AI-Powered Supply Chain Resilience: Navigating Global Disruptions with Intelligent Solutions
Discover how AI technologies are transforming supply chain resilience in the wake of recent global disruptions. Learn actionable strategies to implement AI-driven solutions for more adaptive and crisis-resistant supply networks.
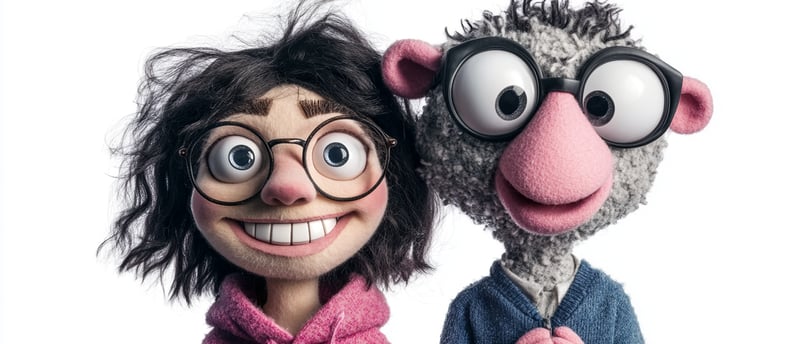
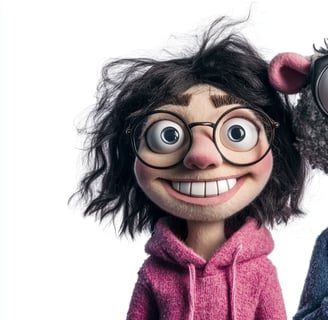
The phrase "supply chain disruption" has become all too familiar in boardrooms and households alike over the past few years. From the unprecedented global pandemic to geopolitical conflicts, extreme weather events, and cybersecurity threats, supply chains worldwide have experienced a perfect storm of challenges that exposed critical vulnerabilities in traditional operating models. These disruptions have resulted in empty store shelves, manufacturing delays, skyrocketing logistics costs, and billions in lost revenue. Against this backdrop of uncertainty, artificial intelligence has emerged as a powerful ally in the quest for supply chain resilience. By harnessing the predictive, adaptive, and analytical capabilities of AI, organizations are not merely responding to disruptions—they're anticipating them, modeling potential impacts, and orchestrating intelligent responses that minimize business disruption. This article explores how AI-powered solutions are revolutionizing supply chain resilience, drawing valuable lessons from recent global disruptions and providing a roadmap for organizations seeking to fortify their operations against future shocks.
Understanding Recent Global Supply Chain Disruptions
The fragility of global supply chains was laid bare beginning in early 2020 when the COVID-19 pandemic triggered unprecedented disruptions across nearly every industry. Manufacturing facilities shut down, transportation networks faltered, and consumer demand patterns underwent dramatic shifts almost overnight. The pandemic exposed the inherent vulnerabilities of just-in-time inventory systems and revealed dangerous dependencies on single-source suppliers or geographically concentrated production hubs. According to research from McKinsey, companies experienced supply chain disruptions lasting a month or longer every 3.7 years on average, with the most severe events causing significant financial damage. The pandemic's impact was particularly severe, with 94% of Fortune 1000 companies reporting supply chain disruptions and 75% reporting negative or strongly negative impacts on their businesses.
Beyond the pandemic, geopolitical tensions have increasingly strained global supply networks in ways that were difficult to predict using traditional methods. The Russia-Ukraine conflict created immediate shortages in energy, agricultural products, and critical raw materials, while ongoing U.S.-China trade tensions have forced companies to reevaluate decades-old sourcing strategies. Climate-related disruptions have added another layer of complexity, with extreme weather events becoming more frequent and severe. Hurricane Ian in 2022, for instance, caused an estimated $50 billion in economic damage and disrupted supply chains across multiple industries. The frequency of weather-related supply chain disruptions has increased by approximately 29% since 2012, according to data from Resilinc's EventWatch.
