AI-Powered Business Intelligence Techniques
iscover how AI-powered business intelligence visualisation transforms complex data into actionable insights. Learn advanced techniques to enhance decision-making, identify hidden patterns, and drive strategic growth in today's data-rich business environment.
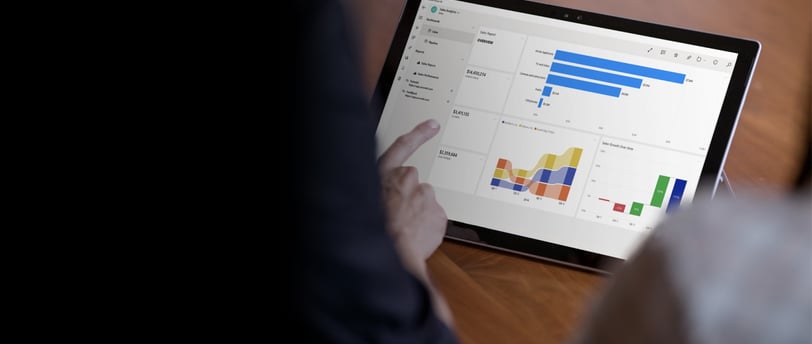
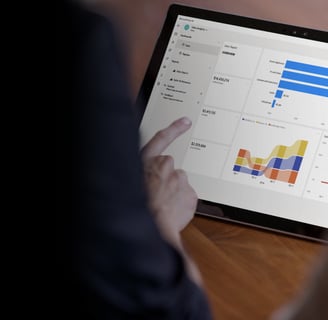
In today's digital landscape, businesses are drowning in data but starving for insights. Every click, transaction, and interaction generates valuable information, but the sheer volume has created a paradox: we have more data than ever before, yet translating it into meaningful business decisions remains a significant challenge. This is where the convergence of artificial intelligence and business intelligence visualization emerges as a game-changing solution. AI-powered visualization techniques are revolutionizing how organizations interpret complex datasets, uncovering hidden patterns and trends that traditional analysis might miss. The evolution from static charts to dynamic, intelligent visualizations represents a quantum leap in how businesses understand their operations, customers, and market opportunities. In this comprehensive guide, we'll explore cutting-edge visualization approaches that leverage AI to transform raw data into strategic assets, examining both the technological innovations driving this revolution and the practical applications that are helping companies gain competitive advantages across industries.
The Evolution of Business Intelligence Visualization
Business intelligence visualization has undergone a remarkable transformation over the past several decades, evolving from simple paper reports to sophisticated interactive dashboards powered by artificial intelligence. In the early days of business analytics, organizations relied heavily on static tables and basic charts that required significant manual effort to create and interpret. These traditional tools, while useful for their time, offered limited insights and required specialized knowledge to generate meaningful conclusions from the data presented. The introduction of digital dashboards in the 1990s marked a significant advancement, allowing for more dynamic representations of data and the ability to update visualizations as new information became available. This period saw the rise of pie charts, bar graphs, and line charts as standard tools in the business intelligence toolkit, providing executives with clearer pictures of organizational performance across various metrics.
The dawn of the 21st century brought about the era of interactive visualization, enabling users to drill down into data points, filter information on demand, and customize their views according to specific business questions. This shift democratized data analysis, allowing non-technical users to explore information independently without relying exclusively on IT departments or data scientists. Cloud computing further accelerated this evolution, making sophisticated visualization tools accessible to organizations of all sizes and eliminating many of the hardware constraints that previously limited analytical capabilities. The introduction of mobile-responsive dashboards ensured that decision-makers could access critical business intelligence on any device, anywhere in the world, fundamentally changing how and when strategic decisions could be made based on data insights.
Today, we stand at the frontier of the AI-driven visualization revolution, where machine learning algorithms enhance every aspect of the data visualization process. Modern systems can automatically identify the most relevant data points, suggest optimal visualization types, and even generate natural language explanations of complex trends detected in the underlying data. These AI capabilities have transformed business intelligence from a retrospective reporting tool into a predictive and prescriptive platform that anticipates business challenges and opportunities before they fully materialize. The integration of augmented analytics has further enhanced this evolution, combining automated insights with human expertise to create visualization experiences that amplify organizational intelligence rather than simply representing information in a visually appealing format.
