AI-Powered Inventory Optimisation
Discover how AI-driven inventory optimisation transforms supply chains by simultaneously reducing inventory costs by up to 30% while improving product availability by 15-20%. Learn implementation strategies, ROI measurement, and future trends in intelligent inventory management.
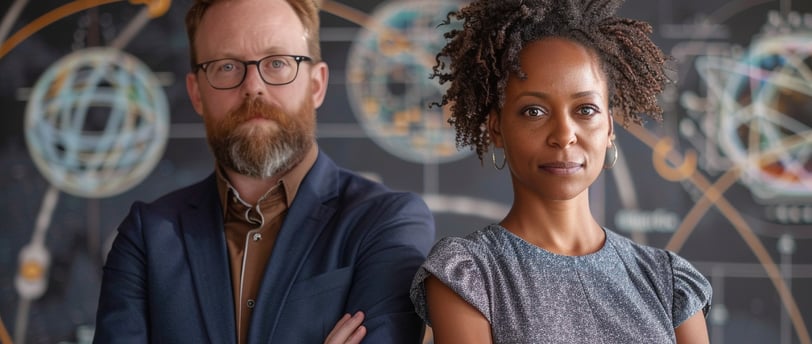
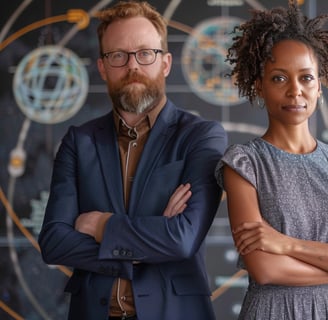
In today's business landscape, the age-old inventory dilemma continues to challenge organizations of all sizes: how to minimize costly excess inventory while ensuring products remain available when customers want them. This fundamental tension between cost control and customer satisfaction has traditionally forced businesses into making uncomfortable compromises, often resulting in either bloated warehouses or disappointed customers. However, artificial intelligence is now revolutionizing this critical aspect of operations by elegantly solving both sides of the equation simultaneously. AI-powered inventory optimization systems are transforming the way businesses manage their supply chains, creating unprecedented efficiencies while maintaining or even improving service levels. By analyzing vast amounts of data, identifying patterns invisible to human analysts, and generating predictive insights, these intelligent systems are helping companies dramatically reduce inventory costs while simultaneously enhancing product availability. This article explores the transformative potential of AI in inventory management, examining the technologies, implementation strategies, measurable benefits, and real-world applications that are reshaping this critical business function.
The Current Challenges in Inventory Management
Inventory management has long been one of the most challenging aspects of business operations, representing a delicate balancing act between competing priorities. Traditional inventory management approaches often rely heavily on historical averages, basic forecasting models, and the intuition of experienced staff—methods that struggle to account for today's complex market dynamics. Many organizations continue to experience significant inefficiencies, with global studies indicating that retailers alone hold approximately $1.43 trillion in inventory, of which nearly $472 billion represents overstock that ties up valuable capital and warehouse space. On the opposite end of the spectrum, stockouts cost retailers an estimated $984 billion annually in lost sales, highlighting the severe consequences of insufficient inventory levels. Modern supply chains face unprecedented volatility from factors such as changing consumer preferences, seasonal fluctuations, supplier disruptions, and global economic uncertainties—variables that conventional systems struggle to model effectively. Additionally, the explosion of SKUs in many industries has exponentially increased complexity, with some retailers managing hundreds of thousands of unique items across multiple locations, each with distinct demand patterns and lead times.
The fundamental problem lies in the limitations of traditional forecasting methods that typically rely on historical data without adequately capturing causal factors or complex interdependencies. Human planners, no matter how experienced, simply cannot process the volume and complexity of data required to optimize inventory decisions across thousands of products and multiple locations simultaneously. Furthermore, the lack of visibility across supply chain tiers creates information silos that further complicate accurate planning and response capabilities. The financial implications of these challenges are substantial, with excess inventory tying up working capital, increasing storage costs, and risking obsolescence, while insufficient stock levels lead to lost sales, reduced customer satisfaction, and potential long-term damage to brand reputation. Even minor improvements in these areas can translate to millions in savings and revenue gains for mid-sized and large organizations.
