Challenges Integrating AI into Existing Cybersecurity Systems
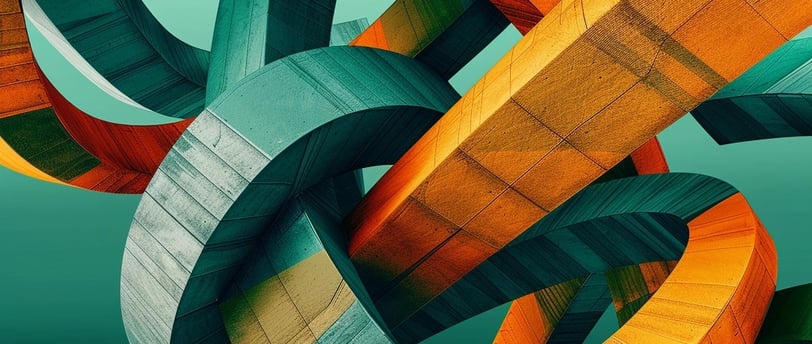
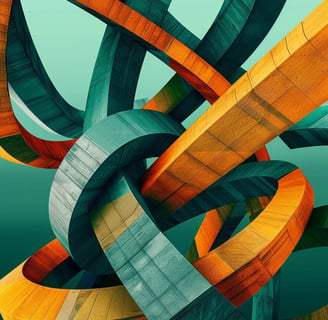
Integrating Artificial Intelligence (AI) into existing cybersecurity systems presents a significant challenge in ensuring that AI comprehends the full context of emerging threats. One of the primary difficulties AI systems encounter is the sheer volume of data they must analyze. This vast amount of data can overwhelm AI, leading to false alarms or, conversely, the oversight of critical security issues. AI's ability to parse and understand the context within this data is crucial for its effective deployment in cybersecurity.
AI systems often struggle with contextual awareness, an essential component in accurately identifying and mitigating threats. Context in cybersecurity can include a multitude of variables such as the historical behavior of network traffic, user behavior patterns, and the nature of the data itself. Without a comprehensive understanding of these variables, AI systems may misinterpret benign activities as malicious or fail to recognize sophisticated attacks. This lack of nuanced understanding can diminish the reliability of AI in cybersecurity applications.
To address these challenges, enhancing AI's contextual awareness is paramount. One effective strategy is diversifying the data sources that AI analyzes. By integrating data from various sources, such as network logs, endpoint data, and external threat intelligence feeds, AI systems can develop a more holistic view of the threat landscape. This comprehensive dataset enables AI to make more informed decisions, reducing the likelihood of false positives and negatives.
Furthermore, the implementation of machine learning techniques that focus on anomaly detection and pattern recognition can significantly improve AI's contextual understanding. These techniques allow AI to learn from past incidents, adapting its algorithms to better recognize genuine threats. Continuous refinement and training of AI models are essential to keep pace with the evolving nature of cyber threats.
In conclusion, while integrating AI into cybersecurity systems holds great promise, it is imperative to address the challenges related to contextual understanding. By enhancing AI's ability to analyze diverse data sources and employing advanced machine learning techniques, we can mitigate these challenges and unlock the full potential of AI in safeguarding our digital environments.
Complexity of AI Systems
Integrating Artificial Intelligence (AI) into existing cybersecurity frameworks presents significant technical challenges largely due to the inherent complexity of AI systems. One of the primary obstacles is the necessity for specialized knowledge to effectively manage and maintain these sophisticated systems. AI technologies often require a deep understanding of machine learning algorithms, data analytics, and neural networks, which can be daunting for cybersecurity professionals who may not possess this specific expertise.
Another critical challenge lies in ensuring interoperability with legacy systems. Many existing cybersecurity infrastructures are built on technologies that may not readily support the integration of advanced AI solutions. This can lead to compatibility issues, necessitating extensive modifications or even complete overhauls of current systems. The process of making these older systems work seamlessly with new AI technologies can be both time-consuming and resource-intensive.