How AI is Transforming Data Visualization
Artificial intelligence has fundamentally reimagined what's possible in business intelligence visualization, shifting the paradigm from passive data representation to active insight generation. The most transformative aspect of AI-powered visualization lies in its ability to process and analyze massive datasets at scales far beyond human capability, identifying complex patterns, correlations, and anomalies that would otherwise remain hidden. Traditional visualization tools frequently struggled with big data challenges, often becoming sluggish or unmanageable when attempting to process millions or billions of data points. AI algorithms, by contrast, excel at handling enormous datasets, automatically identifying the most relevant subsets of information and determining the optimal level of aggregation or detail needed for meaningful visualization. This capability has made previously unwieldy datasets accessible and actionable for businesses across sectors ranging from healthcare and finance to retail and manufacturing.
Natural language processing (NLP) represents another revolutionary AI contribution to business intelligence visualization, enabling conversational interactions with data through intuitive voice or text commands. Users can now simply ask questions like "Show me sales performance by region for the last quarter" or "Which products have seen declining performance year-over-year?" and receive instant visualizations that answer their specific queries. This conversational approach has dramatically lowered the technical barriers to data exploration, allowing executives, managers, and frontline employees to engage with business intelligence without specialized training in data analysis or visualization tools. The democratization effect has accelerated organizational adoption of data-driven decision-making, with research from Datasumi showing that companies implementing conversational analytics see 34% higher employee engagement with business intelligence tools.
Machine learning algorithms have also transformed visualization by introducing predictive elements that extend beyond historical data representation. Modern AI-powered dashboards can now project trends, forecast outcomes, and simulate scenarios based on historical patterns and external variables. These predictive visualizations enable decision-makers to explore potential futures rather than simply analyzing the past, fundamentally changing how businesses approach strategic planning and risk management. Additionally, AI systems can continuously learn from user interactions, refining visualizations based on which representations prove most valuable for specific users or decisions. This personalization capability ensures that visualization interfaces evolve to match the unique cognitive preferences and decision-making processes of individual users, maximizing the impact of visual data communication across diverse teams and organizational levels.
Key Advanced Visualization Techniques
Predictive Analytics Visualizations
Predictive visualization represents one of the most powerful applications of AI in business intelligence, transforming static representations of historical data into dynamic projections of future scenarios. These sophisticated tools leverage machine learning algorithms to identify patterns in historical data and extend them forward, creating visual forecasts that help businesses anticipate market shifts, customer behaviors, and operational challenges. Time-series forecasting visualizations, for example, can display projected revenue streams, inventory requirements, or resource utilization with clearly defined confidence intervals that communicate the level of certainty associated with each prediction. The visual presentation of these forecasts makes complex statistical concepts accessible to business users without specialized analytical training, enabling more widespread use of predictive insights in day-to-day decision making. Interactive elements allow users to adjust variables and immediately see how different assumptions might affect outcomes, creating a sandbox for strategic planning that combines analytical rigor with intuitive visual exploration.
The most advanced predictive visualizations incorporate multiple data sources and variables to create multidimensional models that capture the complex interdependencies driving business outcomes. These visualizations might present heat maps showing the probability of customer churn across different segments, bubble charts displaying the projected impact of marketing investments across channels, or network diagrams revealing emerging risks in supply chains. What makes these visualizations particularly valuable is their ability to highlight the factors most strongly influencing predicted outcomes, helping decision-makers understand not just what might happen but why it might happen. A study by Datasumi's research team found that companies using advanced predictive visualizations reduced forecast errors by an average of 23% compared to traditional forecasting methods, leading to improved inventory management and resource allocation.