The transition to e-commerce and omnichannel retail has further complicated inventory management, requiring businesses to fulfill orders from multiple channels while maintaining consistent service levels. Customer expectations have simultaneously increased, with next-day or same-day delivery becoming the norm rather than a competitive advantage in many markets. Seasonal variations and promotional activities introduce additional complexity, creating demand spikes that are difficult to predict and manage without sophisticated analytical capabilities. Supply chain disruptions, from weather events to global pandemics, have demonstrated the fragility of existing inventory systems and the need for more resilient approaches that can adapt to unexpected changes. These combined pressures have pushed traditional inventory management methods to their breaking point, creating an urgent need for more intelligent, responsive systems that can navigate complexity while balancing competing objectives.
Understanding AI-Powered Inventory Optimization
AI-powered inventory optimization represents a fundamental shift from reactive to proactive inventory management through advanced analytical capabilities. At its core, artificial intelligence brings the power to process massive datasets, identify complex patterns, and generate actionable insights far beyond what traditional systems or human analysts could achieve. Unlike conventional approaches that typically rely on historical averages and basic statistical methods, AI systems incorporate a vastly broader range of variables and can detect subtle correlations that impact inventory requirements. These systems employ various AI and machine learning techniques, including deep learning neural networks, regression models, classification algorithms, and time series analysis to develop highly accurate demand forecasts and inventory recommendations. The technology continuously learns from new data, improving its accuracy over time and adapting to changing market conditions without requiring constant manual recalibration.
Modern AI inventory systems integrate multiple data sources to create a comprehensive view of factors affecting inventory needs. These data streams might include historical sales data, point-of-sale transactions, website traffic, social media trends, weather forecasts, economic indicators, competitor pricing, and even news events that could impact consumer behavior or supply chain operations. By synthesizing these diverse inputs, AI creates multidimensional models that capture complex relationships between variables and their impact on demand patterns. This holistic approach enables the system to distinguish between correlation and causation, identifying the true drivers of demand fluctuations rather than simply projecting historical trends forward. The result is significantly improved forecast accuracy—often showing 20-30% improvement over traditional methods—which directly translates to more precise inventory positioning.
The optimization capabilities extend beyond simply forecasting demand to making nuanced inventory decisions based on business objectives. AI systems can simultaneously balance multiple competing factors including carrying costs, stockout risks, service level agreements, lead times, shelf life constraints, and storage limitations to determine optimal order quantities and timing. These systems typically employ sophisticated optimization algorithms that consider thousands of possible scenarios to identify the most efficient inventory strategy given current constraints and business priorities. Rather than using static reorder points or economic order quantities, AI continuously recalculates optimal inventory levels as conditions change, allowing for dynamic adjustments that maximize efficiency. The technology also enables probabilistic planning that accounts for uncertainty, moving beyond single-point forecasts to understand the range of possible outcomes and make inventory decisions that appropriately hedge against risks.
AI inventory optimization operates across multiple time horizons simultaneously, from immediate operational decisions to medium-term tactical planning and long-term strategic insights. At the operational level, the system might adjust daily order quantities based on real-time sales data and inventory positions. Tactically, it might identify seasonal patterns and recommend inventory build-up strategies for upcoming peak periods. Strategically, AI can uncover long-term trends and structural changes in demand patterns that inform facility planning, supplier relationships, and product portfolio decisions. This multi-layered approach ensures that day-to-day efficiency doesn't come at the expense of long-term preparedness, creating a balanced inventory strategy that remains resilient across various market conditions while continuously optimizing for cost and availability objectives.
Key Benefits: Cost Reduction and Improved Availability
The dual benefits of cost reduction and improved product availability represent the most compelling business case for AI-powered inventory optimization. Traditional inventory management has typically forced companies to choose between these competing objectives, but AI's sophisticated analytical capabilities enable organizations to achieve both simultaneously. On the cost reduction side, companies implementing AI inventory systems report average inventory reductions of 15-30% while maintaining or improving service levels. This translates directly to working capital improvements, with organizations freeing up millions in previously idle inventory investments. A consumer goods manufacturer with $500 million in inventory, for example, might release $75-150 million in working capital through more precise inventory positioning. Beyond the balance sheet impact, reduced inventory levels create operational savings through decreased warehouse space requirements, lower insurance costs, reduced handling expenses, and fewer write-offs for obsolete or expired products.