Cybersecurity threats represent yet another growing source of supply chain vulnerability that traditional risk management approaches struggle to address effectively. The 2021 attack on Colonial Pipeline demonstrated how a single cybersecurity breach could disrupt fuel supplies across an entire region of the United States. Similarly, the SolarWinds hack revealed how software supply chains could be compromised to infiltrate thousands of organizations simultaneously. These multi-faceted disruptions have collectively shattered the illusion that global supply chains would always function reliably with minimal oversight or contingency planning. They have also demonstrated that traditional approaches to supply chain management—often relying on historical data, limited visibility, and manual decision-making processes—are inadequate for navigating the complexity and volatility of today's business environment.
The cascading nature of these disruptions has been particularly challenging, as problems in one area quickly spread to create bottlenecks elsewhere in the supply network. The semiconductor shortage that began during the pandemic illustrates this phenomenon perfectly. What started as a temporary manufacturing shutdown quickly escalated into a global crisis affecting industries from automotive to consumer electronics, with ripple effects still being felt today. These experiences have forced a fundamental reconsideration of supply chain priorities, shifting focus from maximum efficiency and minimum cost to greater resilience, visibility, flexibility, and risk management. This paradigm shift has created the perfect conditions for AI adoption, as organizations seek technologies capable of processing vast amounts of data, identifying emerging risks, and adapting quickly to changing circumstances.
The Role of AI in Supply Chain Resilience
Artificial intelligence represents a fundamental shift in how organizations approach supply chain resilience rather than merely an incremental improvement to existing processes. At its core, AI enables supply chains to become more intelligent, responsive, and forward-looking systems that can detect patterns, anticipate problems, and adapt to changing conditions in ways that traditional approaches simply cannot match. The transformative potential of AI stems from its ability to process and analyze massive datasets from disparate sources, uncovering insights and connections that would remain invisible to human analysts or conventional analytics tools. When applied to supply chain resilience, these capabilities allow organizations to move from reactive disruption management to proactive risk mitigation and rapid adaptation.
The predictive power of AI serves as the foundation for enhanced supply chain resilience by enabling organizations to anticipate potential disruptions before they occur or escalate. Machine learning algorithms can analyze historical disruption patterns alongside real-time data from suppliers, transportation networks, weather systems, social media, news sources, and financial markets to identify early warning signals of emerging risks. Research from Gartner indicates that organizations using AI for predictive analytics are able to reduce forecasting errors by up to 38% and identify potential supply chain disruptions an average of 25 days earlier than those using conventional methods. This early detection capability provides crucial time for implementing contingency plans, securing alternative suppliers, adjusting inventory levels, or rerouting shipments to minimize disruption impacts.
Beyond prediction, AI enhances supply chain resilience through improved visibility and transparency across complex, multi-tier networks. Traditional supply chains often suffer from limited visibility beyond direct suppliers, with little insight into the operations, risks, or dependencies of tier 2 and tier 3 suppliers. AI-powered supply chain mapping and monitoring tools can illuminate these hidden relationships by aggregating and analyzing data from multiple sources, creating comprehensive digital representations of the entire supply network. According to research from Interos, companies leveraging AI for supply chain visibility have achieved an 87% improvement in their ability to detect risks originating from tier 2+ suppliers. This enhanced visibility allows organizations to identify critical dependencies, single points of failure, and potential bottlenecks that might otherwise remain undiscovered until a disruption occurs.
Perhaps most importantly, AI enables supply chains to become more adaptive and responsive when disruptions do occur, minimizing their operational and financial impact. AI-driven decision support systems can rapidly evaluate multiple response scenarios, weighing complex trade-offs and recommending optimal courses of action based on organizational priorities and constraints. These systems can orchestrate coordinated responses across the supply network, automatically adjusting production schedules, reallocating inventory, identifying alternative suppliers, or modifying transportation routes to maintain business continuity. A BCG study found that companies with AI-powered supply chains demonstrated 60-80% faster response times during disruptions compared to those without such capabilities, resulting in significantly reduced revenue impacts and faster recovery times.
Key AI Technologies Transforming Supply Chain Management
Machine learning stands at the forefront of AI technologies revolutionizing supply chain resilience, with applications spanning from demand forecasting to risk identification and mitigation planning. Advanced ML algorithms can process hundreds of variables simultaneously, learning complex patterns and relationships that traditional statistical methods cannot capture. In demand forecasting, for instance, ML models incorporate diverse factors including historical sales data, seasonality, pricing changes, competitor actions, macroeconomic indicators, and even social media sentiment to generate more accurate predictions. During the pandemic, organizations utilizing ML-based forecasting systems were able to adapt to dramatic demand shifts 3-4 times faster than those using traditional methods, according to research from McKinsey. This forecasting precision directly enhances resilience by ensuring appropriate inventory levels and resource allocation, even during periods of high volatility.