Anomaly detection visualizations represent another cutting-edge application, using AI to automatically identify and highlight unusual patterns or outliers that warrant attention. These visualizations can transform overwhelming data landscapes into focused views that direct attention to the most significant deviations from expected patterns. Real-time monitoring dashboards enhanced with anomaly detection can visualize fraud attempts as they occur, highlight quality control issues on manufacturing lines, or identify unusual customer behaviors that represent either risks or opportunities. The combination of automated detection algorithms with intuitive visual cues creates an early warning system that helps organizations respond proactively to emerging issues before they escalate into major problems. This capability is particularly valuable in high-velocity data environments where human analysts would be unable to manually monitor all relevant metrics and identify subtle deviations in real time.
Natural Language Processing for Data Exploration
Natural Language Processing (NLP) has revolutionized how users interact with visualization systems, creating intuitive interfaces that respond to everyday language rather than requiring specialized query syntax or technical expertise. These conversational visualization platforms enable users to simply type or speak questions such as "Show me our top-performing products in emerging markets over the last year" and instantly receive relevant visual representations that answer their specific queries. The AI-powered systems interpret the intent behind these natural language requests, identify the relevant data sources, determine appropriate visualization types, and generate customized charts, graphs, or dashboards on demand. This dramatic simplification of the data exploration process has expanded access to business intelligence beyond traditional analyst roles, empowering decision-makers across all organizational levels to independently investigate business questions as they arise. The reduced friction between having a question and visualizing the answer has accelerated insight generation and created more agile, data-responsive organizations.
Automated insight narratives represent another powerful application of NLP in business intelligence visualization, providing natural language summaries that explain the significance of visual data patterns. These AI-generated narratives accompany charts and graphs with contextual explanations, highlighting key trends, anomalies, and potential implications that might otherwise require expert interpretation. For example, alongside a visualization showing regional sales performance, the system might automatically generate text explaining: "Northeast sales have declined 12% year-over-year, primarily driven by a 24% drop in the enterprise segment, while SMB customers in the same region grew by 8%." These natural language complements enhance understanding of visual data, particularly for users who may struggle with extracting and interpreting complex patterns from charts alone. According to Datasumi's usability research, visualization systems that incorporate automated narratives improve information retention by 37% and reduce the time required to reach accurate conclusions by 28%.
Sentiment analysis visualization combines NLP with advanced display techniques to create powerful tools for understanding emotional and opinion-based data. These visualizations transform unstructured text from sources like customer reviews, social media posts, support tickets, or employee feedback into structured visual representations that reveal emotional patterns and attitude shifts. Color-coded sentiment maps can display customer satisfaction levels across different product lines, animated time-series visualizations can track brand perception changes following marketing campaigns, and word clouds weighted by sentiment scores can highlight the most emotionally charged topics in customer communications. The integration of sentiment scoring with traditional business metrics creates multidimensional visualizations that connect emotional responses to tangible business outcomes, helping organizations understand how subjective factors influence objective results. This capability is particularly valuable for brand managers, customer experience teams, and product developers who need to understand not just what customers are doing, but how they're feeling about their experiences.
Interactive Dashboards and Real-time Analytics
Interactive dashboards represent the command centers of modern business intelligence, consolidating multiple visualizations into cohesive interfaces that support comprehensive situation awareness and coordinated decision-making. The latest generation of AI-enhanced dashboards transcends static reporting by offering dynamic, personalized experiences that adapt to user needs and evolving business conditions. These interfaces typically feature linked visualizations where interactions with one chart automatically update related displays, creating an intuitive exploration environment that maintains contextual connections across different data dimensions. Drill-down capabilities allow users to seamlessly transition from high-level overviews to granular details, investigating the specific factors driving broader trends without losing their analytical flow. Cross-filtering enables exploration of relationships between different metrics and dimensions, such as examining how marketing channel performance varies across customer segments or geographic regions. The most sophisticated dashboards incorporate AI-driven recommendations that suggest relevant explorations based on user behavior and data patterns, actively guiding users toward valuable insights they might otherwise miss.