Improved product availability delivers equally significant benefits through enhanced customer satisfaction and increased sales. AI-driven systems typically improve in-stock rates by 5-15 percentage points, directly addressing the estimated $984 billion in annual lost sales from stockouts. This availability improvement drives revenue through both immediate sales capture and long-term customer loyalty enhancements. Research indicates that customers experiencing stockouts will often substitute a competitor's product and may permanently switch if stockouts occur repeatedly, making availability a critical factor in customer retention. The precise demand forecasting capabilities of AI systems enable businesses to not only maintain appropriate stock levels for existing products but also better plan for new product introductions, promotions, and seasonal fluctuations that traditionally create inventory management challenges. By anticipating demand spikes with greater accuracy, businesses can ensure product availability during critical selling periods without excessive safety stock investments.
The financial impact of these combined benefits creates compelling ROI cases for AI inventory systems. A mid-sized retailer might see annual improvements of $5-10 million through a combination of reduced carrying costs, decreased obsolescence, and increased sales from better availability. Larger enterprises with global operations often report nine-figure annual impacts from comprehensive AI inventory optimization initiatives. Beyond these direct financial benefits, organizations gain substantial competitive advantages through improved customer satisfaction, faster response times to market changes, and more efficient use of working capital. The improved inventory turns also create virtuous cycles of freshness for products with shelf-life constraints, reducing waste and improving customer experiences with consistently fresher products. Additionally, better inventory management reduces the environmental impact of excess production and waste, supporting sustainability initiatives that increasingly influence consumer purchasing decisions.
For organizations with complex, multi-echelon supply chains, AI optimization delivers particularly significant advantages through global inventory optimization. Rather than optimizing each node independently, AI systems can model the entire supply network to identify the optimal distribution of inventory across locations. This network-wide perspective prevents the common problem of inventory imbalances, where some locations experience stockouts while others hold excess inventory of the same products. By dynamically shifting inventory based on real-time demand signals and transportation constraints, AI enables true inventory pooling across the network. This approach typically reduces total system-wide inventory requirements by an additional 10-20% compared to location-by-location optimization approaches, while simultaneously improving product availability through more responsive inventory positioning. The ability to optimize across locations also enhances resilience against disruptions, allowing the system to rebalance inventory when supply or demand shocks affect specific regions.
AI Technologies Transforming Inventory Management
The AI revolution in inventory management leverages multiple technologies working in concert to create intelligent, adaptive systems that far surpass traditional approaches. Machine learning algorithms form the foundation of modern inventory optimization, with various approaches addressing different aspects of the inventory challenge. Regression models analyze relationships between variables to understand how factors like pricing, promotions, seasonality, and external events influence demand patterns. Time series analysis methods, including ARIMA (AutoRegressive Integrated Moving Average) and Prophet models, capture temporal patterns in historical data to project future trends while accounting for seasonality and growth factors. More advanced deep learning approaches, such as recurrent neural networks (RNNs) and Long Short-Term Memory (LSTM) networks, excel at identifying complex patterns in sequential data, making them particularly effective for demand forecasting with multiple influencing variables.
Natural Language Processing (NLP) extends the capabilities of inventory optimization systems by incorporating unstructured data sources that traditionally remained untapped. By analyzing news articles, social media conversations, customer reviews, and other text-based information, NLP algorithms can identify emerging trends, consumer sentiment shifts, and potential supply chain disruptions before they impact traditional metrics. This early warning capability enables proactive inventory adjustments rather than reactive responses to already-changing conditions. For example, an NLP system might detect growing social media buzz about a product, indicating potential demand increases weeks before sales data would show the trend. Similarly, it might identify negative news about a supplier or region that could signal upcoming supply chain disruptions, allowing for preemptive inventory adjustments to mitigate risks. This capability to incorporate qualitative information alongside quantitative data provides a much more comprehensive view of factors affecting inventory requirements.
Computer vision technology is creating new possibilities for real-time inventory tracking and management, particularly in retail environments. Advanced image recognition systems can automatically track shelf inventory through either fixed cameras or mobile robots that patrol store aisles, identifying products, counting stock levels, and detecting misplaced items with remarkable accuracy. These systems create near-real-time visibility into actual store conditions, enabling much more responsive replenishment processes than traditional methods that rely on point-of-sale data alone. The same technology extends to warehouse operations, where computer vision systems monitor storage locations, verify picking accuracy, and optimize space utilization. By maintaining continuous awareness of actual inventory positions rather than system estimates, these technologies eliminate the discrepancies that traditionally plague inventory management and lead to either excess safety stock or unexpected stockouts.
