Applying Data Analytics in the Manufacturing
The Manufacturing industry has witnessed significant advancements in recent years, thanks to the integration of data analytics into its processes. By leveraging the power of data analytics, manufacturers can uncover valuable insights, optimize operations, and ultimately improve efficiency across the board.
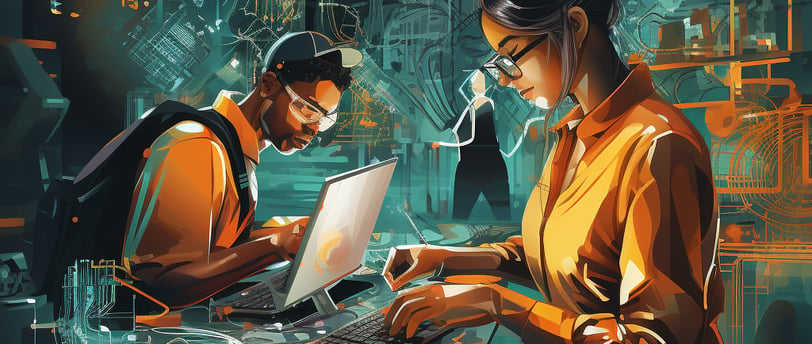
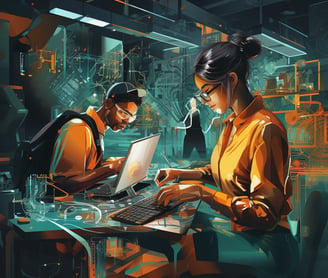
The integration of data analytics in the manufacturing industry has brought about significant advancements, offering real-time insights, process improvements, and predictive maintenance. By leveraging data analytics, manufacturers can streamline warehouse operations, improve demand forecasting, enhance quality control, and achieve end-to-end visibility. This integration has become even more crucial in the face of challenges such as the COVID-19 pandemic, which has intensified the need for efficiency and agility in manufacturing.
Data analytics in manufacturing involves capturing, cleansing, and analyzing machine data to predict future use, prevent failures, forecast maintenance requirements, and identify areas for improvement. The practice of manufacturing analytics is a vital component of modern manufacturing, enabling companies to automate traditional processes, reduce costs, and improve efficiency. The market for manufacturing analytics is projected to grow significantly, with the global manufacturing analytics market size predicted to reach $28.5 billion by 2026.
Some key use cases of manufacturing analytics include demand forecasting, inventory management, predictive maintenance, supply chain optimization, risk management, price optimization, order management, and maintenance optimization. These use cases demonstrate the diverse applications of data analytics in addressing various aspects of manufacturing operations.
While the potential benefits of data analytics in manufacturing are substantial, it's important to note that its successful implementation requires careful consideration of factors such as data management, domain expert involvement, process and culture change, and the adoption of modern data analysis tools. Additionally, the use of technologies such as robotic process automation (RPA) and the Internet of Things (IoT) can further enhance the capabilities of manufacturing analytics.
What is Data Analytics?
Data analytics refers to the process of examining vast sets of data to uncover patterns, correlations, and insights that can drive informed decision-making. When applied to the manufacturing process, data analytics enables organizations to transform raw data from various sources, such as equipment sensors, production lines, and supply chain, into actionable intelligence. By analyzing this data, manufacturers can identify inefficiencies, spot trends, predict maintenance needs, and optimize resource allocation, leading to improved efficiency and reduced costs.
Data analytics in manufacturing involves the examination of extensive data sets to reveal patterns, correlations, and insights that drive informed decision-making. This process transforms raw data from various sources, such as equipment sensors, production lines, and the supply chain, into actionable intelligence. By analyzing this data, manufacturers can identify inefficiencies, predict maintenance needs, and optimize resource allocation, leading to improved efficiency and reduced costs.
Manufacturing analytics is a vital component of modern manufacturing, enabling real-time insights, process improvements, and predictive maintenance. It involves capturing, cleansing, and analyzing machine data to predict future use, prevent failures, forecast maintenance requirements, and identify areas for improvement. The practice of manufacturing analytics is a critical step for manufacturing digital transformation required for Industry 4.0, which aims to automate traditional manufacturing processes, reduce costs, and improve efficiency.
The benefits of data analytics in manufacturing are substantial and include more accurate demand forecasting, improved quality control, more efficient inventory management, and true end-to-end visibility. The use cases of manufacturing analytics range from demand forecasting and inventory management to predictive maintenance, risk management, price optimization, and order management.While the potential benefits of data analytics in manufacturing are significant, its successful implementation requires careful consideration of factors such as data management, domain expert involvement, process and culture change, and the adoption of modern data analysis tools. Additionally, the use of technologies such as robotic process automation (RPA) and the Internet of Things (IoT) can further enhance the capabilities of manufacturing analytics.