Real-time analytics visualization has transformed business intelligence from a retrospective activity into a continuous monitoring and response system that visualizes data as it's generated. These dynamic visualizations update automatically as new information becomes available, enabling immediate identification of emerging opportunities or developing problems. Advanced streaming analytics platforms can process and visualize millions of events per second, supporting use cases like network security monitoring that displays attack patterns as they develop, manufacturing quality control that visualizes production anomalies in real time, or financial trading platforms that visualize market movements and algorithmically identified opportunities. The combination of real-time data processing with AI-powered anomaly detection creates particularly powerful visualization systems that automatically highlight significant deviations from expected patterns, directing attention to the most important signals within noisy data streams. A case study published by Datasumi demonstrated how a telecommunications provider reduced network outage response times by 64% after implementing real-time visualization of network performance metrics with AI-powered anomaly detection.
Geospatial intelligence visualization integrates geographical information with business metrics to create interactive maps that reveal location-based patterns and relationships. These visualizations leverage AI to enhance traditional mapping approaches, automatically identifying spatial clusters, optimizing choropleth color schemes for maximum perceptual accuracy, and integrating multiple data layers into coherent geographic narratives. Modern geospatial visualizations can display complex information like customer density heat maps overlaid with store locations and competitor presence, supply chain network diagrams showing material flows and bottleneck risks, or sales performance maps that highlight regional variations and growth opportunities. The integration of temporal elements creates animated geospatial visualizations that reveal how patterns evolve over time and space, such as the geographic spread of product adoption or the movement of service disruptions across network infrastructures. Mobile-responsive design ensures these visualizations remain effective across devices, allowing field personnel to access location-relevant intelligence wherever they operate and creating alignment between strategic planning and tactical execution.
Augmented Analytics Visualizations
Augmented analytics represents the cutting edge of AI-powered business intelligence, where machine learning algorithms work alongside human analysts to enhance every aspect of the visualization workflow. These systems begin by automating data preparation and feature engineering, intelligently handling missing values, identifying outliers, and transforming variables to optimize them for analysis and visualization. The AI components then evaluate thousands of potential variable combinations and visualization techniques to discover meaningful patterns that human analysts might never have time to explore manually. The most significant patterns are automatically presented through recommended visualizations, with the system prioritizing insights based on statistical significance, business relevance, and user preferences. This augmented approach dramatically accelerates the insight discovery process, allowing analysts to focus their expertise on interpreting and applying findings rather than spending hours on mechanical data preparation and exploration tasks. The continuous learning capabilities of these systems create virtuous improvement cycles where each interaction helps the platform better understand which visualizations and insights provide the most value in specific business contexts.
Automated multivariate analysis visualization tackles one of the most challenging aspects of data exploration: understanding complex relationships involving multiple variables simultaneously. Traditional visualization approaches struggle with high-dimensional data, often requiring analysts to examine many separate two-dimensional charts to piece together a comprehensive understanding of multivariate relationships. AI-powered visualization tools overcome this limitation by automatically identifying the most relevant variable combinations and generating innovative visual representations that capture multidimensional patterns in accessible formats. These might include parallel coordinates plots that visualize how multiple metrics vary across different categories, dimensionality reduction visualizations that project complex relationships into interpretable two-dimensional spaces, or advanced correlation matrices that highlight the strength and direction of relationships between dozens of variables simultaneously. By making multidimensional relationships visually comprehensible, these tools help organizations discover non-obvious connections between seemingly unrelated business factors, such as how combinations of product features, customer characteristics, and support interactions collectively influence retention rates.