Reinforcement learning represents perhaps the most sophisticated application of AI in inventory optimization. Unlike supervised learning approaches that require labeled historical data, reinforcement learning systems learn optimal inventory policies through simulated environments where they can explore different strategies and discover optimal approaches through trial and error. The system receives rewards for desired outcomes (maintaining stock while minimizing costs) and penalties for undesired results (stockouts or excessive inventory), gradually refining its approach to maximize long-term performance. This technique is particularly valuable for complex inventory scenarios with multiple constraints and competing objectives, as it can discover non-obvious strategies that human planners might never consider. Reinforcement learning also excels at balancing exploration (trying new approaches) with exploitation (leveraging known successful strategies), creating systems that continuously adapt to changing conditions while maintaining reliable performance.
The integration of these AI technologies with traditional inventory management systems creates intelligent platforms that combine advanced analytical capabilities with practical operational execution. Modern systems typically feature intuitive dashboards that provide visibility into current inventory positions, forecast accuracy, potential risks, and recommended actions. These interfaces translate complex AI insights into actionable information for human planners, who retain oversight of the system while benefiting from its analytical power. The most effective implementations combine AI recommendations with human judgment, allowing experienced staff to apply contextual knowledge and business priorities while leveraging the system's computational abilities. This human-machine collaboration creates inventory management capabilities far beyond what either could achieve independently, enabling organizations to navigate the increasing complexity of modern supply chains while optimizing for both cost efficiency and customer satisfaction.
Implementation Strategies for AI Inventory Systems
Successful implementation of AI-powered inventory optimization requires a strategic approach that addresses technical, organizational, and process considerations. The journey typically begins with a thorough assessment of current inventory management practices, data availability, system capabilities, and business objectives. This diagnostic phase identifies specific pain points, quantifies the potential value of improvements, and establishes clear success metrics aligned with strategic priorities. Organizations should resist the temptation to pursue a "big bang" implementation, instead adopting a phased approach that delivers incremental value while building organizational capabilities and confidence. A common strategy starts with a limited pilot focused on a specific product category, business unit, or geographic region where the potential impact is significant and measurable. This approach allows the organization to demonstrate value quickly while refining the implementation methodology before broader deployment.
Data quality and availability represent fundamental requirements for AI inventory systems, as even the most sophisticated algorithms cannot compensate for incomplete or inaccurate inputs. Organizations must take a comprehensive approach to data management, identifying all relevant data sources and establishing processes to ensure data accuracy, completeness, and timeliness. Critical data elements typically include transaction history (ideally at the most granular level available), promotional calendars, pricing changes, competitor activities, supply chain parameters (lead times, minimum order quantities, etc.), and external factors that influence demand patterns. Integration capabilities are equally important, as the system must be able to receive inputs from and send outputs to various operational systems including ERP platforms, warehouse management systems, and e-commerce platforms. Cloud-based architectures often provide the most flexible foundation for these integrations, enabling real-time data flows while minimizing infrastructure requirements.
Change management deserves particular attention during implementation, as AI inventory systems often represent a significant shift in how inventory decisions are made. Organizations must address both technical and cultural aspects of this transition to ensure successful adoption. The role of inventory planners and managers evolves from making routine stocking decisions to managing exceptions, analyzing system recommendations, and contributing domain expertise to continuous improvement efforts. This transition requires thoughtful communication about how AI augments rather than replaces human capabilities, along with comprehensive training programs that build both technical skills and confidence in the new approach. Executive sponsorship proves crucial for navigating organizational resistance and ensuring alignment across functions that impact or are impacted by inventory decisions, including sales, marketing, finance, and operations. The most successful implementations position AI inventory optimization as a strategic initiative rather than a purely technical project, ensuring it receives appropriate resources and attention.
Implementation timelines and resource requirements vary significantly based on organizational size, complexity, and existing technical capabilities. A mid-sized company with relatively straightforward inventory challenges and good data availability might implement a basic AI optimization system within 3-6 months. More complex environments with multiple distribution tiers, thousands of SKUs, and numerous sales channels typically require 9-18 months for full implementation, often with a phased approach delivering incremental benefits throughout the process. Resource requirements include data scientists and engineers to configure and train the AI models, integration specialists to connect with existing systems, business analysts to translate between technical and operational teams, and training resources to support user adoption. Many organizations partner with specialized vendors or consultants to supplement internal capabilities, particularly for the initial implementation, while building internal expertise for ongoing management and optimization.