Digital twins represent another transformative AI technology that is fundamentally changing how organizations plan for and respond to supply chain disruptions. These virtual replicas of physical supply networks integrate real-time data from IoT sensors, ERP systems, and external sources to simulate operations, test scenarios, and optimize responses. During the semiconductor shortage, companies like Merck utilized digital twins to model the impact of component shortages across their production network and evaluate different mitigation strategies before implementing them in the real world. Gartner reports that organizations employing digital twins for supply chain planning have reduced disruption impacts by up to a third compared to those without such capabilities. The technology's value lies in its ability to provide a safe environment for testing various disruption scenarios and response strategies, allowing organizations to develop more effective contingency plans and make faster decisions when actual disruptions occur.
Natural language processing (NLP) capabilities enable supply chain systems to monitor and analyze unstructured data sources that often contain the earliest signals of potential disruptions. AI systems equipped with NLP can continuously scan news reports, social media feeds, regulatory announcements, and supplier communications for relevant information about events that might impact supply chain operations. For example, systems from companies like Everstream Analytics use NLP to monitor global events and provide early warnings about potential disruptions, from factory fires to port congestion, often days or weeks before these issues would otherwise become apparent. During the Suez Canal blockage in 2021, organizations with advanced NLP-based monitoring systems received alerts about the incident approximately 18 hours before it became widely reported, allowing them critical time to begin rerouting shipments and notifying customers of potential delays.
Computer vision combined with IoT sensors is transforming supply chain visibility and quality control, enhancing resilience by ensuring real-time monitoring of goods and facilities. These technologies enable automated inspection of products, verification of shipping conditions, and detection of anomalies across the supply chain. During the pandemic, pharmaceutical companies deployed computer vision systems to monitor vaccine production and distribution, ensuring temperature control and handling requirements were consistently met despite strained logistics networks. In warehouse operations, computer vision systems from companies like Fizyr and Covariant have enabled more flexible robotics applications that can adapt to changing products and conditions, maintaining operational continuity even when human workers are unavailable. The combination of these visual monitoring capabilities with predictive analytics creates powerful early warning systems that can identify potential quality issues or bottlenecks before they escalate into major disruptions.
Reinforcement learning algorithms are particularly valuable for supply chain resilience because they excel at optimizing complex processes in dynamic environments. Unlike supervised learning approaches that require extensive historical data, reinforcement learning allows AI systems to learn optimal strategies through trial and error in simulated environments. Companies like JD.com have applied reinforcement learning to optimize inventory allocation across their distribution network, automatically adjusting stocking levels based on shifting demand patterns and transportation constraints. During COVID-19, these systems demonstrated remarkable adaptability, learning new optimal strategies as consumer behavior and supply conditions changed dramatically. According to research from Accenture, organizations implementing reinforcement learning for supply chain optimization have achieved 15-25% reductions in logistics costs while simultaneously improving resilience metrics such as order fulfillment rates and on-time delivery performance.
Implementation Strategies for AI-Powered Supply Chain Resilience
Successful AI implementation for supply chain resilience begins with a methodical assessment of current vulnerabilities and capabilities rather than a rush to adopt the latest technologies. Organizations should conduct thorough supply chain risk assessments to identify critical weaknesses, single points of failure, and areas where enhanced visibility or decision support would deliver the greatest resilience benefits. This process typically involves mapping the end-to-end supply network, including tier 2+ suppliers, and evaluating historical disruptions to identify recurring patterns or challenges. A survey by Deloitte found that companies taking this structured assessment approach were 2.5 times more likely to achieve positive ROI from their AI supply chain investments compared to those pursuing technology-first strategies. Once these priority areas are identified, organizations can develop a phased implementation roadmap that addresses the most critical resilience gaps first while building foundational capabilities for more advanced applications.
