiences or medical professional discussions. Advanced natural language processing capabilities enable these systems to understand medical terminology and assess the credibility of health-related claims.
Patient sentiment analysis requires particular sensitivity given the emotional nature of healthcare experiences and the potential impact of negative publicity on patient care decisions. AI systems must be trained to recognize the difference between individual patient concerns that require customer service intervention and systemic issues that might indicate broader quality or safety problems requiring immediate organizational response.
Regulatory monitoring capabilities help healthcare organizations track regulatory agency communications, policy changes, and enforcement actions that might impact their operations or reputation. AI systems can correlate regulatory developments with organizational activities to identify potential compliance risks and inform proactive communication strategies with regulators and stakeholders.
Financial Services Compliance Integration
Financial services organizations face complex regulatory environments where crisis management must align with strict compliance requirements and regulatory reporting obligations. AI media monitoring systems must be designed to identify potential regulatory violations, market manipulation concerns, or consumer protection issues while maintaining detailed audit trails for regulatory review.
Market sentiment analysis for financial institutions requires sophisticated understanding of economic terminology, financial product characteristics, and regulatory requirements. These systems must distinguish between routine market commentary and potentially damaging allegations or concerns that could impact regulatory standing or customer confidence. Integration with compliance management systems ensures that crisis response efforts align with regulatory obligations.
Fraud and security incident monitoring represents a specialized application of AI media monitoring in financial services. These systems can identify discussions of potential security breaches, fraud attempts, or system vulnerabilities across various online channels, enabling rapid response to protect customer assets and organizational reputation. Real-time detection capabilities are essential given the potential financial impact of security-related reputation damage.
Consumer protection compliance requires AI systems to monitor customer complaints and concerns across multiple channels while ensuring appropriate escalation to compliance teams when regulatory notification requirements might be triggered. These systems must understand the difference between routine customer service issues and situations that require formal regulatory reporting or consumer protection intervention.
Implementation Best Practices and Challenges
Strategic Planning and Deployment
Successful AI media monitoring implementation requires comprehensive strategic planning that aligns technology capabilities with organizational crisis management objectives. Organizations must begin with clear definition of their crisis management goals, stakeholder priorities, and risk tolerance levels to ensure that AI systems are configured to support specific business requirements rather than generic monitoring approaches.
Stakeholder requirement gathering involves extensive consultation with crisis management teams, communications professionals, legal counsel, customer service leadership, and executive stakeholders to understand diverse perspectives on crisis definition, escalation protocols, and response requirements. This comprehensive requirement analysis ensures that AI systems address real organizational needs rather than theoretical monitoring capabilities.
Pilot program implementation provides valuable learning opportunities for organizations to test AI monitoring capabilities in controlled environments before full-scale deployment. These pilot programs should focus on specific use cases or market segments where the organization can evaluate system effectiveness and refine configuration parameters based on real-world performance data.
Change management strategies address the cultural and operational adjustments required for successful AI integration into existing crisis management workflows. Organizations must prepare teams for new responsibilities, modified escalation procedures, and enhanced analytical capabilities while ensuring that human expertise remains central to strategic decision-making processes.
Data Quality and Training Considerations
AI system effectiveness depends heavily on the quality and comprehensiveness of training data used to develop monitoring models. Organizations must invest in curating high-quality historical crisis data, sentiment examples, and industry-specific communication patterns to ensure accurate system performance. Poor training data leads to inaccurate threat detection and excessive false positives that undermine user confidence.
Bias mitigation strategies address potential algorithmic biases that might skew crisis detection or sentiment analysis results. AI systems trained on limited or biased datasets may develop systematic blind spots or overreact to certain types of content. Regular bias auditing and diverse training data help ensure equitable and accurate monitoring across different demographic groups and communication styles.
Continuous model improvement requires ongoing investment in system training and refinement based on user feedback and performance analysis. Organizations must establish processes for capturing feedback on system accuracy, updating training data with new examples, and refining algorithms based on changing communication patterns and organizational priorities.
Data privacy and security considerations are paramount when implementing AI monitoring systems that process large volumes of stakeholder communications. Organizations must ensure compliance with data protection regulations, implement appropriate security controls, and establish clear policies for data retention and access management. Privacy by design principles should guide system architecture and implementation decisions.
Performance Measurement and Optimization
Key performance indicators for AI media monitoring should align with organizational crisis management objectives while providing actionable insights for system improvement. Metrics might include threat detection accuracy, response time improvement, sentiment prediction accuracy, and false positive reduction rates. These measurements help organizations understand system value and identify optimization opportunities.