Cognitive visualization represents an emerging frontier where AI systems adapt visual presentations based on principles from cognitive psychology and human perception research. These intelligent interfaces consider factors like color theory, visual hierarchy, and cognitive load to optimize how information is presented for maximum comprehension and retention. The systems might automatically adjust color palettes for colorblind users, increase font sizes on mobile devices, or reorganize dashboard elements based on screen size and viewing context. More advanced cognitive visualization platforms track user attention patterns and comprehension indicators, continually refining presentations to match individual cognitive preferences and learning styles. For example, the system might recognize that a particular user responds better to tabular presentations for financial data but prefers graphical formats for operational metrics, automatically adjusting future visualizations accordingly. According to research from Datasumi's UX laboratory, personalized cognitive visualizations improve information comprehension by up to 42% compared to standard visualization approaches, particularly for complex multidimensional data sets commonly encountered in enterprise business intelligence applications.
Implementing AI-Powered Visualization Tools
Successful implementation of AI-powered visualization begins with a clearly defined strategy that aligns analytical capabilities with specific business objectives and user needs. Organizations should avoid the common pitfall of pursuing advanced visualization for its own sake, instead focusing on identifying the critical decisions that would benefit most from enhanced visual analytics. This requires close collaboration between technical teams and business stakeholders to understand the questions that drive strategic and operational choices across different organizational functions. A thorough assessment of existing data assets is equally important, evaluating not just the volume and variety of available information but also its quality, accessibility, and relevance to priority business questions. Many organizations find that creating a data visualization maturity model helps map their current capabilities against future ambitions, creating a structured roadmap for progressive implementation that delivers incremental value while building toward more sophisticated capabilities. The most successful strategies also incorporate governance considerations from the outset, establishing clear protocols for data access, visualization standards, and insight sharing that balance innovation with appropriate controls for sensitive information.
Technical infrastructure considerations play a crucial role in the successful deployment of AI-enhanced visualization platforms. The computational demands of processing large datasets and running complex machine learning algorithms often exceed the capabilities of traditional business intelligence infrastructure, necessitating investments in more robust processing capabilities either on-premises or in the cloud. Organizations increasingly favor cloud-based solutions that offer scalable resources and simplified management, though regulatory requirements or data sovereignty concerns may influence this decision for specific industries or regions. Integration with existing data sources represents another critical technical consideration, with the most effective implementations creating seamless connections to both structured repositories like data warehouses and unstructured sources such as document management systems or communication platforms. The technical architecture should also accommodate both batch and real-time data processing to support a range of visualization needs from historical analysis to live monitoring. According to a Datasumi technical implementation survey, organizations that establish dedicated sandbox environments for testing new visualization approaches before enterprise-wide deployment report 58% higher satisfaction with their advanced analytics initiatives.
User adoption represents perhaps the most crucial factor in realizing value from AI-powered visualization investments. Even the most technically sophisticated visualization platforms deliver minimal business impact if users don't incorporate them into regular decision-making processes. Successful implementations typically include comprehensive training programs tailored to different user personas, from executives who need high-level orientation to power users who require deeper technical understanding. Creating communities of practice where users can share experiences, exchange visualization techniques, and collectively solve analytical challenges significantly accelerates adoption across the organization. Change management efforts should emphasize the specific benefits that enhanced visualization brings to each user group, demonstrating how it makes their work more effective or efficient rather than positioning it as a technical initiative. Many organizations find that designating visualization champions within each business unit helps bridge the gap between technical capabilities and practical applications, providing peer-level support that complements formal training programs. Finally, establishing clear metrics for visualization adoption and impact creates accountability and helps identify areas where additional support or refinement may be needed to fully realize the potential of AI-powered business intelligence.
Case Studies: Success Stories in AI Visualization
Retail Giant Transforms Customer Analytics
A leading multinational retailer facing intense competition from e-commerce platforms leveraged AI-powered visualization to transform their understanding of customer behavior across physical and digital channels. The company integrated data from point-of-sale systems, online interactions, mobile app usage, loyalty programs, and third-party demographic information into a unified customer intelligence platform enhanced with advanced visualization capabilities. Interactive pathway visualizations revealed how customers navigated between online and offline touchpoints, highlighting previously invisible patterns in the omnichannel journey. Predictive visualizations identified customer segments at risk of attrition with specific product categories, while recommendation engines visualized personalization opportunities across the customer base. Store managers accessed geospatial visualizations showing localized preferences and competitive pressures specific to their locations, allowing for customized merchandising strategies. The implementation resulted in a 14% increase in customer retention, 23% improvement in marketing campaign effectiveness, and an 8% lift in average transaction value across targeted segments. Perhaps most significantly, the democratization of these visualizations beyond the central analytics team to frontline managers created a more responsive, customer-centric organization capable of adapting quickly to emerging behavioral trends.
