AI-Powered Fraud Detection in Insurance Claims: Transforming the Industry
Discover how cutting-edge AI technologies are revolutionising fraud detection in insurance claims processing, offering unprecedented accuracy, efficiency, and cost savings for insurers while reducing false positives and improving customer experience.
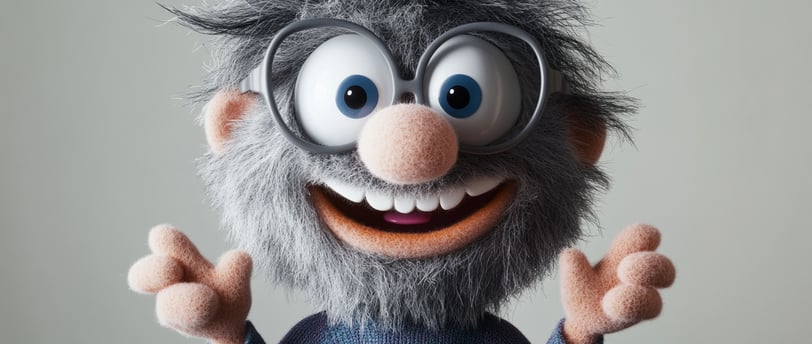
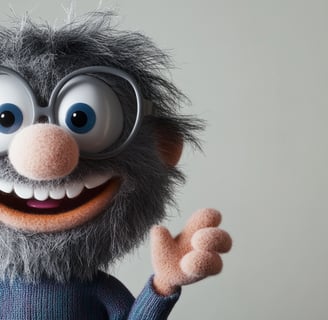
Insurance fraud costs the industry an estimated $40 billion annually in the United States alone, adding approximately $400-$700 to the average family's insurance premiums each year. Behind these staggering numbers lies a sophisticated battle between fraudsters developing increasingly complex schemes and insurers racing to identify and prevent these deceptive practices. The introduction of artificial intelligence has fundamentally altered this landscape, transforming fraud detection from a primarily manual, experience-based process into a data-driven, predictive science. This shift represents nothing short of a revolution in how insurance companies approach claim verification, investigation prioritization, and fraud prevention. In this comprehensive exploration, we'll examine how AI-powered methodologies are redefining insurance fraud detection, from machine learning algorithms that identify subtle patterns to natural language processing systems that flag suspicious narratives. We'll also investigate real-world implementation strategies, ethical considerations, and emerging technologies that promise to further advance this critical field.
The Growing Challenge of Insurance Fraud
Insurance fraud has evolved dramatically over the past decade, transforming from primarily opportunistic individual actions to sophisticated operations sometimes run by organized criminal networks. These operations often span multiple jurisdictions, utilize stolen or synthetic identities, and employ advanced techniques to circumvent traditional detection methods. The complexity of modern fraud schemes makes them particularly challenging to detect using conventional approaches, as fraudsters continuously adapt their tactics to exploit system vulnerabilities. Digital transformation has paradoxically both helped and hindered insurers, providing new data sources for verification while simultaneously creating new avenues for fraudulent activities. The COVID-19 pandemic further accelerated this trend, with the rapid shift to digital claims processing creating opportunities for fraudsters to exploit hastily implemented systems and processes.
The financial impact extends far beyond direct losses, encompassing investigation costs, litigation expenses, and damaged brand reputation. For health insurers alone, the National Health Care Anti-Fraud Association estimates that fraud represents between 3% and 10% of total healthcare spending, translating to tens of billions of dollars annually. Property and casualty insurers face similar challenges, with the Insurance Information Institute reporting that fraud comprises approximately 10% of property/casualty insurance losses and loss adjustment expenses each year. Auto insurance fraud remains particularly prevalent, with staged accidents, exaggerated injuries, and fictitious medical treatments constituting a significant portion of fraudulent claims.