Post-implementation, continuous improvement processes ensure the system evolves with changing business needs and market conditions. Regular performance reviews should compare actual results against the initial success metrics, identifying areas for refinement and expansion. As users gain experience with the system, they often identify new use cases and opportunities for extending the capabilities to address additional business challenges. Organizations should establish formal feedback loops between operational teams and technical specialists to capture these insights and prioritize enhancements. The AI models themselves require ongoing monitoring to ensure they maintain accuracy as conditions change, with regular retraining as necessary to incorporate new data patterns. This lifecycle management approach treats the AI inventory system as a continuously evolving asset rather than a one-time implementation, maximizing long-term value while adapting to emerging business priorities and market dynamics.
Real-World Success Stories and Case Studies
The transformative potential of AI-powered inventory optimization is perhaps best illustrated through real-world implementations across various industries. In the retail sector, a leading global apparel company implemented an AI-driven inventory management system across its 3,000+ stores and e-commerce operations. The retailer had historically struggled with significant inventory imbalances, maintaining over $2 billion in total inventory while still experiencing stockouts on popular items and accumulating excess stock of slower-moving products. After implementing an AI optimization system that incorporated weather patterns, social media trends, and local events alongside traditional sales data, the company reduced overall inventory by 22% while simultaneously improving in-stock rates by 9 percentage points. This dual improvement released approximately $440 million in working capital while increasing annual sales by an estimated $370 million through better product availability. The system proved particularly valuable for managing seasonal transitions, reducing markdown rates by identifying optimal timing for inventory reductions ahead of season changes.
A pharmaceutical distributor serving hospitals and pharmacies nationwide provides another compelling example of AI optimization benefits. The company managed over 25,000 SKUs across 12 distribution centers, balancing the critical need for product availability with the challenges of limited shelf life and high carrying costs for specialized medications. Their AI implementation focused on multi-echelon inventory optimization, creating a network-wide view that enabled more effective inventory positioning and transfer policies between distribution centers. The results included a 31% reduction in inventory for non-critical medications while maintaining 99.8% availability for life-saving drugs. The system's ability to predict demand surges for seasonal medications (like flu treatments) and respond to regional disease outbreaks significantly improved service levels during critical periods. The company also reported substantial reductions in expired product write-offs, with the AI system's precise forecasting reducing waste by identifying optimal order quantities that balanced availability with shelf-life constraints.
In manufacturing, a global automotive parts supplier implemented AI inventory optimization across its complex supply chain spanning 75 manufacturing facilities and over 100,000 SKUs. The company faced significant challenges with long and variable lead times from global suppliers, fluctuating raw material costs, and the need to maintain high service levels for just-in-time delivery to automotive manufacturers. Their implementation focused on end-to-end supply chain visibility and optimization, incorporating supplier performance data, production schedules, transportation constraints, and customer forecasts into a comprehensive AI model. The system identified opportunities to strategically position inventory at different tiers of the supply chain, reducing overall inventory investment by 27% while improving on-time delivery performance by 5 percentage points. These improvements translated to approximately $300 million in working capital reduction and significant cost savings through more efficient logistics planning. The system also enhanced resilience against disruptions, using early warning indicators to adjust inventory positions in anticipation of potential supply chain interruptions.
A consumer packaged goods company specializing in food products demonstrates how AI optimization can address industry-specific challenges, particularly for products with limited shelf life. The company implemented an AI system focused on freshness optimization across its distribution network, ensuring products reached consumers with maximum remaining shelf life while minimizing waste. The implementation incorporated detailed data on production schedules, transportation times, retailer handling practices, and historical sales patterns to optimize the entire product journey. Results included a 43% reduction in unsaleables (expired or damaged products), 29% less safety stock while maintaining service levels, and a measurable improvement in consumer perception of product freshness. The system proved particularly valuable during promotions and new product launches, using reinforcement learning to rapidly adapt stocking strategies based on early sales signals rather than waiting for complete sales cycles to establish patterns.
These diverse examples illustrate the versatility and impact of AI inventory optimization across different business contexts. While the specific technologies and implementation approaches varied based on industry requirements and organizational capabilities, all cases shared common elements: comprehensive data integration, sophisticated predictive modeling, dynamic optimization algorithms, and careful alignment with business processes. The consistent achievement of both cost reduction and service level improvements demonstrates that AI approaches can successfully resolve the traditional inventory management dilemma, creating competitive advantages through simultaneously leaner and more responsive inventory operations. As these technologies continue to mature and become more accessible, they represent a critical capability for organizations seeking to optimize their supply chain operations in increasingly volatile and competitive markets.
