Big Data Challenges and How to Overcome Them
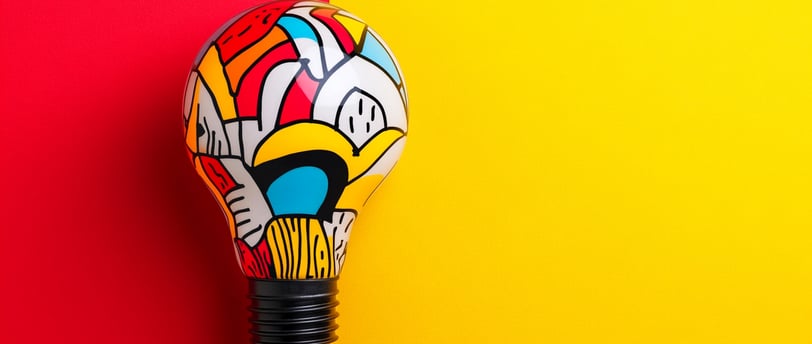
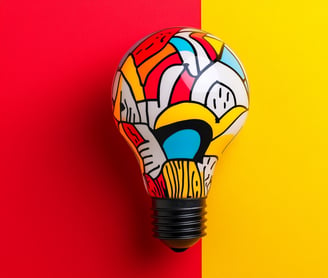
In the digital age, data has become the lifeblood of organisations, fueling decision-making processes and driving business growth. However, as data volume, variety, and velocity grow exponentially, businesses face significant challenges in harnessing their full potential. This article will explore significant data challenges and strategies to overcome them.
Understanding Big Data Challenges
Significant data challenges come in various forms, from technical hurdles to organisational and ethical concerns. The primary issues include data volume, velocity, variety, veracity, and value. Each challenge requires a tailored approach to manage and leverage big data effectively.
1. Data Volume
The sheer volume of data generated daily is staggering. Organisations deal with petabytes or even exabytes of data, making traditional storage solutions inadequate. This overwhelming amount of data can strain existing infrastructures and make it challenging to extract meaningful insights.
Organisations can implement data compression and deduplication techniques to manage data volume, reduce storage costs, and optimise available space. Additionally, leveraging cloud solutions can provide scalable and flexible storage options, allowing businesses to handle large datasets more efficiently12.
2. Data Velocity
Data is generated and collected at an unprecedented speed, requiring real-time processing and analysis. Traditional data processing methods often struggle to keep up with this velocity, leading to delays and missed opportunities.
Organisations can adopt stream processing technologies, allowing real-time data ingestion and analysis to address data velocity. Organisations can implement data integration tools and platforms that support multiple data formats to handle the variety of data. Technologies like Apache Kafka and Apache Flink enable processing data as it arrives, ensuring timely insights and quick decision-making1.
3. Data Variety
Big data encompasses a wide variety of data types, including structured data (e.g., databases), semi-structured data (e.g., XML, JSON), and unstructured data (e.g., text, images, videos). Managing and integrating these diverse data types can be challenging.
To handle data variety, organisations can implement data integration tools and platforms that support multiple data formats. Additionally, data lakes can store and manage diverse data types, providing a centralised repository for all data sources1.
4. Data Veracity
Data quality, accuracy, and reliability are crucial for deriving meaningful insights. However, big data often suffers from issues like incomplete records, errors, and duplicates, which can lead to flawed analysis and decision-making.
Organisations can use data cleansing and validation tools to maintain veracity and identify and correct data quality issues. Regular data quality assessments and governance frameworks can help ensure data remains accurate and reliable13.
Overcoming Big Data Challenges
Addressing significant data challenges requires a strategic approach that combines technological solutions with organisational practices. Here are some key strategies to overcome significant data challenges:
1. Investing in Advanced Technologies
Investing in advanced technologies like machine learning, artificial intelligence, and data visualisation tools can help organisations make sense of their data. These technologies can automate data processing, identify patterns, and provide actionable insights.
For example, machine learning algorithms can analyse large datasets to predict customer behavior, optimise supply chains, and detect fraud. Visualisation tools can present complex data in an easy-to-understand format, enabling stakeholders to make informed decisions.
2. Building a Data-Driven Culture
Fostering a data-driven culture within the organisation is essential for overcoming significant data challenges. This involves encouraging data literacy, promoting data-driven decision-making, and ensuring data accessibility to all relevant stakeholders.
Organisations can achieve this by providing employee training and development opportunities, fostering collaboration between data scientists and business units, and implementing data governance policies that ensure data quality and security.
3. Implementing Robust Data Governance
Data governance encompasses the policies, procedures, and practices that ensure data quality, security, and compliance. Implementing robust data governance frameworks can help organisations manage their data effectively and mitigate risks.
Data governance involves defining data standards, establishing quality controls, and implementing access controls to protect sensitive information. It also includes compliance with data protection regulations like the General Data Protection Regulation (GDPR) and the California Consumer Privacy Act (CCPA).