These escalating challenges have forced insurers to reevaluate their approach to fraud detection, moving beyond rules-based systems toward more sophisticated, AI-driven methodologies. The insurance sector now recognizes that effective fraud detection requires not only identifying known patterns but also predicting and adapting to emerging fraud schemes before they become widespread. This realization has driven significant investment in advanced analytics and artificial intelligence technologies specifically designed for fraud detection and prevention.
Traditional Fraud Detection Methods and Their Limitations
Historically, insurance companies relied heavily on manual review processes conducted by experienced claims adjusters and special investigation units (SIUs). These professionals developed intuition for identifying suspicious patterns based on years of experience handling claims and investigating potential fraud. While valuable, this approach suffered from significant scalability limitations, subjective decision-making, and inconsistent application across different adjusters and regions. The knowledge remained largely tacit, difficult to transfer to new personnel, and challenging to apply systematically across high volumes of claims.
Rules-based systems represented the first major technological advancement in fraud detection, implementing explicit if-then logic to flag suspicious claims for further investigation. These systems codified known fraud indicators—such as claims filed shortly after policy inception, treatment dates falling on weekends or holidays, or round-dollar billing amounts—into automated screening protocols. While this approach improved consistency and scalability, it suffered from inherent limitations: rules could only detect known fraud patterns, required constant manual updating, and generated excessive false positives that overwhelmed investigation teams. Additionally, sophisticated fraudsters quickly learned to circumvent established rules by strategically designing claims to avoid triggering specific indicators.
Statistical models offered incremental improvement by analyzing historical claim data to identify correlations between claim characteristics and fraud outcomes. These models utilized techniques like regression analysis to assign fraud probability scores to incoming claims, enabling more efficient prioritization of investigative resources. However, traditional statistical approaches struggled with the complex, non-linear relationships often present in fraudulent activities and required significant domain expertise to develop and maintain. Furthermore, these models typically relied on structured data elements, missing valuable signals contained in unstructured data like adjuster notes, police reports, or medical documentation.
Another significant limitation of traditional methods was their reactive nature. Most conventional approaches detected fraud only after payment, requiring costly recovery efforts rather than preventing improper payments in the first place. This "pay and chase" model resulted in low recovery rates and additional administrative burden. The inability to adapt quickly to new fraud schemes created a perpetual lag between fraud innovation and detection capability, allowing significant losses before detection methods could be updated.
The AI Revolution in Fraud Detection
Artificial intelligence has fundamentally transformed insurance fraud detection by addressing the core limitations of traditional approaches. Unlike rules-based systems that rely on predetermined scenarios, AI methodologies can identify subtle patterns and anomalies without explicit programming, adapting continuously as fraud tactics evolve. This represents a paradigm shift from reactive to proactive detection, fundamentally changing how insurers approach this persistent challenge. The power of AI lies in its ability to process vast volumes of both structured and unstructured data, extracting insights from diverse sources that would be impossible for human analysts to synthesize manually.
Machine learning algorithms form the foundation of this revolution, learning from historical claims data to distinguish between legitimate and fraudulent patterns. These systems improve over time through continuous exposure to new data, becoming increasingly accurate at identifying suspicious claims while reducing false positives that create friction in the customer experience. Advanced ensemble models combining multiple algorithms often achieve the highest performance, leveraging the strengths of different approaches to address various fraud scenarios. Deep learning neural networks have proven particularly effective for complex pattern recognition in insurance data, identifying connections that would remain invisible to traditional analysis.
The transformative impact of AI extends beyond mere technical capability to fundamental business outcomes. Companies implementing AI-powered fraud detection systems typically report 30-50% increases in fraud detection rates, 60-80% reductions in false positives, and 40-60% improvements in investigative efficiency. These performance gains translate directly to bottom-line impact through reduced fraud losses, lower operational costs, and improved customer satisfaction. Furthermore, AI systems operate continuously, processing claims 24/7 without fatigue or variation in judgment quality that might affect human reviewers.