Benefits of Data Analytics in Manufacturing
One of the key concerns when implementing data analytics in the manufacturing process is data quality. The success of any data analytics initiative relies heavily on the availability of accurate and reliable data. Manufacturers must ensure that the data collected is consistent, complete, and of high quality. Inconsistent or incomplete data can lead to flawed analysis and erroneous insights, rendering the entire data analytics effort ineffective. To address this concern, organizations can implement robust data collection mechanisms, invest in data cleansing and validation processes, and establish data governance practices to maintain data integrity.
Another crucial concern is data security. Manufacturers deal with sensitive information related to production processes, trade secrets, and customer data. As data analytics involves the collection and analysis of vast amounts of data, it becomes imperative to establish robust cybersecurity measures to protect against potential breaches or unauthorized access. Data encryption, access controls, regular security audits, and employee training on data security best practices are some of the measures manufacturers can adopt to safeguard their data and maintain the trust of their stakeholders.
Despite these concerns, the benefits of applying data analytics in the manufacturing process are substantial. Improved efficiency is one of the primary advantages. By leveraging data analytics, manufacturers can identify bottlenecks, optimize production schedules, and streamline workflows. For example, by analyzing historical production data, organizations can identify patterns and optimize machine maintenance schedules, reducing downtime and maximizing productivity. Additionally, predictive analytics can help forecast demand accurately, enabling manufacturers to optimize inventory levels and reduce the risk of stockouts or overstocking.
Cost reduction is another significant benefit that data analytics brings to the manufacturing industry. By identifying inefficiencies and optimizing processes, manufacturers can reduce operational costs. For instance, data analytics can uncover energy consumption patterns, allowing organizations to optimize energy usage and reduce utility costs. Furthermore, predictive maintenance, made possible through data analytics, can help prevent equipment failures, reducing maintenance costs and minimizing unplanned downtime.
In addition to efficiency and cost reduction, data analytics enables manufacturers to enhance product quality. By analyzing production data and identifying quality patterns, organizations can proactively address issues and minimize defects. Real-time monitoring of production processes, combined with analytics, can provide early warning signals, allowing manufacturers to take corrective actions promptly. This not only improves product quality but also enhances customer satisfaction and reduces the need for costly rework or recalls.
How to Leverage Data Analytics?
Data analytics has revolutionized various industries, and manufacturing is no exception. With the increasing use of IoT devices, automation tools, and other technologies, manufacturers are generating vast amounts of data every day. This data provides valuable insights into production processes, supply chain management, product quality, and customer preferences. In this article, we will explore how manufacturers can apply data analytics to improve their operations, increase efficiency, and drive business growth.
Predictive Maintenance
Predictive maintenance is a critical application of data analytics in manufacturing, enabling organizations to identify potential equipment failures before they occur. By analyzing sensor data from machines and equipment, manufacturers can schedule maintenance during downtime, reducing the impact on production and improving overall efficiency. This approach also helps in reducing costs associated with repairs, replacement parts, and lost productivity.
Predictive maintenance leverages a combination of data elements, such as AI technology and the Internet of Things (IoT), to continuously analyze equipment conditions and predict maintenance needs. It monitors information from machines and devices to predict when and where maintenance will be needed, allowing organizations to detect abnormalities throughout machine operation and send instant alerts to the appropriate personnel.
The benefits of predictive maintenance in manufacturing are substantial, including reduced downtime, increased productivity, lower maintenance costs, improved equipment reliability, and enhanced safety for maintenance personnel. This approach also offers cost savings in the forms of improved uptime, reduced costs associated with unnecessary parts replacement, and greater efficiencies.
The implementation of predictive maintenance in manufacturing comes with its challenges, including data volume, change management, and business model obstacles. However, by overcoming these challenges and leveraging the power of predictive maintenance, organizations can maximize asset availability, optimize maintenance tasks in real time, and avoid disruption to operations.
Quality Control
Data analytics in manufacturing enables organizations to identify quality issues in real-time by analyzing production data. This process allows manufacturers to detect anomalies in the production process, such as variations in product quality or unexpected changes in equipment performance, and take corrective action quickly. By examining historical production data, manufacturers can identify patterns and trends that correlate with defects, enabling them to pinpoint specific areas of improvement and implement targeted strategies[1].