Furthermore, the added complexity of AI systems can inadvertently increase system vulnerabilities. The more intricate a system becomes, the more potential entry points there are for cyber threats. AI systems, with their layers of algorithms and data processing capabilities, can create additional pathways for malicious actors to exploit. This paradoxically increases the risk profile of the cybersecurity system they are meant to enhance.
To manage and simplify these complexities, several strategies can be employed. Firstly, investing in continuous education and training for cybersecurity professionals can bridge the knowledge gap, equipping them with the necessary skills to handle AI technologies. Secondly, adopting modular AI solutions that can be incrementally integrated into existing systems can mitigate compatibility issues. Finally, implementing robust security measures specifically designed to protect AI components can reduce the risk of increased vulnerabilities. By addressing these challenges methodically, organizations can more effectively incorporate AI into their cybersecurity infrastructures, enhancing their overall defense mechanisms while managing the complexities involved.
Data Privacy and Security Concerns
Integrating Artificial Intelligence (AI) into existing cybersecurity systems introduces a range of data privacy and security concerns. One of the primary issues is the potential for data breaches. AI systems often require vast amounts of data to function effectively, and this data can include sensitive personal and corporate information. If not managed properly, this data can become a target for cybercriminals, leading to unauthorized access and significant breaches.
Another key concern is the misuse of sensitive information. AI systems have the capability to analyze large datasets, which can inadvertently lead to the exposure of confidential information. For instance, an AI system designed to detect threats might process and store sensitive data without adequate encryption, making it vulnerable to unauthorized access.
Unauthorized access is a significant risk when integrating AI into cybersecurity systems. AI models and algorithms often need to access different types of data to improve their accuracy and efficiency. This access can create potential entry points for cyber attackers if proper access controls are not implemented. Ensuring that only authorized personnel can access sensitive data is crucial to maintaining data security.
Adhering to data protection regulations is essential when incorporating AI into cybersecurity systems. Regulations such as the General Data Protection Regulation (GDPR) and the California Consumer Privacy Act (CCPA) set stringent requirements for data handling and protection. Organizations must ensure that their AI systems comply with these regulations to avoid legal repercussions and maintain user trust.
Implementing robust data security measures is vital to safeguarding against these risks. This includes encrypting data both in transit and at rest, using multi-factor authentication, and regularly updating security protocols to address new threats. Additionally, organizations should conduct regular security audits and vulnerability assessments to identify and mitigate potential weaknesses in their AI-integrated cybersecurity systems.
In conclusion, while AI offers significant benefits for cybersecurity, it also presents considerable data privacy and security challenges. Addressing these concerns through stringent data protection measures and regulatory compliance is critical to leveraging AI's full potential in enhancing cybersecurity.
Scalability Issues
One of the primary challenges in integrating Artificial Intelligence (AI) into existing cybersecurity systems is ensuring that these AI solutions are scalable. As organizations continue to generate vast amounts of data, AI systems must be capable of handling this influx efficiently. The scalability issue encompasses not only the volume of data but also the increasing complexity and sophistication of cybersecurity threats. Traditional systems often struggle to adapt to the dynamic nature of cyber threats, thus necessitating scalable AI solutions that can evolve alongside these challenges.
Ensuring that AI can manage large datasets is a formidable task. The computational resources required to process and analyze data in real-time can be significant. Moreover, as the volume of data grows, so does the need for more advanced machine learning models, which can further strain existing infrastructure. This is where cloud-based solutions come into play. By leveraging cloud computing, organizations can access scalable and flexible resources that can be adjusted based on demand. Cloud-based AI solutions provide the necessary computational power and storage, making it easier to manage and analyze large datasets efficiently.
Another approach to achieving scalable AI integration is through distributed computing. Distributed computing involves spreading computational tasks across multiple machines or servers, thereby enhancing processing power and efficiency. This method allows for the distribution of workloads, ensuring that no single system is overwhelmed by the sheer volume of data or complexity of tasks. Distributed AI systems can dynamically allocate resources where needed, ensuring that cybersecurity measures remain robust against evolving threats.