Data quality and integration represent fundamental challenges that must be addressed early in any AI implementation journey. Even the most sophisticated algorithms will produce poor results if trained on inaccurate, incomplete, or outdated information. Organizations should invest in establishing reliable data pipelines that consolidate information from internal systems, supplier networks, logistics partners, and external sources into unified data platforms that can feed AI applications. According to Gartner, data quality issues are the primary reason for AI project failures, with 64% of organizations citing poor data quality as their biggest obstacle to successful implementation. Cloud-based data integration platforms from vendors like Datasumi can accelerate this process by providing pre-built connectors for common supply chain systems and standardized data models specifically designed for supply chain analytics.
Selecting the right technological approach is critical for balancing quick wins with long-term resilience goals. Many organizations find success with a hybrid strategy that combines targeted point solutions for immediate needs with investments in more comprehensive platforms for the future. For example, a company might deploy a specialized AI solution for demand forecasting while simultaneously laying the groundwork for an integrated control tower that will eventually orchestrate end-to-end supply chain operations. Research from IDC indicates that organizations following this balanced approach achieve positive ROI from their initial AI implementations approximately 40% faster than those pursuing either point solutions or comprehensive platforms exclusively. Cloud-based solutions often offer advantages for supply chain resilience applications by providing greater scalability, faster implementation, and built-in connectivity with external data sources and partner networks.
Change management and skills development deserve as much attention as the technical aspects of AI implementation. Supply chain professionals accustomed to making decisions based on experience and intuition may be reluctant to trust AI-generated recommendations, particularly in high-pressure disruption scenarios. Organizations should invest in comprehensive training programs that help employees understand how AI systems work, when to trust their outputs, and how to effectively collaborate with these new tools. A BCG study found that companies that invested at least 10% of their AI project budgets in change management and skills development were three times more likely to report successful outcomes than those that invested less. This human element becomes even more critical as AI systems take on increasingly autonomous roles in supply chain decision-making, requiring new governance structures and oversight mechanisms to ensure appropriate use.
Measuring success through well-defined metrics helps maintain momentum and secure ongoing support for AI resilience initiatives. Organizations should establish clear KPIs that directly connect to resilience objectives, such as time to detect disruptions, response time to events, percentage of disruptions successfully mitigated, or financial impact of disruptions relative to baseline periods. These metrics should be tracked consistently and communicated broadly to demonstrate the value of AI investments and guide continuous improvement efforts. According to research from MIT, companies that implemented rigorous measurement frameworks for their AI supply chain initiatives were 76% more likely to expand these programs after initial pilots compared to those without such frameworks. Successful organizations also recognize that AI implementation is an iterative journey rather than a one-time project, requiring ongoing refinement as supply chain conditions evolve and new capabilities emerge.
Case Studies: Successful AI Integration in Supply Chains
Procter & Gamble's implementation of AI-powered supply chain resilience offers compelling evidence of the technology's potential to mitigate the impact of major disruptions. When COVID-19 dramatically shifted consumer demand patterns and disrupted production capabilities, P&G leveraged its AI-driven control tower to quickly identify emerging risks and opportunities across its global network. The system analyzed data from more than 200 internal and external sources to create real-time visibility into potential bottlenecks and automatically generated mitigation recommendations prioritized by business impact. This capability enabled P&G to maintain 99.5% product availability for essential items like cleaning supplies and personal care products despite unprecedented demand fluctuations. The company's AI system also facilitated rapid supply chain reconfiguration, automatically identifying alternative suppliers when primary sources became unavailable and optimizing production schedules to prioritize high-demand items. According to P&G's supply chain leadership, this AI-enabled resilience preserved an estimated $500 million in revenue that would otherwise have been lost to stockouts and production disruptions.
Merck's application of digital twins and simulation technologies demonstrates how pharmaceutical companies can leverage AI to enhance supply chain resilience in highly regulated environments. Following previous disruptions that affected critical medication availability, Merck developed a comprehensive digital twin of its global supply network, incorporating detailed models of manufacturing facilities, transportation routes, and inventory positions. This virtual replica enabled the company to run thousands of simulations testing different disruption scenarios and response strategies, ranging from supplier failures to transportation disruptions and demand spikes. When Hurricane Maria damaged Puerto Rican manufacturing facilities in 2017, Merck's AI-powered system evaluated 30+ response scenarios within hours, identifying optimal production reallocation that minimized patient impact while considering complex constraints like regulatory requirements and quality control procedures. The company has subsequently expanded its digital twin capabilities to incorporate real-time data streams and autonomous decision-making for certain scenarios, reducing disruption response time by approximately 60% and maintaining critical medication availability during subsequent challenges, including the COVID-19 pandemic.