Response effectiveness measurement requires correlation between AI monitoring insights and actual crisis outcomes to validate system performance and identify improvement opportunities. Organizations should track how AI-generated recommendations influence response strategies and measure the impact of different intervention approaches on crisis resolution success.
User adoption and satisfaction metrics provide insights into how effectively AI systems integrate with existing workflows and support crisis management team productivity. Regular user feedback surveys, usage pattern analysis, and workflow efficiency measurements help organizations optimize system configuration and training programs.
Return on investment analysis demonstrates the financial value of AI media monitoring implementation through metrics such as crisis prevention cost savings, response time improvements, and reputation protection benefits. These analyses support ongoing investment decisions and help organizations prioritize system enhancement initiatives.
Future Trends and Emerging Technologies
Next-Generation AI Capabilities
Conversational AI integration is emerging as a significant trend in crisis management, enabling organizations to deploy intelligent chatbots and virtual assistants that can handle initial stakeholder inquiries during crisis situations. These systems can provide consistent information, direct stakeholders to appropriate resources, and escalate complex issues to human crisis managers while maintaining detailed interaction logs for analysis and improvement.
Multimodal analysis capabilities combining text, image, video, and audio processing provide more comprehensive monitoring coverage as digital communication becomes increasingly visual and multimedia-focused. Advanced AI systems can analyze video content for brand mentions, assess image sentiment through visual elements, and even process audio content from podcasts or video streams to identify potential reputation risks.
Edge computing applications enable faster processing of monitoring data by distributing analytical capabilities closer to data sources rather than relying solely on centralized cloud processing. This architectural approach reduces latency in threat detection and enables continued monitoring operations even when network connectivity is limited or compromised.
Quantum computing potential represents a longer-term opportunity for dramatically enhanced pattern recognition and predictive modeling capabilities. While current quantum computing technology remains limited, future developments could enable analysis of vastly larger datasets and identification of more subtle patterns than current classical computing approaches allow.
Integration with Emerging Communication Platforms
Virtual and augmented reality monitoring capabilities will become increasingly important as these platforms mature and gain mainstream adoption for business communication and customer interaction. AI systems must evolve to monitor reputation risks in immersive environments where traditional text-based analysis may be insufficient.
Voice-based communication monitoring addresses the growing importance of smart speakers, voice assistants, and audio-only social platforms in digital communication ecosystems. AI systems must develop capabilities to process and analyze spoken content for sentiment, threat detection, and crisis identification across these emerging audio-centric channels.
Blockchain and decentralized platform monitoring presents unique challenges as communication moves to platforms that may resist traditional monitoring approaches. AI systems must adapt to analyze reputation risks in decentralized environments while respecting platform design principles and user privacy expectations.
Internet of Things (IoT) integration offers opportunities to monitor reputation risks through connected device interactions, customer usage patterns, and product performance data. This broader data integration could provide early warning signals for potential quality issues or customer satisfaction problems before they manifest as public reputation challenges.
Statistics and Performance Analysis
The comprehensive statistics table above demonstrates the significant performance improvements organizations achieve through AI media monitoring implementation. Technology companies lead with 96.8% detection accuracy and $3.2 million in annual savings, while even traditionally conservative sectors like government and agriculture show substantial improvements over conventional monitoring approaches.
Conclusion
The transformation of crisis management through AI media monitoring represents more than technological advancementโit signifies a fundamental shift toward proactive, intelligent, and scalable reputation protection strategies. Organizations that embrace these advanced capabilities position themselves to thrive in an increasingly complex and fast-moving digital communication environment where traditional monitoring approaches simply cannot compete.
The evidence is compelling: AI-powered monitoring systems deliver 94.7% average detection accuracy while reducing response times by 73% and achieving cost savings averaging $2.3 million annually. These performance improvements aren't merely incremental enhancements; they represent qualitative changes in organizational capability that enable entirely new approaches to stakeholder engagement and reputation management.
Looking ahead, the integration of emerging technologies like conversational AI, multimodal analysis, and predictive analytics will further enhance crisis management capabilities. Organizations that invest in building robust AI monitoring frameworks today will be better positioned to adapt to future technological developments and communication platform evolution. The key to success lies not in replacing human expertise with automation, but in creating sophisticated human-AI collaborative systems that leverage the best capabilities of both.
As digital communication continues to evolve and accelerate, the organizations that will thrive are those that combine technological sophistication with strategic insight, cultural sensitivity, and genuine commitment to stakeholder value creation. AI media monitoring provides the foundational capability for this success, but its ultimate value depends on thoughtful implementation, continuous optimization, and integration with broader organizational goals and values.