Statistics & Tables
Below is a comprehensive statistical overview of AI-powered inventory optimization impact and adoption across industries:
Measuring ROI from AI Inventory Optimization
Quantifying the return on investment from AI inventory optimization initiatives requires a comprehensive approach that captures both direct financial impacts and broader operational improvements. Organizations should establish clear baseline metrics before implementation to enable accurate measurement of improvements. Key performance indicators typically include inventory turns (the number of times inventory is sold and replaced annually), carrying costs as a percentage of inventory value, service level metrics (fill rates, on-time delivery, perfect order fulfillment), forecast accuracy compared to actual demand, and obsolescence/shrinkage rates. These core metrics provide the foundation for ROI calculation, with improvements in each area contributing to the overall financial impact of the implementation. While some benefits materialize immediately through reduced safety stock requirements and improved initial forecasts, the full value develops over time as the AI system learns from additional data and adapts to changing business conditions.
The most substantial financial returns typically come from working capital reductions through lower average inventory levels. A comprehensive analysis should quantify this benefit based on the organization's weighted average cost of capital, translating inventory reductions into freed cash flow available for other investments or debt reduction. Beyond these balance sheet improvements, companies should track operational cost reductions including decreased warehouse space requirements, reduced handling costs, lower insurance premiums, and diminished obsolescence write-offs. Revenue impacts represent the third major ROI component, capturing increased sales from improved product availability, reduced stockouts, and the ability to better service high-margin orders. Properly attributing these revenue improvements requires careful analysis to isolate the impact of inventory optimization from other business factors, often using A/B testing approaches where possible or econometric modeling to control for external variables.
ROI timelines vary significantly by industry and implementation scope, with retail and consumer goods companies typically seeing faster returns (6-8 months) compared to industrial manufacturers or healthcare organizations (12-18 months). The implementation approach also impacts ROI timing, with phased rollouts often delivering incremental benefits that help fund subsequent expansion. Organizations should avoid overly narrow ROI calculations that consider only direct inventory reductions, instead capturing the comprehensive value across working capital improvements, operational efficiencies, and revenue enhancement. This holistic approach typically reveals ROI levels between 150% and 300% in the first year for successful implementations, with ongoing annual returns as the system continues to optimize inventory decisions and adapts to evolving business requirements.
Beyond financial metrics, organizations should track operational improvements that may not directly translate to short-term monetary gains but contribute to strategic objectives and long-term competitive advantages. These include improved planning efficiency (measured through reduced time spent on routine forecasting and order management), enhanced decision-making capabilities (evaluated through exception rate reductions and planning cycle time improvements), and greater organizational agility in responding to market changes or disruptions. While these benefits might not appear in traditional ROI calculations, they represent valuable outcomes that should be documented as part of the overall project assessment. Companies should also establish regular review cycles to track performance against targets and identify opportunities for expanded capabilities or scope, ensuring the system continues to deliver increasing value over time.
Future Trends in AI-Driven Inventory Management
The evolution of AI-powered inventory management continues at a rapid pace, with several emerging trends poised to further transform this critical business function. Autonomous planning represents one of the most significant advancements, moving beyond AI-generated recommendations to fully automated decision execution within defined parameters. These systems continuously monitor inventory positions, demand signals, and supply conditions, automatically initiating replenishment orders, transfer requests, or production schedules without human intervention. Organizations typically implement this capability gradually, starting with stable, predictable products before expanding to more volatile items as confidence in the system grows. Advanced implementations include automatic escalation protocols that engage human planners only for exceptions that fall outside established parameters or require special consideration, freeing inventory professionals to focus on strategic initiatives rather than routine transactions.
Edge computing capabilities are extending AI optimization to the physical inventory locations themselves, with distributed intelligence embedded directly in shelves, pallets, and containers. These systems use a combination of sensors, RFID technology, and local processing power to continuously monitor inventory status and environmental conditions without requiring constant communication with central systems. The resulting real-time visibility enables much more responsive inventory management, with adjustments made instantly based on actual conditions rather than periodic inventory checks or system estimates. For perishable goods, these systems can monitor temperature, humidity, and shelf-life conditions to prioritize inventory movement based on remaining viability rather than simple FIFO rules. In retail environments, smart shelves can trigger replenishment requests automatically when inventory reaches critical thresholds, eliminating the delays and inaccuracies associated with manual shelf checks or point-of-sale-based replenishment systems.