The benefits of data analytics in manufacturing for improving product quality and reducing defects are substantial. It offers more accurate demand forecasting, improved quality control, more efficient inventory management, and true end-to-end visibility[2]. Predictive analytics can enable manufacturers to anticipate potential quality issues before they manifest or become significant, allowing them to develop and implement preventative measures in a timely manner to maintain consistent product quality and reputation[3].
Supply Chain Optimization
Data analytics plays a crucial role in optimizing supply chain operations for manufacturers. By leveraging data from various sources, including inventory levels, shipment times, and supplier performance, manufacturers can identify inefficiencies and bottlenecks, leading to reduced lead times, lower costs, and improved customer satisfaction[1].
The use of data analytics in supply chain management enables organizations to achieve several benefits, such as enhanced efficiency and performance, improved decision-making, and increased visibility into operations. It also allows for the identification of areas for optimization and improvement within the supply chain[2].
Data analytics tools provide the capability to analyze vast amounts of data from multiple sources, offering insights that can be used to optimize supply chain operations. These tools enable companies to make proactive changes, such as shifting production methods and pursuing demand-based supply chains, ultimately leading to a competitive advantage[1].
In addition to optimizing supply chain operations, data analytics can be used for inventory management, demand forecasting, logistics, and overall decision-making efficiency. It also helps in mitigating risks and disruptions by providing real-time insights and enhancing transparency within the supply chain[3].
Workforce Optimization
Data analytics can significantly contribute to optimizing the workforce in manufacturing. By analyzing data on employee performance, engagement, and other workforce-related factors, organizations can make more informed decisions about managing their employees, improving overall performance, and achieving their business objectives[1]. Some key strategies of workforce optimization in people analytics include identifying key performance indicators (KPIs) for the workforce, collecting and analyzing data, developing targeted interventions, and monitoring and adjusting interventions[1].
Predictive analytics is also a game-changer for manufacturing workforce optimization, as it can identify potential skill gaps before they impact production, analyze trends, and predict future requirements, enabling proactive planning for training and development[2]. The integration of predictive analytics in workforce management leads to a holistic improvement in operational efficiency, providing a significant competitive advantage[2].
Workforce optimization (WFO) is a set of strategies and practices that aim to improve employee and organizational efficiency and decrease operational costs. WFO software combines analytics, quality management, call recording, coaching, speech analytics, text analytics, surveying, and feedback to optimize workforce processes[3]. Manufacturers can leverage workforce data to gain valuable insight into labor productivity and personnel costs at each stage of production. By analyzing this data, manufacturers can make informed decisions about staffing levels, scheduling, and training programs to improve overall efficiency and reduce costs associated with labor turnover[4].
Customer Insights
Data analytics can provide manufacturers with valuable customer insights, enabling them to understand customer preferences and behavior. By analyzing customer data, manufacturers can identify trends and patterns that inform product design, pricing strategies, and promotional activities. This helps to improve customer satisfaction and loyalty, ultimately driving business growth[1].
Customer insights are essential for manufacturers to develop targeted marketing campaigns and improve product development. By leveraging consumer data, manufacturers can gain a deeper understanding of their customers' behaviors, preferences, and needs. This allows for more personalized and tailored interactions with customers, leading to increased customer satisfaction and improved business outcomes[2].
In today's competitive retail landscape, customer insights are crucial for providing high-quality experiences and products. By harnessing the power of consumer insights, manufacturers can make more informed decisions on how to best serve their customers and win over new ones. These insights allow companies to make more informed decisions on how to best serve their customers and win over new ones[3].
Overall, customer insights obtained through data analytics are fundamental in providing valuable information to help businesses exceed customer expectations, improve product development, and drive business growth. By leveraging consumer data, manufacturers can make more informed decisions on how to best serve their customers and win over new ones[4].
Data Security and Privacy
Manufacturers must prioritize data security and privacy as they collect and analyze large amounts of data from various sources. To ensure the security and privacy of this data, manufacturers should implement robust security measures, such as encryption, access controls, and data loss prevention (DLP) solutions. Additionally, they must comply with data protection regulations, conduct regular security audits, and provide employee training on cybersecurity best practices[1][2][3].
Data security best practices in manufacturing include protecting sensitive data from insider threats, controlling removable devices, addressing sensitive data stored locally, and ensuring compliance with relevant regulations such as NIST SP 800-171 and NIST SP 800-53 in the United States[1]. Manufacturers should also focus on gaining visibility into where sensitive data resides, training employees to recognize cybersecurity threats, and using data classification to enhance compliance efforts[3].