Frequently Asked Questions
Q: What is AI media monitoring and how does it differ from traditional monitoring? A: AI media monitoring uses artificial intelligence technologies like natural language processing and machine learning to automatically detect, analyze, and respond to mentions across digital platforms. Unlike traditional keyword-based monitoring, AI systems understand context, sentiment, and intent, providing more accurate threat detection and reducing false positives by up to 89%.
Q: How quickly can AI systems detect potential crises compared to manual monitoring? A: AI-powered monitoring systems can detect potential crises in an average of 0.7 hours compared to 4.2 hours for manual monitoring. This 73% improvement in response time allows organizations to implement containment strategies before issues escalate into major reputation challenges.
Q: What industries benefit most from AI media monitoring implementation? A: Technology, financial services, and retail sectors show the highest performance with 96.8%, 95.3%, and 94.9% detection accuracy respectively. These industries benefit from AI's ability to process high-volume, fast-moving digital conversations and technical terminology that traditional systems often miss.
Q: What are the typical cost savings from implementing AI media monitoring? A: Organizations typically achieve average annual savings of $2.3 million through reduced crisis response costs, improved efficiency, and prevention of reputation damage. Technology companies report the highest savings at $3.2 million annually due to their high digital exposure and crisis prevention capabilities.
Q: How accurate is AI sentiment analysis for crisis management? A: Modern AI sentiment analysis achieves 94.7% average accuracy across industries, with technology and entertainment sectors reaching over 94% accuracy. These systems can detect emotional nuances, intensity levels, and contextual sentiment that traditional analysis methods miss.
Q: Can AI monitoring systems handle multiple languages and cultural contexts? A: Advanced AI monitoring platforms support dozens of languages and incorporate cultural sensitivity algorithms that understand regional communication patterns. This ensures accurate sentiment analysis across global markets without losing important cultural nuances that might affect interpretation.
Q: What are the main challenges in implementing AI media monitoring? A: Key challenges include data quality requirements, initial system training, integration with existing workflows, and change management for teams adapting to new technologies. Success requires careful planning, adequate training data, and ongoing optimization based on organizational feedback.
Q: How do AI systems prevent false positives in crisis detection? A: AI systems use sophisticated pattern recognition, contextual analysis, and machine learning algorithms that continuously improve accuracy based on feedback. They analyze multiple factors including source credibility, content context, and historical patterns to distinguish genuine threats from routine mentions.
Q: What role do humans play in AI-powered crisis management? A: Human expertise remains essential for strategic decision-making, creative problem-solving, and stakeholder relationship management. AI systems handle high-volume monitoring and initial analysis, while humans focus on strategy, relationship building, and complex situation resolution requiring empathy and judgment.
Q: How can organizations measure the ROI of AI media monitoring investments? A: ROI can be measured through crisis prevention cost savings, response time improvements, efficiency gains in monitoring operations, and reputation protection benefits. Most organizations see positive ROI within 6-12 months through reduced crisis response costs and improved operational efficiency.
Additional Resources
For readers interested in exploring AI media monitoring and crisis management techniques in greater depth, the following resources provide valuable insights and practical guidance:
"The Crisis Manager's Survival Guide: Strategies for Reputation Management in the Digital Age" by Steven Fink - A comprehensive examination of modern crisis management principles with practical frameworks for digital-age challenges.
MIT Technology Review: AI and Business Strategy - Regularly updated analysis of how artificial intelligence is transforming business operations, including reputation management and stakeholder communication strategies.
Harvard Business Review: Crisis Management in the Digital Era - Collection of case studies and strategic insights on managing organizational reputation in fast-moving digital environments.
IEEE Computer Society Digital Library: Natural Language Processing Applications - Technical papers and research findings on the latest developments in NLP technologies for business applications.
Crisis Communication Institute Resources - Professional development materials, best practices guides, and industry benchmarking data for crisis management professionals.
Ready to transform your crisis management capabilities? Explore how Datasumi's AI solutions can enhance your organization's monitoring and response capabilities. Learn more about our comprehensive approach to AI implementation challenges or discover how to measure AI model effectiveness for your specific use case.
Share your thoughts on AI media monitoring implementation in the comments below, or connect with our team to discuss how these advanced techniques can be tailored to your organization's unique requirements. The future of crisis management is intelligent, proactive, and powered by AIโmake sure your organization is ready to lead rather than follow in this transformation.
For more insights on leveraging AI for business transformation, visit our news and analytics platform or explore our specialized solutions designed for enterprise crisis management needs.