Perhaps most importantly, AI methodologies have shifted fraud detection from a cost center focused on loss recovery to a strategic capability that creates competitive advantage. Organizations leveraging advanced AI for fraud detection report not only financial benefits but also improved underwriting accuracy, enhanced customer segmentation, and more effective risk pricing. This integration of fraud detection with broader business intelligence capabilities represents a fundamental evolution in how insurers approach the fraud challenge, moving from isolated loss prevention to comprehensive risk management.
Key AI Technologies Transforming Fraud Detection
Several specific AI technologies have proven particularly transformative in the insurance fraud detection landscape. Supervised machine learning algorithms, including random forests, gradient boosting machines, and support vector machines, excel at classifying claims based on historical patterns where fraud outcomes are known. These algorithms analyze hundreds of variables simultaneously, identifying complex interactions and subtle indicators that might escape human notice. For example, a leading auto insurer implemented a gradient boosting model that identified non-obvious connections between repair facility locations, claimant demographics, and specific injury patterns, resulting in a 45% increase in fraud detection within six months of deployment.
Unsupervised machine learning approaches offer complementary capabilities by identifying anomalies without requiring labeled training data. These techniques excel at detecting emerging fraud schemes that differ from historical patterns, addressing one of the key limitations of traditional methods. Clustering algorithms group similar claims together, highlighting outliers that deviate from expected patterns in ways that may indicate fraudulent activity. Dimensionality reduction techniques like principal component analysis help visualize complex relationships within high-dimensional data, making patterns more apparent to fraud analysts. Together, these unsupervised approaches serve as an early warning system for novel fraud tactics.
Natural Language Processing (NLP) has revolutionized the analysis of unstructured text data, extracting valuable signals from claim descriptions, adjuster notes, medical reports, and other documentation. Advanced NLP models can identify inconsistencies in narratives, detect sentiment indicating potential deception, and extract key entities for cross-verification. Sentiment analysis can even assess the emotional tone of written communications, flagging unusual patterns that might indicate deception or coaching. Entity recognition capabilities automatically extract and validate information about people, places, and events mentioned in claim documentation, enabling automated cross-referencing against known data points.
Computer vision algorithms analyze images and videos associated with claims, automatically detecting signs of manipulation, inconsistencies with reported damage, or evidence of prior damage. These technologies can assess vehicle damage photographs, property loss documentation, or medical imaging to verify consistency with claimed injuries or damages. A major property insurer implemented a computer vision system that automatically detected discrepancies between reported hail damage and photographic evidence, reducing fraudulent claims by 28% in storm-affected regions. Some advanced systems can even analyze subtle metadata in digital images to identify signs of editing or manipulation that might indicate fraudulent documentation.
Network analysis techniques identify connections between claimants, medical providers, attorneys, and other parties across seemingly unrelated claims, revealing organized fraud rings that might otherwise remain undetected. By mapping relationships across thousands of claims, these systems can identify suspicious patterns of connections that indicate coordinated fraud activity. One health insurer uncovered a sophisticated fraud ring involving 23 providers, 198 claimants, and over $4.2 million in fraudulent claims by implementing network analysis technology that identified subtle connections between supposedly unrelated parties.
Implementation Case Studies
The theoretical promise of AI-powered fraud detection becomes tangible through real-world implementation examples. A leading European auto insurer deployed a comprehensive AI fraud detection system that reduced fraudulent claim payments by €25 million in the first year while simultaneously improving customer satisfaction scores for legitimate claimants. The system combined predictive models analyzing over 200 variables with natural language processing of claim descriptions and network analysis to identify potential fraud rings. Claims identified as potentially fraudulent received additional verification steps, while low-risk claims proceeded through an expedited process, benefiting honest customers.
In the health insurance sector, a major U.S. payer implemented an AI system focused on provider fraud detection, analyzing billing patterns, procedure codes, and provider networks to identify suspicious activities. The system flagged unusual billing patterns, inappropriate coding, and statistical outliers compared to peer providers in similar specialties and regions. Within eight months, the system identified three previously undetected provider fraud schemes involving coordinated billing for unnecessary services, resulting in recoveries exceeding $12 million and prevention of approximately $30 million in additional fraudulent payments.