The integration of scalable AI solutions into cybersecurity frameworks also requires careful consideration of data management and interoperability. Ensuring that AI systems can seamlessly operate alongside existing cybersecurity tools is crucial. This can be achieved through standardized protocols and APIs that facilitate smooth communication and data exchange between systems. By addressing these scalability issues, organizations can enhance their cybersecurity posture, making it more resilient against the ever-growing landscape of cyber threats.
Human-AI Collaboration
Integrating AI into existing cybersecurity systems presents both opportunities and challenges, particularly in the realm of human-AI collaboration. While AI has the potential to augment human capabilities significantly, achieving seamless interaction between human experts and AI systems remains a complex task. The primary challenge lies in fostering an environment where human cybersecurity professionals can effectively collaborate with AI tools, leveraging their unique strengths to enhance overall security measures.
One of the key benefits of AI in cybersecurity is its ability to process vast amounts of data at speeds unattainable by humans. AI systems can detect anomalies, identify threats, and predict potential breaches, providing valuable insights that human experts can use to make informed decisions. However, for AI to be truly effective, it must be integrated into workflows in a manner that complements human expertise rather than replacing it. This necessitates the development of intuitive interfaces and clear communication channels between AI systems and their human counterparts.
Despite these advantages, several difficulties arise in achieving seamless human-AI interaction. One significant hurdle is the trust factor; cybersecurity professionals need to trust the AI's analysis and recommendations. Transparency in AI decision-making processes is crucial to build this trust. Additionally, AI systems must be designed to provide explanations for their actions and decisions, enabling human experts to understand and verify the AI's conclusions.
Moreover, the rapid evolution of AI technology necessitates continuous training and upskilling of cybersecurity professionals. These experts must be equipped with the knowledge and skills to work alongside AI tools effectively. This involves not only understanding how to operate and interpret AI systems but also staying abreast of the latest developments and advancements in AI-driven cybersecurity.
In conclusion, while AI has the potential to revolutionize cybersecurity, effective human-AI collaboration is essential to maximize its benefits. Overcoming the challenges of trust, seamless interaction, and continuous upskilling will be key to integrating AI into existing cybersecurity systems successfully.
Bias and Ethical Considerations
Integrating artificial intelligence (AI) into existing cybersecurity systems presents significant ethical challenges, particularly concerning biases in AI models. These biases often stem from the training data used to develop AI algorithms. If the training data is not representative of the diverse range of potential cyber threats, the AI system may produce unfair or inaccurate threat assessments. For instance, an AI system trained predominantly on data from a specific geographic region may be less effective at identifying threats originating from other parts of the world.
Bias in AI can manifest in various ways, including overfitting to particular types of attacks or underrepresenting less common threats. This can lead to significant gaps in cybersecurity defenses, potentially leaving systems vulnerable to exploitation. Moreover, biases can result in the misclassification of benign activities as malicious, leading to false positives and unnecessary resource allocation to address non-existent threats.
The ethical implications of AI in cybersecurity extend beyond biases. There is a potential for misuse of AI technologies, particularly in the creation of autonomous systems that can operate without human oversight. Such systems might inadvertently execute actions that are ethically questionable or even illegal. Ensuring that AI in cybersecurity is used responsibly requires the implementation of transparent and accountable AI practices. This includes establishing clear guidelines for AI development and deployment, regular audits of AI decisions, and maintaining human oversight to ensure ethical standards are upheld.
Transparency in AI decision-making processes is crucial for accountability. Organizations must document the rationale behind AI-driven decisions and provide stakeholders with explanations of how specific outcomes were reached. This transparency helps build trust and ensures that AI technologies are used in ways that align with ethical standards and societal values.
In summary, addressing the biases and ethical considerations in AI-driven cybersecurity systems is essential for developing fair and effective defenses. By implementing transparent and accountable practices, organizations can mitigate the risks associated with AI biases and ensure that AI technologies are used responsibly.