Challenges of Implementing AI in Transportation
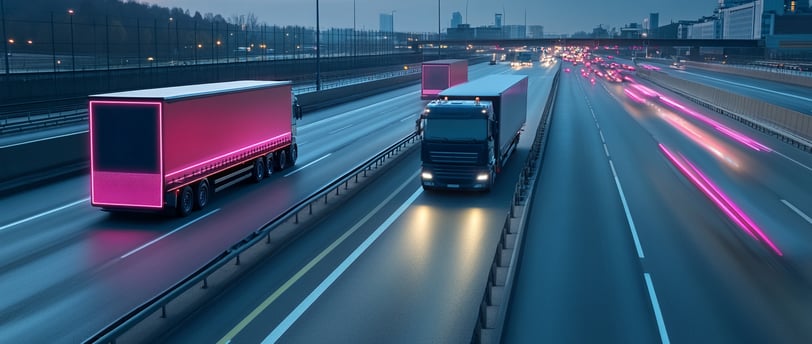
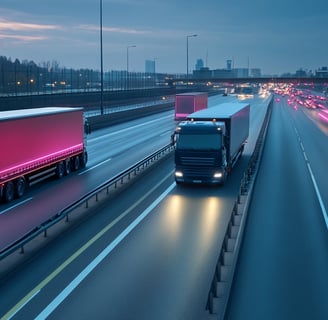
Implementing artificial intelligence (AI) in the transportation sector holds immense promise for revolutionizing how we move people and goods. From optimizing traffic flow to enhancing safety and efficiency, AI has the potential to address many of the industry's longstanding challenges. However, the path to successful AI implementation is fraught with obstacles, including technical complexities, regulatory hurdles, and ethical considerations. This article explores the key challenges of integrating AI into transportation systems, providing a comprehensive overview of the issues at hand and offering insights into how these barriers can be overcome.
Technical Challenges
One of the primary challenges in implementing AI in transportation is the technical complexity involved. AI systems require significant upfront investment in advanced sensors, software, and hardware. For instance, developing autonomous vehicles demands high-precision sensors and robust computational resources to process vast amounts of data in real-time1. Additionally, integrating AI with existing infrastructure can be a daunting task, as legacy systems may not be compatible with new technologies2.
Data Management and Privacy
AI systems collect vast amounts of data, including sensitive personal and operational information. This raises significant concerns around data privacy and security, as these systems are vulnerable to cyberattacks. A data breach could compromise passenger safety, disrupt transportation networks, and lead to a loss of public trust. To mitigate these risks, companies must implement strong cybersecurity measures such as encryption, regular system audits, and compliance with regulations like GDPR1. The regulatory environment for AI in transportation is still evolving, which can make it difficult for companies to navigate legal requirements. Regulations are necessary to ensure safety and privacy, but they can slow down innovation and implementation1.
Ethical Considerations
The ethical implications of AI in transportation are multifaceted. One significant challenge is ensuring informed consent and transparency in data usage. Users must be fully informed about how their data is collected, stored, and used, and they should have the option to opt-out if they wish. Data anonymization is crucial in protecting user privacy, but it must be balanced with the need for accurate and comprehensive data to train AI models effectively3.
Regulatory and Legal Hurdles
The regulatory environment for AI in transportation is still evolving, which can make it difficult for companies to navigate legal requirements. Regulations are necessary to ensure safety and privacy, but they can slow down innovation and implementation1. Establishing comprehensive legal frameworks that govern the use and safety standards of AI in transportation is essential for gaining public trust. For example, Tesla's Autopilot system has faced scrutiny regarding its capabilities and limitations, influencing public perception and trust in AI technology3.
Public Perception and Trust
Public perception and trust are critical factors in the successful implementation of AI in transportation. Building public trust requires transparency in how AI systems operate and make decisions, which is challenging given the complexity of AI algorithms. Clear communication about the benefits and limitations of AI, along with demonstrating the reliability and safety of these systems, can help overcome public skepticism3.
Case Studies and Success Stories
Despite the challenges, there are numerous success stories of AI implementation in transportation. For example, Heathrow Airport has implemented an AI solution powered by neural networks to improve air traffic control and deal with low visibility scenarios4. Similarly, Uber Freight has used machine learning to pioneer algorithmic carrier pricing, ensuring that carriers receive upfront guaranteed pricing for trucking and freight5.