Property insurance has similarly benefited from AI implementation, with a global insurer deploying a system that analyzes weather data, property characteristics, and claim details to identify potentially fraudulent storm damage claims. The system automatically cross-references reported damage with verified weather events and typical damage patterns, flagging inconsistencies for further investigation. This targeted approach increased special investigation unit effectiveness by 65% while reducing the number of legitimate claimants subjected to unnecessary investigation by 78%, improving both operational efficiency and customer experience.
Workers' compensation represents another area where AI has demonstrated significant impact. A regional workers' compensation insurer implemented a multi-faceted AI system that analyzes claim documentation, medical records, and claimant behavior patterns to identify potentially fraudulent claims. The system automatically detects inconsistencies between reported injuries and treatment patterns, identifies unusual provider billing practices, and flags claims with characteristics similar to previously confirmed fraud cases. Since implementation, the insurer has reduced fraudulent claim payments by 32% while shortening processing time for legitimate claims by an average of 4.3 days.
These case studies highlight a critical implementation lesson: successful AI fraud detection deployments typically adopt a hybrid approach combining multiple AI technologies with human expertise rather than relying solely on automation. The most effective systems leverage AI to augment human judgment rather than replace it entirely, using technology to identify potential issues while relying on trained investigators to make final determinations. This balanced approach maximizes detection capabilities while maintaining appropriate human oversight and judgment.
Ethical and Privacy Considerations
The increasing power of AI-based fraud detection brings significant ethical considerations that insurers must carefully navigate. Algorithmic bias represents one of the most pressing concerns, as models trained on historical data may inadvertently perpetuate or amplify existing biases in the fraud investigation process. If certain demographic groups have historically been investigated at higher rates, an AI system might learn to flag similar groups at disproportionate rates, potentially creating discriminatory outcomes. Leading organizations address this risk through regular bias audits, diverse training data, and explicit testing for disparate impact across protected characteristics. Regulatory frameworks increasingly require such testing, with several jurisdictions implementing specific requirements for algorithmic fairness in insurance applications.
Privacy considerations also feature prominently in AI fraud detection implementations, particularly as systems increasingly incorporate external data sources and advanced analytics capabilities. The tension between comprehensive fraud detection and appropriate data use requires careful balancing, with explicit policies regarding data collection, retention, and purpose limitation. Many organizations implement privacy-by-design principles in their AI systems, incorporating data minimization, anonymization techniques, and strict access controls. Federated learning approaches have emerged as a promising solution, enabling models to learn from distributed data sources without centralizing sensitive information. This approach allows insurers to benefit from broad pattern recognition while maintaining stronger privacy protections.
The "black box" nature of certain AI algorithms presents another significant ethical challenge, particularly when decisions affect customer outcomes or trigger fraud investigations. Insurance regulators increasingly require explainability for AI models used in consequential decisions, necessitating approaches that balance performance with interpretability. Leading organizations implement interpretable AI frameworks that provide human-understandable explanations for model decisions, enabling appropriate oversight and contestability. These frameworks typically include visualization tools that help investigators understand key factors driving fraud suspicion, supporting human judgment rather than replacing it entirely.
Perhaps most fundamentally, organizations must determine the appropriate balance between automation and human judgment in fraud determination. While AI excels at identifying patterns and prioritizing cases, most insurers maintain human oversight for final determinations, particularly for complex or high-value cases. This hybrid approach recognizes both the unique capabilities of AI systems and their limitations, ensuring appropriate checks on automated decision processes. Human experts typically review model outputs, investigate flagged claims, and make ultimate decisions about fraud designation and appropriate action. This collaborative approach leverages the strengths of both technology and human expertise while mitigating the risks of over-reliance on either component.
Measuring ROI and Performance Metrics
Quantifying the return on investment for AI-powered fraud detection systems requires a comprehensive measurement framework that captures both direct and indirect benefits. Direct fraud savings represent the most visible impact, typically measured through prevented fraudulent payments and recovered improper payments identified through AI-enhanced detection. These metrics should be compared to historical baseline performance using traditional methods to isolate the incremental impact of AI implementation. Organizations typically report 30-50% increases in fraud identification within the first year of implementation, with continuous improvement as systems learn from new data over time.