Blockchain integration with AI inventory systems promises to resolve persistent challenges related to supply chain visibility and data trustworthiness. By creating immutable records of inventory movements, production dates, environmental conditions, and custody transfers, blockchain technology provides the reliable data foundation necessary for AI systems to generate accurate recommendations. This capability proves particularly valuable in industries with stringent traceability requirements or where counterfeit products represent significant risks, such as pharmaceuticals, luxury goods, and aerospace components. The combination of AI analytics with blockchain verification creates systems that can not only optimize inventory positions but also verify product authenticity, monitor compliance with handling requirements, and provide complete chain-of-custody documentation. While still in relatively early adoption stages, this integration represents a powerful approach to simultaneously addressing inventory optimization and risk management objectives.
Federated learning models are emerging as a solution to the challenge of optimizing inventory across multiple organizations without compromising proprietary data. This approach allows AI systems to learn from datasets across different companies in the supply chain—manufacturers, distributors, retailers—without directly sharing the underlying information. The models train locally on each organization's data, then share only the learning outcomes rather than the raw data itself. The result is a collective intelligence that benefits from the combined insights of all participants while respecting competitive boundaries and data privacy requirements. These collaborative systems enable much more sophisticated optimization across the full supply chain, identifying opportunities for synchronized production scheduling, consolidated transportation, and strategic inventory positioning that would be impossible with organization-specific optimization approaches. Early implementations in consumer goods supply chains have demonstrated significant efficiency improvements through better alignment of production capacities with actual consumer demand signals.
Quantum computing represents perhaps the most transformative future development for inventory optimization, though still several years from mainstream commercial implementation. Traditional computing approaches struggle with the combinatorial complexity of multi-echelon inventory optimization across thousands of products and locations, often resorting to simplifications and heuristics that limit optimization potential. Quantum computing's ability to process multiple possible scenarios simultaneously makes it ideally suited for these complex optimization problems, potentially revolutionizing what's possible in inventory management. Early experimental applications show promising results, with quantum-inspired algorithms already delivering performance improvements in specific inventory optimization scenarios. As this technology matures and becomes more accessible through cloud services, it will enable true global optimization across even the most complex supply networks, identifying non-obvious inventory strategies that maximize efficiency while ensuring resilience against disruptions.
Conclusion
AI-powered inventory optimization represents one of the most compelling applications of artificial intelligence in business operations, delivering tangible benefits that directly impact financial performance and customer satisfaction. The traditional tension between minimizing inventory costs and maximizing product availability has long forced organizations into uncomfortable compromises, typically erring on the side of excess inventory to avoid stockouts. AI systems fundamentally change this equation by leveraging advanced analytics, machine learning, and optimization algorithms to achieve both objectives simultaneously. The resulting improvements—typically 15-30% inventory reductions coupled with 5-15 percentage point improvements in product availability—create substantial competitive advantages for organizations that successfully implement these capabilities. As AI technologies continue to evolve, the gap between organizations employing intelligent inventory optimization and those relying on traditional approaches will likely widen, making this capability increasingly critical for competitive success.
The implementation journey requires careful planning, cross-functional alignment, and a phased approach that builds confidence and capabilities over time. Organizations should begin with a clear assessment of current inventory challenges and prioritize use cases where AI can deliver the most significant initial value. Data quality and integration capabilities form the foundation for success, requiring investments in both technology infrastructure and organizational processes. Change management deserves particular attention, as AI inventory systems often represent a significant shift in how inventory decisions are made and the roles of inventory professionals. By addressing both technical and organizational aspects of the transformation, companies can ensure successful adoption and sustainable value creation. The most effective implementations position AI not as a replacement for human judgment but as a powerful tool that augments human capabilities, enabling inventory professionals to focus on strategic decisions while the system handles routine optimization.
As we look to the future, AI-powered inventory optimization will continue to evolve, incorporating new technologies and capabilities that further extend its value. Edge computing will bring intelligence directly to physical inventory locations, enabling real-time monitoring and response. Blockchain integration will enhance data trustworthiness and traceability, creating more reliable foundations for optimization decisions. Federated learning will enable cross-organizational optimization without compromising proprietary data, unlocking new efficiencies across supply chain partners. Quantum computing may eventually revolutionize what's possible in complex, multi-echelon optimization scenarios. Organizations that begin building AI inventory capabilities today will be best positioned to incorporate these emerging technologies as they mature, creating sustainable competitive advantages through continuously improving operational efficiency and customer satisfaction.