Maersk's transformation from traditional shipping company to AI-powered logistics provider illustrates how the technology can enhance resilience across entire supply chain ecosystems. Following the NotPetya cyberattack in 2017, which cost the company approximately $300 million, Maersk accelerated its digital transformation with a particular focus on predictive risk intelligence. The company now employs machine learning algorithms that analyze over 30 million data points daily from sources including vessel locations, port conditions, weather patterns, customs data, and even social media to identify potential disruptions before they impact operations. During the Suez Canal blockage, Maersk's AI systems detected the incident within minutes and immediately began generating alternative routing recommendations for hundreds of affected vessels, prioritizing critical cargo and considering factors like fuel consumption, port capacity, and downstream scheduling impacts. The company also leverages reinforcement learning to continuously optimize container positioning across its global network, ensuring empty containers are available where needed despite disruptions. According to Maersk's digital transformation team, these AI capabilities have reduced the average impact of major disruptions by approximately 35% while simultaneously improving overall network efficiency.
Walmart's deployment of AI for multi-tier visibility and risk management demonstrates how retailers can enhance resilience across complex global supply networks. The company has developed an integrated supply chain intelligence platform that maintains near real-time visibility into more than 100,000 suppliers across multiple tiers, processing data from diverse sources including supplier systems, logistics providers, weather services, news feeds, and financial markets. Machine learning algorithms automatically identify potential disruption signals, evaluate their likelihood and business impact, and trigger appropriate responses based on predefined playbooks. During the early stages of the pandemic, this system enabled Walmart to identify vulnerable supplier categories 2-3 weeks before actual disruptions occurred, providing crucial time to secure additional inventory and develop alternative sourcing strategies. The company's AI capabilities also supported rapid adaptation to changing consumer behaviors, with natural language processing algorithms analyzing online searches, social media, and news coverage to predict demand shifts for essential items. According to Walmart executives, these AI-enabled resilience capabilities helped the company maintain approximately 7% higher in-stock levels for critical products compared to competitors during peak pandemic disruptions.
Small and medium enterprises can also achieve significant resilience improvements through targeted AI implementations, as demonstrated by Celsius Logistics, a regional cold chain provider. Recognizing that full-scale AI transformation was beyond its immediate resources, Celsius adopted a focused approach prioritizing resilience for its most vulnerable operations. The company implemented a cloud-based machine learning solution specifically designed to predict refrigeration equipment failures across its distribution centers and transportation fleet, combining data from IoT sensors with maintenance records and environmental conditions. This targeted system detected early warning signs of potential failures with 92% accuracy, enabling preventive maintenance that avoided an estimated 37 major disruptions during its first year of operation. Celsius subsequently expanded its AI capabilities to include demand forecasting and route optimization, achieving these enhancements through pre-built supply chain AI services rather than custom development. This incremental approach delivered significant resilience improvements—including a 28% reduction in service disruptions and 15% decrease in emergency maintenance costs—while requiring relatively modest investment suitable for the company's scale and resources.
Challenges and Limitations of AI in Supply Chain Resilience
Data quality and integration challenges present significant barriers to effective AI implementation for supply chain resilience. Many organizations struggle with fragmented data landscapes where critical information resides in disparate systems with inconsistent formats, quality standards, and update frequencies. This fragmentation is particularly problematic for supply chain applications, which require comprehensive visibility across organizational boundaries to deliver meaningful resilience benefits. A survey by Capgemini found that 64% of companies cited data quality issues as their primary obstacle to successful AI implementation in supply chains. The problem becomes even more acute when attempting to incorporate external data from suppliers, logistics providers, or market intelligence sources, which often use different data models and taxonomies. Organizations frequently underestimate the time and resources required for data preparation, with McKinsey reporting that data scientists typically spend 50-80% of their time cleaning and organizing data rather than building and optimizing models. Without addressing these fundamental data challenges, AI systems may generate inaccurate predictions or recommendations that undermine trust and limit adoption.