Operational efficiency gains constitute another significant ROI component, encompassing reduced manual review requirements, improved investigator productivity, and faster claim processing timelines. Leading organizations track metrics like investigator caseload capacity, time-to-resolution for investigations, and special investigation unit productivity to quantify these benefits. The most sophisticated measurement approaches incorporate time studies comparing pre-implementation and post-implementation workflows to quantify labor savings and capacity enhancements. One major insurer reported that investigators could handle 3.5 times more cases after AI implementation by focusing primarily on claims with strong fraud indicators rather than performing extensive manual screening.
Customer experience impact represents an often-undervalued benefit of effective AI fraud detection. By reducing false positives and enabling straight-through processing for legitimate claims, these systems can significantly improve cycle times and satisfaction for honest customers. Organizations should track metrics like claim processing time for legitimate claims, customer satisfaction scores, and retention rates to quantify these benefits. Several studies have demonstrated a correlation between improved fraud detection capabilities and higher customer satisfaction, primarily through faster processing for the majority of legitimate claims.
A comprehensive ROI assessment should also include cost factors such as technology investment, implementation expenses, ongoing maintenance, and operational changes. These costs vary significantly based on implementation approach, with cloud-based solutions typically requiring lower upfront investment but higher ongoing expenses compared to on-premise deployments. Integration complexity with existing claims systems represents another significant cost driver, particularly for organizations with legacy technology environments. Despite these costs, most insurers report positive ROI within 12-18 months of implementation, with payback periods continuing to decrease as AI technologies mature and implementation best practices become more established.
Challenges and Limitations
Despite significant advances, AI-powered fraud detection systems face persistent challenges that limit their effectiveness in certain scenarios. Data quality issues represent one of the most common obstacles, as inconsistent, incomplete, or inaccurate data can significantly degrade model performance. The insurance industry's legacy of siloed systems often creates particular challenges for data integration, requiring significant preprocessing and normalization before advanced analytics can be effectively applied. Organizations must invest in robust data governance frameworks, quality monitoring processes, and integration capabilities to address these foundational issues before AI implementation can achieve optimal results.
Talent acquisition and retention represent another significant challenge, particularly for organizations implementing sophisticated AI systems requiring specialized expertise. The competitive market for data scientists, machine learning engineers, and AI specialists makes building and maintaining these capabilities difficult for many insurers. This challenge is particularly acute for mid-sized and smaller organizations competing against both large insurers and technology companies for limited talent. Successful organizations typically adopt multi-faceted talent strategies combining hiring, partnering with specialized vendors, and upskilling existing technical personnel to build sustainable capabilities.
Regulatory compliance adds another layer of complexity to AI implementation, with requirements varying significantly across jurisdictions and continuing to evolve as oversight frameworks adapt to new technologies. Requirements for model transparency, explainability, data protection, and fair treatment create implementation challenges, particularly for global insurers operating across multiple regulatory environments. These requirements sometimes create tension with performance objectives, as the most explainable models may not always deliver the highest detection accuracy. Organizations must implement robust model governance frameworks, documentation practices, and testing protocols to address these regulatory considerations while still achieving performance objectives.
Change management represents perhaps the most underestimated challenge, as effective implementation requires significant workflow and process changes across claims processing, special investigation units, and related functions. Resistance to new tools and processes can significantly impact adoption and effectiveness, particularly when systems are perceived as threatening investigator autonomy or expertise. Successful implementations typically involve extensive stakeholder engagement, targeted training programs, and carefully designed integration with existing workflows to build trust and adoption. Organizations that treat AI implementation as a change management initiative rather than merely a technology deployment typically achieve significantly better outcomes and sustainable performance improvement.