Manufacturing Excellence Through Visual Intelligence
A global industrial manufacturer struggling with inconsistent production quality and rising warranty costs implemented an AI-powered visualization system that transformed their operations. The platform ingested real-time data from thousands of IoT sensors across multiple production facilities, creating interactive visualizations that revealed previously undetected relationships between manufacturing variables and product quality outcomes. Predictive maintenance visualizations identified equipment likely to fail before it caused production disruptions, while process optimization dashboards highlighted opportunities to reduce waste and energy consumption. The system's natural language capabilities allowed factory floor supervisors to ask questions like "Show me the factors most strongly correlated with defect rates on production line B" and receive instant visual answers without requiring database query skills. The implementation delivered a 32% reduction in warranty claims, 18% decrease in unplanned downtime, and 7% improvement in overall equipment effectiveness. According to a detailed case study published by Datasumi, the company attributed their success to the combination of AI-powered pattern detection with visualizations specifically designed for different user roles, from operators needing real-time production metrics to executives tracking facility-wide performance trends.
Financial Services Risk Visualization
A multinational financial services institution facing increasing regulatory scrutiny and sophisticated fraud attempts deployed an advanced visualization platform to transform their risk management capabilities. The system integrated structured transaction data with unstructured information from compliance reports, news feeds, and regulatory updates, creating multidimensional risk visualizations that highlighted emerging threats in real time. Network graph visualizations revealed complex relationships between entities that might indicate money laundering or other financial crimes, automatically highlighting suspicious patterns for further investigation. Anomaly detection algorithms visualized unusual transaction patterns across millions of daily activities, directing analyst attention to the highest-priority concerns while reducing false positives by 67%. Interactive scenario modeling visualizations enabled risk officers to simulate the potential impact of different economic conditions or regulatory changes, visually representing vulnerability exposures across the institution's portfolio. The implementation resulted in a 42% reduction in fraud losses, 28% decrease in compliance investigation time, and significantly improved regulatory audit outcomes. The financial institution's Chief Risk Officer attributed their success to the way visualization transformed abstract risk concepts into tangible, actionable insights that facilitated more effective communication between technical risk specialists and business decision-makers across the organization.
Overcoming Challenges in Advanced Visualization
Effective implementation of AI-powered visualization requires addressing several significant technical and organizational challenges that can otherwise limit adoption and impact. Data quality issues represent one of the most common obstacles, as inconsistent, incomplete, or inaccurate information undermines even the most sophisticated visualization capabilities. Organizations must invest in robust data governance frameworks and quality management processes before expecting meaningful results from advanced visualization initiatives. Integration challenges across disparate data sources also frequently impede progress, particularly in organizations with complex legacy systems or siloed departmental data stores. Successful implementations typically establish unified data architectures that standardize access protocols and semantic definitions across the enterprise. Performance concerns can emerge when visualizing extremely large datasets or implementing complex real-time analytics, requiring careful attention to optimization techniques like data aggregation, caching strategies, and efficient query design. Security and privacy considerations must be addressed from the outset, particularly when visualizations incorporate sensitive customer information or proprietary business data that might create compliance risks if improperly exposed. According to Datasumi's implementation research, organizations that proactively address these technical foundations before focusing on visualization functionality report 47% higher satisfaction with their business intelligence investments.