Algorithmic transparency and explainability represent growing concerns as AI takes on more critical roles in supply chain decision-making. During disruptions, supply chain leaders need to understand not only what actions an AI system recommends but also why those actions make sense given the specific circumstances. Black-box AI models that cannot explain their reasoning face significant adoption barriers in high-stakes resilience applications, where decisions may have substantial financial and operational consequences. Research from MIT indicates that supply chain professionals are approximately 60% more likely to follow AI recommendations when the system provides clear explanations for its logic. This explainability challenge becomes particularly acute in regulated industries like pharmaceuticals or food, where companies must demonstrate sound reasoning behind supply chain decisions that might affect product safety or availability. Balancing the sophistication of AI models with appropriate levels of transparency remains a significant technical and design challenge that many organizations struggle to address effectively.
The talent gap in AI and advanced analytics continues to limit the pace and scale of supply chain resilience transformations. Organizations require professionals who understand both supply chain operations and AI technologies—a relatively rare combination in today's job market. According to a 2023 survey by Deloitte, 76% of companies reported difficulty recruiting and retaining the specialized talent needed for AI supply chain initiatives. This shortage extends beyond data scientists to include roles like AI translators who can bridge the gap between technical and business teams, change management specialists who can drive adoption, and domain experts who can validate models and results. The talent constraint often forces difficult trade-offs between building internal capabilities versus partnering with external providers, with each approach offering distinct advantages and limitations for long-term resilience. Organizations that underinvest in talent development may achieve short-term gains from initial AI implementations but struggle to sustain momentum or adapt these systems as supply chain conditions evolve.
Ethical considerations and unintended consequences require careful attention as AI increasingly influences supply chain decisions. Algorithms trained on historical data may perpetuate or amplify existing biases in supplier selection, resource allocation, or market prioritization during disruptions. For example, AI systems optimizing for maximum financial return might consistently deprioritize smaller markets or vulnerable populations when allocating scarce resources during shortages, creating ethical dilemmas and potential reputational risks. These concerns extend to environmental impact as well, with AI systems potentially recommending actions that optimize for short-term resilience at the expense of sustainability goals. According to research from the MIT Sloan Management Review, fewer than 30% of organizations implementing AI for supply chain applications have established formal governance frameworks addressing ethical considerations and potential unintended consequences. Without appropriate oversight mechanisms, companies risk deploying systems that technically enhance resilience metrics while undermining broader organizational values or social responsibilities.
Integration with legacy systems and processes presents practical challenges that often delay or diminish the benefits of AI implementations. Many supply chains rely on older enterprise systems that were not designed for the real-time data exchange and computational requirements of modern AI applications. Upgrading or replacing these systems typically requires significant investment and carries substantial operational risk, creating natural hesitation among leadership teams. Even when technical integration is achieved, organizations frequently struggle to modify established business processes and decision-making structures to fully leverage AI capabilities. A Boston Consulting Group study found that approximately 70% of supply chain AI projects delivered less value than expected, with integration challenges cited as the primary reason for underperformance. These difficulties are particularly pronounced in organizations with complex global operations spanning multiple business units, each potentially using different systems and following different processes. Successfully navigating these integration challenges requires strong executive sponsorship, cross-functional collaboration, and realistic expectations about implementation timelines and change management requirements.
Future Outlook: Emerging Trends in AI-Powered Supply Chains
Autonomous supply chain orchestration represents the next frontier in AI-powered resilience, with systems evolving from advisory roles to increasingly independent decision-making and execution capabilities. Future supply chains will feature AI orchestrators that continuously monitor operations, detect deviations from optimal patterns, and automatically implement corrective actions within predefined parameters. Research from Gartner suggests that by 2026, more than 25% of large enterprises will have implemented some form of autonomous supply chain orchestration, up from less than 5% today. These systems will leverage reinforcement learning to continuously improve their response strategies, learning from the outcomes of previous disruptions to enhance future performance. The evolution toward greater autonomy will require significant advances in both technical capabilities and governance structures, as organizations establish appropriate boundaries and oversight mechanisms for AI decision authority. Leaders in this space are already developing tiered autonomy models that grant AI systems different levels of independent action based on the nature and severity of disruptions, similar to approaches used in autonomous vehicle development.