Future Trends and Developments
The future of AI-powered fraud detection promises even greater capabilities through several emerging technologies and approaches. Real-time fraud detection represents one of the most significant frontiers, moving beyond post-submission analysis to identify potential fraud indicators during initial quote and application processes. These capabilities enable insurers to detect fraud before policies are issued or claims are paid, fundamentally shifting from recovery to prevention. Advanced systems incorporate predictive risk scoring at each customer touchpoint, from initial website visit through policy issuance and claim submission, creating a continuous fraud prevention ecosystem rather than isolated point solutions.
Federated learning approaches are gaining traction as a solution to data privacy constraints, enabling models to learn from distributed data sources without centralizing sensitive information. This approach allows insurers to benefit from industry-wide patterns without compromising customer privacy or competitive information. Several industry consortiums are developing federated learning platforms that enable cross-carrier fraud detection while maintaining appropriate data separation and protection. These collaborative approaches show particular promise for identifying organized fraud rings that operate across multiple insurers to avoid detection through activity dispersion.
Explainable AI models represent another crucial development area, as both regulatory requirements and practical considerations drive demand for interpretable fraud detection systems. Next-generation approaches focus on inherently interpretable models rather than post-hoc explanations, enabling both regulatory compliance and more effective investigator collaboration. These models provide transparent reasoning for fraud suspicions, helping investigators understand not just what triggered an alert but why specific patterns indicate potential fraud. This explainability enhances both regulatory compliance and operational effectiveness by building investigator trust and enabling more targeted follow-up activities.
Integration with emerging data sources continues to expand detection capabilities, with social media analysis, IoT device data, and publicly available information enhancing verification capabilities. Advanced computer vision systems now analyze repair shop videos, telematics data from connected vehicles provides objective accident information, and property sensors offer real-time environmental monitoring for disaster claims. These expanded data sources enable new verification approaches that were previously impossible, fundamentally changing the information asymmetry that has historically favored fraudsters. As these capabilities mature, we can expect increasingly robust, multi-dimensional fraud detection ecosystems that combine diverse data sources with specialized AI technologies for each fraud scenario.
Conclusion
AI-powered fraud detection represents a transformative force in the insurance industry, fundamentally changing how companies approach this persistent challenge. The evolution from rules-based systems to sophisticated AI methodologies has enabled unprecedented detection capabilities, operational efficiencies, and customer experience improvements. Organizations implementing these technologies report substantial increases in fraud identification, dramatic reductions in false positives, and significant ROI, establishing AI as an essential component of modern insurance operations rather than merely an experimental technology. The impact extends beyond direct fraud savings to fundamental business capabilities, creating competitive advantage through improved risk assessment, operational efficiency, and customer experience.
As these technologies continue to mature, we can expect further evolution toward real-time prevention, enhanced collaboration across the industry, and increasingly sophisticated detection capabilities leveraging diverse data sources and advanced AI techniques. These advancements will continue to shift the advantage away from fraudsters toward insurers and honest policyholders who ultimately bear the cost of fraud through higher premiums. However, realizing these benefits requires thoughtful implementation that addresses data quality challenges, talent constraints, ethical considerations, and change management requirements. Organizations that approach AI implementation as a comprehensive business transformation rather than merely a technology deployment will achieve the greatest success.
The future of insurance fraud detection will likely be characterized by increasingly sophisticated hybrid approaches combining specialized AI technologies with human expertise in a collaborative ecosystem. This balanced approach leverages the unique strengths of both components—AI's ability to process vast data volumes and identify subtle patterns alongside human judgment, contextual understanding, and ethical decision-making. As these capabilities continue to advance, the insurance industry stands to benefit not only from reduced fraud losses but also from fundamentally improved risk assessment, customer experience, and operational efficiency. The AI revolution in fraud detection represents not merely an incremental improvement but a fundamental transformation of how insurers protect themselves and their customers from the persistent challenge of fraud.
Frequently Asked Questions
How much does insurance fraud cost the industry annually?
Insurance fraud costs the industry an estimated $80-$90 billion annually in the United States alone, with global estimates exceeding $250 billion. This translates to increased premiums for all policyholders, with the average U.S. family paying an additional $400-$700 per year due to fraud.