The compelling combination of financial returns, operational improvements, and strategic benefits makes AI-powered inventory optimization a priority investment for forward-thinking organizations across industries. By transforming this fundamental business function, companies can simultaneously reduce costs, improve customer satisfaction, and enhance organizational agility—outcomes that have traditionally required trade-offs rather than simultaneous achievement. As market volatility and supply chain complexity continue to increase, the capabilities provided by AI inventory systems will become not just competitive advantages but essential requirements for effective operations. Organizations that embrace this transformation today will not only realize immediate benefits but also build the foundation for ongoing innovation and adaptation in an increasingly dynamic business environment.
Frequently Asked Questions
What is AI-powered inventory optimization and how does it differ from traditional inventory management?
AI-powered inventory optimization uses artificial intelligence and machine learning algorithms to analyze vast amounts of data and make precise inventory decisions. Unlike traditional inventory management that relies on historical averages and basic statistical methods, AI incorporates complex variables like weather patterns, social media trends, and competitive activities to predict demand with much greater accuracy.
How much inventory reduction can companies expect after implementing AI optimization?
Most organizations achieve inventory reductions of 15-30% while maintaining or improving product availability. Results vary by industry, with electronics and fashion retail seeing the highest reductions (25-35%) and aerospace and healthcare experiencing more modest reductions (10-22%) due to service level requirements.
What data is required to implement an effective AI inventory optimization system?
At minimum, organizations need historical sales data, current inventory positions, lead times, and pricing information. More advanced systems incorporate point-of-sale transactions, promotional calendars, competitor pricing, weather forecasts, social media trends, and external events that might impact demand patterns.
How long does it typically take to implement AI inventory optimization?
Implementation timeframes range from 4-18 months depending on industry complexity and organizational readiness. Grocery retail typically sees shorter timelines (4-8 months) while aerospace and healthcare require longer implementations (12-18 months) due to regulatory requirements and supply chain complexity.
What ROI can companies expect from AI inventory optimization?
Most organizations achieve positive ROI within 9-12 months, with first-year returns ranging from 150-300% of investment. This comes through combined benefits of working capital reduction, decreased operating costs, and increased revenue from better product availability.
How does AI inventory optimization improve product availability?
AI systems improve availability by predicting demand patterns with greater accuracy, identifying potential stockout risks before they occur, and dynamically adjusting inventory positions across locations. This typically improves in-stock rates by 5-15 percentage points while simultaneously reducing overall inventory levels.
What organizational changes are required for successful implementation?
Successful implementation requires cross-functional alignment across supply chain, IT, finance, and sales departments. The role of inventory planners evolves from transaction processing to exception management and strategic decision-making, requiring training and change management support.
How do AI inventory systems handle supply chain disruptions?
Advanced AI systems continuously monitor for disruption signals from suppliers, transportation networks, and external events. They use early warning indicators to automatically adjust inventory positions, suggest alternative sources, and recalculate optimal buffer levels to maintain business continuity during disruptions.
Can AI inventory optimization work with existing ERP and supply chain systems?
Yes, most AI inventory solutions are designed to integrate with existing ERP and supply chain systems. They typically sit as an analytical layer above transactional systems, receiving data inputs and providing recommendations that feed back into execution platforms.
What future developments are expected in AI inventory optimization?
Emerging trends include autonomous planning with minimal human intervention, edge computing that brings intelligence directly to physical inventory locations, blockchain integration for enhanced traceability, federated learning across supply chain partners, and eventually quantum computing applications for complex optimization scenarios.
Additional Resources
"Supply Chain Visibility: The Ultimate Guide" - A comprehensive DataSumi guide exploring how enhanced visibility enables more effective inventory optimization.
"AI Implementation Best Practices for Supply Chain Management" - DataSumi's practical framework for successfully implementing AI solutions in supply chain operations.
"The Future of AI in Supply Chain Management" - An exploration of emerging technologies and approaches shaping next-generation inventory management.
"Data Cleansing Best Practices for AI Implementations" - Essential guidance on preparing data foundations for successful AI inventory optimization.
"Retail Analytics Metrics: KPIs That Drive Performance" - A detailed overview of the metrics that matter most when measuring inventory optimization success.