Risks associated with data loss in the manufacturing industry include operational disruptions, supply chain issues, loss of business continuity, and potential legal and compliance outcomes[2]. Data loss can also lead to the exposure of intellectual property, reputational damage, and violations of data privacy regulations[2].
To mitigate these risks, manufacturers should implement robust data protection measures, including regular data backups, secure storage, access controls, encryption, employee training, cybersecurity measures, and disaster recovery plans[2]. By prioritizing data security and privacy, manufacturers can bolster their competitiveness, maintain customer confidence, and mitigate the risks associated with data breaches and unauthorized access[2].
Why Datasumi?
The integration of data analytics in the manufacturing industry has the potential to revolutionize operations, improve efficiency, and drive growth. By leveraging data analytics, manufacturers can realize significant benefits, including end-to-end visibility, improved quality control, more accurate demand forecasting, efficient inventory management, and true end-to-end visibility[1].
Data analytics in manufacturing enables organizations to optimize their workflows, improve product quality and consistency, and make informed decisions to drive growth[3]. It allows manufacturers to identify patterns, measure impact, predict outcomes, and gain valuable insights into their operations[4]. By analyzing data from various sources such as equipment sensors, production lines, and supply chains, manufacturers can uncover valuable insights and optimize their processes[5].
Partnering with a data and digital consultancy like Datasumi can provide manufacturers with the expertise needed to implement robust data collection mechanisms, design customized data analytics models, and develop data-driven strategies to improve efficiency[1]. Such partnerships can help manufacturers identify relevant data sources, develop data integration frameworks, deploy advanced analytics models, and provide ongoing support and maintenance to ensure the effectiveness of data analytics solutions[1].
Conclusion
In conclusion, the application of data analytics in the manufacturing process offers immense potential for improved efficiency, cost reduction, and enhanced product quality. By leveraging the power of data analytics, manufacturers can unlock valuable insights, optimize operations, and gain a competitive edge in the market. However, it is crucial for organizations to address concerns regarding data quality and security to ensure the success of their data analytics initiatives. Partnering with a data and digital consultancy like Datasumi can provide the necessary expertise and support to harness the full potential of data analytics and drive business success in the manufacturing industry.
The integration of data analytics in the manufacturing industry offers a wealth of opportunities for improving operational efficiency, product quality, and supply chain management. As companies continue to embrace Industry 4.0 and the digital transformation of manufacturing processes, data analytics will play a pivotal role in driving innovation and competitiveness within the industry.
References
"The Future of Manufacturing: Predictive Maintenance." Forbes, 26 Jan. 2022, https://www.forbes.com/sites/forbestechcouncil/2022/01/26/the-future-of-manufacturing-predictive-maintenance/?sh=54c93f0e7f6c
"How Data Analytics Can Improve Supply Chain Management." Harvard Business Review, 18 Jan. 2019, https://hbr.org/2019/01/how-data-analytics-can-improve-supply-chain-management
"The Power of Data Analytics in Manufacturing." IndustryWeek, 15 Mar. 2022, https://www.industryweek.com/manufacturing/power-data-analytics-manufacturing
"How Data Analytics Can Help Improve Workforce Productivity." Manufacturing Tomorrow, 23 June 2020, https://www.manufacturingtomorrow.com/articles/how-data-analytics-can-help-improve-workforce-productivity
"Understanding Customer Insights in Manufacturing." CMO.ai, https://cmo.ai/understanding-customer-insights-in-manufacturing
"Managing Data Security and Privacy in Manufacturing." Manufacturing Business Technology, 25 Jan. 2022, https://www.manufacturingbusinesstechnology.com/features/managing-data-security-and-privacy-in-manufacturing-1876459/
https://www.snowflake.com/trending/data-analytics-manufacturing/
https://www.insight.com/en_US/content-and-resources/2022/data-analytics-in-manufacturing.html
https://www.fogwing.io/blog/data-analytics-in-manufacturing/
https://research.aimultiple.com/manufacturing-analytics-use-cases/
https://www.weforum.org/agenda/2022/09/manufacturing-data-advanced-analytics/
https://www.snowflake.com/trending/data-analytics-manufacturing/
https://news.lineview.com/benefits-and-importance-of-data-analytics-in-manufacturing
https://www.insight.com/en_US/content-and-resources/2022/data-analytics-in-manufacturing.html
https://www.fogwing.io/blog/data-analytics-in-manufacturing/
https://www.snowflake.com/guides/using-data-for-supply-chain-optimization
https://proventainternational.com/the-impact-of-data-analytics-on-supply-chain-decision-making/
https://www.snowflake.com/guides/smarter-supply-chain-manufacturing/