What is the difference between soft fraud and hard fraud in insurance?
Soft fraud involves exaggerating legitimate claims (like inflating values of stolen items), while hard fraud refers to deliberately fabricated claims or staged incidents. Soft fraud is more common but harder to detect, while hard fraud is less frequent but typically involves larger dollar amounts.
How does AI improve fraud detection compared to traditional methods?
AI significantly improves fraud detection by analyzing vast amounts of structured and unstructured data simultaneously, identifying subtle patterns human analysts might miss, adapting to new fraud schemes in real-time, reducing false positives by 60-80%, and processing claims 24/7 without fatigue or judgment variation.
What types of insurance are most vulnerable to fraud?
Health insurance experiences the highest dollar value of fraud ($68+ billion annually), while auto insurance has the highest percentage of fraudulent claims (13-17%). Workers' compensation, property insurance, and disability insurance also experience significant fraud rates, each with unique vulnerability patterns.
What role does Natural Language Processing play in fraud detection?
Natural Language Processing (NLP) analyzes unstructured text in claims descriptions, adjuster notes, and medical reports to identify inconsistencies, deceptive language patterns, and suspicious narratives. NLP can extract key entities for verification and detect sentiment indicating potential fraud that might be missed in structured data analysis.
How do insurance companies measure ROI from AI fraud detection implementations?
Insurers measure AI ROI through direct fraud savings (prevented + recovered fraudulent payments), operational efficiency gains (investigator productivity and reduced manual reviews), improved customer experience metrics (faster processing times for legitimate claims), and reduced false positive rates that minimize unnecessary investigations.
What ethical concerns exist around AI-powered fraud detection?
Key ethical concerns include algorithmic bias potentially affecting certain demographic groups unfairly, privacy implications of extensive data collection and analysis, explainability challenges with 'black box' AI models, and the appropriate balance between automation and human judgment in final fraud determination decisions.
How are organized fraud rings detected using AI?
AI detects organized fraud rings through network analysis algorithms that identify connections between supposedly unrelated claims by mapping relationships between claimants, providers, addresses, phone numbers, and other entities. These systems can uncover subtle patterns of connection across thousands of claims that would be impossible to detect manually.
What challenges do insurers face when implementing AI fraud detection?
Major implementation challenges include data quality and integration issues across legacy systems, talent acquisition for specialized AI roles, resistance to workflow changes, complex regulatory compliance requirements, model explainability for audit purposes, and building trust in AI-driven decisions among claims handlers and investigators.
What future trends are emerging in AI-powered fraud detection?
Emerging trends include real-time fraud detection at point of quotation, federated learning for cross-carrier fraud prevention without sharing sensitive data, explainable AI models for regulatory compliance, IoT device integration for verification, blockchain for immutable claim histories, and behavioral biometrics to authenticate legitimate claimants.
Additional Resources
Coalition Against Insurance Fraud - "The State of Insurance Fraud Technology" (2023) - Comprehensive industry study examining adoption rates, implementation approaches, and performance metrics for fraud detection technologies across North American insurers.
McKinsey & Company - "AI in Insurance: Harnessing AI for Fraud Detection and Prevention" (2022) - Detailed analysis of implementation strategies, organizational considerations, and performance benchmarks for AI fraud detection across global insurers.
Insurance Information Institute - "Insurance Fraud: Trends, Challenges and Solutions" (2023) - Industry whitepaper examining the evolving fraud landscape and emerging detection methodologies with case studies from leading implementations.
SAS Institute - "Machine Learning for Insurance Fraud Detection: From Detection to Prevention" (2024) - Technical whitepaper exploring specific algorithms, implementation approaches, and performance metrics with anonymized case studies.
Journal of Insurance Fraud Analytics - "Ethical Considerations in AI-Powered Fraud Detection" (2023) - Academic paper examining regulatory requirements, fairness considerations, and governance frameworks for responsible AI use in insurance applications.