Cultural and organizational barriers often prove more challenging than technical obstacles when implementing advanced visualization. Resistance to data-driven decision-making remains surprisingly common in organizations with strong traditions of intuition-based leadership or entrenched departmental fiefdoms. Building a data visualization culture requires visible executive sponsorship that consistently reinforces the value of evidence-based approaches and creates accountability for incorporating visual analytics into decision processes. Skill gaps among potential users can significantly limit adoption, particularly when visualization platforms require specialized knowledge to create or interpret complex visual representations. While AI has reduced some technical barriers, organizations still need comprehensive training programs that address both analytical thinking and tool-specific capabilities. Trust issues frequently emerge when AI-powered systems generate automated insights or recommendations without transparent explanations of their analytical methods. The most successful implementations address this challenge by incorporating explainable AI approaches that visualize not just conclusions but also the reasoning processes and data factors that led to those conclusions. Creating cross-functional centers of excellence that combine visualization expertise with domain knowledge helps bridge organizational silos and ensures that technical capabilities translate into business-relevant insights across different functional areas.
Future-proofing visualization investments requires thoughtful planning for evolving capabilities and changing business needs. Organizations should establish flexible architectural foundations that can accommodate emerging visualization techniques and data types without requiring complete platform replacements. Modular approaches that separate data management layers from visualization components allow for incremental updates as technologies mature or new requirements emerge. Establishing clear but adaptable standards for visualization design creates consistency across the organization while allowing for appropriate customization based on specific use cases or user needs. Regular evaluation of visualization effectiveness through user feedback and objective performance metrics helps identify opportunities for refinement or areas where new approaches might deliver greater value. Organizations that establish formal innovation processes for exploring emerging visualization capabilities report greater agility in responding to changing business conditions and analytical requirements. The most forward-thinking implementations incorporate continuous learning capabilities that enable visualization systems to evolve based on usage patterns and effectiveness metrics, creating self-improving platforms that increase in value over time rather than depreciating as static technological assets.
Conclusion
As we've explored throughout this article, AI-powered business intelligence visualization represents far more than an aesthetic upgrade to traditional analytics—it fundamentally transforms the relationship between organizations and their data. The evolution from static reports to intelligent, interactive visualizations has collapsed the distance between raw information and actionable insights, enabling decisions that are both faster and more informed across all organizational levels. The democratization of analytical capabilities through natural language interfaces and automated insight generation has extended the benefits of advanced visualization beyond technical specialists to frontline managers and executives who previously relied on secondhand interpretations of complex data patterns. This broader access to visual intelligence has accelerated organizational responsiveness, allowing companies to identify opportunities and address challenges with unprecedented speed and precision in an increasingly competitive global landscape.
The convergence of artificial intelligence and visualization technologies continues to push the boundaries of what's possible in business intelligence, with each new development expanding the horizons of insight discovery and decision support. Predictive visualizations have transformed business planning from a backward-looking exercise into a forward-focused exploration of potential futures, while real-time analytics dashboards have compressed response times from days to minutes for critical operational issues. Through advanced techniques like augmented analytics, cognitive visualization, and natural language processing, organizations are discovering patterns and relationships that would have remained hidden in traditional analysis approaches, unlocking new sources of value across diverse industry contexts. As implementation challenges are overcome through improved governance frameworks, technical architectures, and change management practices, these capabilities are becoming accessible to a broader range of organizations beyond early adopters.
Looking ahead, the future of business intelligence visualization promises even greater integration of human and artificial intelligence, creating symbiotic systems that leverage the unique strengths of each. As organizations build the necessary foundations—from data quality initiatives to visualization literacy programs—they position themselves to capitalize on emerging capabilities that will further transform how business insights are discovered, communicated, and applied. Those who successfully navigate this evolution will find themselves with a powerful competitive advantage: the ability to see patterns others miss, understand relationships others overlook, and act on opportunities others recognize too late. In a business environment increasingly defined by the ability to extract meaning from complexity, advanced visualization techniques powered by artificial intelligence have become not merely valuable tools but essential capabilities for sustainable success in the data-driven future of global commerce.