Multi-agent AI systems will enhance resilience by enabling more sophisticated collaboration across organizational boundaries. Traditional supply chain technologies typically operate within the confines of a single enterprise, but disruptions rarely respect these boundaries. Future resilience applications will feature networks of specialized AI agents representing different entities and functions across the supply ecosystem, each pursuing local objectives while collaborating toward system-wide resilience goals. For example, an AI agent representing a manufacturer might negotiate in real-time with agents representing logistics providers, component suppliers, and retail partners to rapidly reconfigure operations during a disruption event. Research from the MIT Center for Transportation and Logistics indicates that these multi-agent approaches can reduce system-wide disruption costs by up to 35% compared to traditional centralized planning methods. However, realizing these benefits will require new standards for secure information sharing, coordination protocols, and trust mechanisms that enable effective collaboration while protecting proprietary information and competitive interests.
Quantum computing promises to address computational limitations that currently constrain AI's ability to optimize complex supply networks under disruption scenarios. While classical computing struggles with the combinatorial complexity of large-scale supply chain optimization problems, quantum algorithms could potentially evaluate millions of possible response scenarios in seconds to identify truly optimal solutions. Companies including Volkswagen, ExxonMobil, and DHL have already begun exploring quantum applications for supply chain resilience, focusing on areas like network design, multi-echelon inventory optimization, and real-time logistics routing during disruptions. Although practical quantum advantage for production supply chain applications remains several years away, early experimental results suggest potential performance improvements of several orders of magnitude for certain optimization problems. Organizations should monitor quantum developments and consider how future capabilities might transform their resilience strategies, potentially enabling new approaches that are computationally infeasible with current technologies.
AI-human collaborative intelligence will evolve beyond today's relatively simple advisory systems to create more sophisticated partnerships that leverage the complementary strengths of human judgment and machine computation. Future resilience applications will feature bi-directional learning, with AI systems adapting based on human feedback while simultaneously helping humans recognize and overcome cognitive biases in disruption response. According to research from Harvard Business School, these collaborative intelligence approaches can improve decision quality by up to 60% compared to either humans or AI working independently. Next-generation interfaces will facilitate this collaboration through multimodal interaction, allowing supply chain professionals to engage with AI systems through natural language, visual interfaces, and immersive environments that provide intuitive representations of complex supply networks. Organizations exploring these capabilities, like Datasumi's AI-Powered Planning Suite, are focused on creating systems that augment human capabilities rather than replacing them, recognizing that optimal resilience requires both computational power and human creativity, especially for unprecedented disruption scenarios.
Integrated sensing networks combining IoT, edge AI, and 5G connectivity will provide unprecedented real-time visibility into physical supply chain operations and environmental conditions. These networks will feature millions of connected sensors monitoring everything from individual shipments to infrastructure conditions and environmental factors that might impact supply chains. Edge AI capabilities will enable intelligent filtering and analysis at the point of data collection, ensuring that only relevant information is transmitted to central systems for further processing. According to Ericsson Research, the combination of 5G and edge computing will reduce latency for critical supply chain alerts by approximately 75% compared to current technologies, enabling faster disruption detection and response. Progressive organizations are already developing digital sensing strategies that extend beyond their own operations to include suppliers, logistics networks, and even relevant public infrastructure. These capabilities will be particularly valuable for enhancing resilience against physical disruptions like extreme weather events, transportation infrastructure failures, or manufacturing facility incidents that require immediate detection and response.
Conclusion
The convergence of artificial intelligence and supply chain management represents a pivotal development in organizational resilience, fundamentally changing how companies anticipate, prepare for, and respond to disruptions. The experiences of recent years have demonstrated beyond doubt that traditional approaches to supply chain management—focused primarily on efficiency and cost optimization—are inadequate for navigating the complexity and volatility of today's global business environment. As disruptions become more frequent, severe, and multifaceted, AI provides the computational power, predictive capability, and decision support needed to maintain operational continuity even under challenging conditions. The organizations that have successfully implemented these technologies have gained significant competitive advantages during recent disruptions, maintaining higher service levels, responding more quickly to changing conditions, and recovering faster from unavoidable impacts.