FAQ Section
What is AI-powered business intelligence visualization? AI-powered business intelligence visualization combines artificial intelligence algorithms with data visualization techniques to automatically identify patterns, generate insights, and create dynamic visual representations of complex business data that adapt to user needs and changing conditions. The technology leverages machine learning to enhance every aspect of the visualization workflow, from data preparation and pattern detection to presentation optimization and insight generation.
How does AI improve traditional data visualization? AI enhances traditional visualization by automatically identifying relevant patterns, suggesting optimal visualization types, processing larger datasets, enabling natural language interactions, creating predictive visualizations, and personalizing displays based on user preferences and behavior. These capabilities dramatically reduce the technical expertise required to generate insights while increasing the depth and relevance of visualizations for specific business contexts.
What industries benefit most from AI visualization techniques? Financial services, healthcare, retail, manufacturing, and telecommunications have seen the highest adoption and impact from AI visualization, with financial services leading at 78% adoption focusing on risk management and fraud detection applications. Healthcare organizations leverage visualization for patient outcome analysis, while retailers apply these techniques to understand complex customer journeys across digital and physical touchpoints.
What is augmented analytics in visualization? Augmented analytics uses machine learning to enhance human analysis throughout the visualization workflow, automatically preparing data, discovering patterns, recommending visualizations, generating insights, and continuously learning from user interactions to improve future recommendations. This approach dramatically accelerates the insight discovery process while reducing the specialized expertise required for complex data exploration.
How do interactive dashboards differ from traditional reports? Interactive dashboards differ from static reports by enabling dynamic data exploration, drill-down capabilities, cross-filtering between visualizations, real-time updates, and personalized views, creating an environment for discovery rather than just information consumption. Modern AI-enhanced dashboards also incorporate predictive elements and automated insight generation that extend beyond simple data representation.
What role does natural language processing play in visualization? NLP enables conversational interfaces where users can ask questions in plain language and receive relevant visualizations, generates automated explanations of visual patterns, and transforms unstructured text data into visual sentiment analysis and opinion mining. These capabilities dramatically lower the technical barriers to data exploration and make business intelligence accessible to broader audiences.
What are the biggest challenges in implementing AI-powered visualization? Major challenges include data quality issues, integration across disparate systems, technical infrastructure requirements, cultural resistance to data-driven approaches, skill gaps among users, and establishing trust in AI-generated insights. Organizations that proactively address these challenges through comprehensive implementation strategies report significantly higher satisfaction with their visualization investments.
How can organizations measure ROI from visualization investments? Organizations can measure ROI through metrics like decision-making speed (64% average improvement), operational efficiency (32% improvement), cost reduction (28% improvement), revenue growth (23% improvement), and user adoption rates of visualization tools. Successful implementations typically establish baseline measurements before deployment and track improvements across multiple performance indicators.
What skills are needed to implement AI visualization effectively? Successful implementation requires a combination of data science knowledge, visualization design principles, domain expertise, change management capabilities, and technical skills in data integration and platform architecture. Many organizations establish cross-functional centers of excellence that bring together these diverse capabilities to support enterprise-wide visualization initiatives.
What future trends are emerging in AI-powered visualization? Emerging trends include cognitive visualization that adapts to individual perception patterns, extended reality (AR/VR) for immersive data exploration, edge analytics visualization for IoT applications, and multimodal interfaces combining voice, touch, and gesture controls. These innovations will further expand the accessibility and impact of business intelligence across diverse user contexts and use cases.
Additional Resources
Business Intelligence Implementation Guide - A comprehensive resource covering technical architecture, data governance, and change management considerations for organizations implementing advanced visualization capabilities.
The Impact of Data Democratization - An in-depth analysis of how visualization accessibility affects organizational performance, with case studies from multiple industries.
Advanced Visualization Techniques Research - Original research examining the quantitative business impact of different visualization approaches across various operational contexts.
Real-Time Analytics Case Studies - Collection of implementation stories highlighting how organizations have successfully leveraged real-time visualization to transform operational performance.
Cognitive Visualization Research - Cutting-edge research on how visualization techniques can be optimized based on human perception principles to maximize comprehension and retention.