The path toward AI-powered supply chain resilience is neither simple nor universal, with each organization facing unique challenges based on its industry, scale, existing capabilities, and specific vulnerability profile. Data quality and integration challenges remain significant hurdles for many companies, requiring sustained investment and organizational commitment to overcome. Talent limitations, integration with legacy systems, and governance considerations further complicate implementation efforts, demanding thoughtful strategies that balance technical requirements with human and organizational factors. Despite these challenges, the ROI potential of AI for supply chain resilience is compelling, with leading organizations reporting substantial reductions in disruption impacts and corresponding financial benefits. The technology's ability to simultaneously enhance efficiency under normal conditions while providing adaptive capacity during disruptions creates a strong business case that extends beyond mere risk management.
Looking forward, the evolution of AI capabilities promises even greater transformations in how supply chains respond to disruptions. Autonomous orchestration, multi-agent systems, quantum computing applications, and advanced collaborative intelligence will create resilience capabilities that far exceed current possibilities. Organizations that begin building foundational capabilities today will be best positioned to leverage these emerging technologies as they mature, creating sustainable competitive advantages in an increasingly volatile business environment. The most successful companies will view AI not merely as a technological solution but as a catalyst for broader transformation in how they design, manage, and evolve their supply networks. By embracing this perspective and committing to the necessary investments in technology, talent, and organizational change, forward-thinking leaders can create supply chains that don't just survive disruptions but thrive through them, turning potential crises into opportunities for differentiation and growth.
FAQ Section
How is AI helping to prevent supply chain disruptions? AI helps prevent supply chain disruptions through predictive analytics that forecast potential issues, enhanced visibility across multi-tier networks, and early warning systems that detect emerging risks from various data sources including news, weather, and supplier performance metrics.
What is the ROI for implementing AI in supply chain resilience? Organizations implementing AI for supply chain resilience typically see ROI through multiple channels: 25-30% inventory cost reduction, 15-25% logistics cost savings, 38% improvement in forecast accuracy, and potentially millions saved by preventing or mitigating major disruptions.
What are digital twins in supply chain management? Digital twins are virtual replicas of physical supply chains that integrate real-time data to simulate operations, test scenarios, and optimize responses to disruptions before implementing them in the real world. They enable organizations to virtually test the impact of potential disruptions and evaluate mitigation strategies.
How do machine learning algorithms improve supply chain forecasting? Machine learning algorithms improve forecasting by analyzing hundreds of variables simultaneously, identifying non-obvious patterns in historical data, incorporating external factors like weather and social media trends, and continuously learning from forecasting errors to improve future predictions.
What challenges do companies face when implementing AI for supply chain resilience? Key challenges include poor data quality and integration across systems, lack of visibility into tier 2+ suppliers, shortage of talent with both AI and supply chain expertise, algorithmic transparency issues, and organizational resistance to AI-driven decision-making processes.
How did companies with AI-powered supply chains perform during the COVID-19 pandemic? Companies with advanced AI-powered supply chains demonstrated 3-7x faster response to disruptions during COVID-19, maintained 15-30% better product availability, and recovered to normal operations approximately 50% faster than competitors without AI capabilities.
What's the difference between AI for supply chain efficiency versus resilience? AI for efficiency typically focuses on cost reduction and operational optimization under normal conditions, while AI for resilience emphasizes adaptability, risk detection, scenario planning, and rapid response capabilities during disruptions, often accepting some efficiency trade-offs for greater flexibility.
How can small and medium enterprises implement AI for supply chain resilience? SMEs can start with focused applications like demand forecasting or supplier risk monitoring, leverage cloud-based AI solutions that don't require significant infrastructure investment, partner with specialized service providers, and prioritize data quality improvements before expanding to more advanced applications.
What role does natural language processing play in supply chain resilience? NLP enables supply chain resilience by monitoring news, social media, and other text sources for early warning signs of disruptions, automatically extracting key information from supplier communications, analyzing customer sentiment that might affect demand, and improving documentation processes across the supply network.
How will quantum computing impact supply chain resilience in the future? Quantum computing will revolutionize supply chain resilience by solving complex network optimization problems beyond classical computing capabilities, enabling real-time simulation of thousands of disruption scenarios, optimizing multi-objective resilience parameters simultaneously, and creating more robust network designs that balance efficiency with disruption resistance.