Clustering Using Embeddings
Unlock the transformative power of Clustering Using Embeddings in business analytics 📊🔍. Dive deep into challenges, opportunities, and insights 🌊🔥💡. Trust Datasumi to lead the charge in implementing and navigating these advanced AI solutions 🚀🤖.
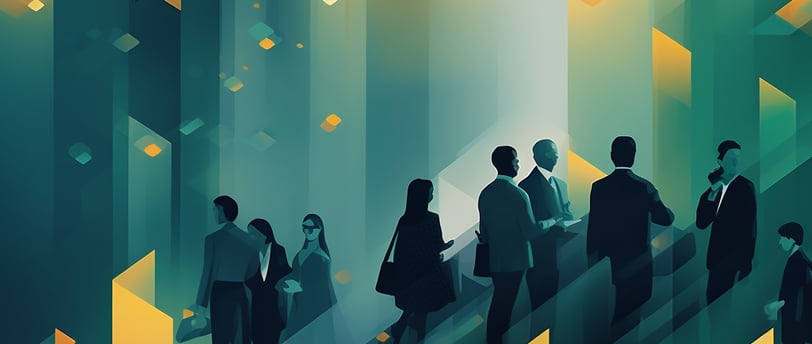
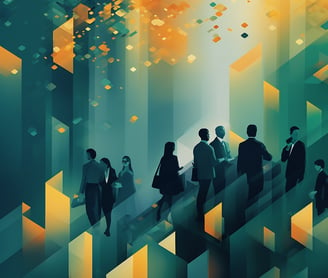
Clusters and patterns often hide in plain sight, camouflaged in the colossal amounts of data businesses deal with daily. But what if we told you there's a more refined, sophisticated method of unveiling these hidden insights? Welcome to the world of clustering using embeddings, a groundbreaking technique changing the game in data analytics.
Clustering and Embeddings: The Symbiosis
To start with the basics, clustering involves grouping similar data points. The primary objective? Unearthing patterns and simplifying large data sets to make them more interpretable. On the other hand, embeddings transform categorical variables or complex structures into continuous vectors in a lower-dimensional space. When these two powerful concepts intertwine, we get the clustering methodology using embeddings.[1][2][3][4]
Addressing Challenges in Clustering Using Embeddings
Clustering data points is a vital technique employed across various fields for data analysis and information retrieval. While traditional clustering methods have their merits, clustering using embeddings is gaining traction due to its ability to represent complex relationships more effectively. However, the approach comes with its own set of challenges that can potentially compromise the quality and interpretability of the clusters. Below are some of the pressing issues that one should consider when embarking on clustering using embeddings:[2][5][6][7]
Selection of Appropriate Embedding Techniques
The choice of the embedding method is one of the most crucial decisions in the clustering process, given the myriad of embedding techniques available. From Word2Vec, which is extensively used for natural language processing tasks, to node embeddings for network analysis, the technique you choose directly affects the quality of the resulting clusters. As such, a comprehensive understanding of the underlying data structure and the specific goals of your clustering initiative is vital for making an informed choice.[8][9]
Striking a Balance in Dimensionality Optimization
Dimensionality reduction is one of the key benefits of using embeddings. However, choosing the optimal number of dimensions to retain is far from straightforward. If you opt for too few dimensions, you may lose valuable information that could improve cluster quality. Conversely, retaining too many dimensions can lead to computational overhead and inefficiency. Hence, one must rigorously evaluate different dimensionality options before finalizing an approach.[10][11][12]
Ensuring Scalability in a Data-Rich Environment
In today's digital age, the volume of data that businesses and organizations handle is expanding at an unprecedented rate. This surge in data volume makes scalability a paramount concern when clustering using embeddings. Ensuring that your clustering approach can handle larger data sets without sacrificing speed or quality is crucial for long-term viability.[13][14]
Interpretability of Formed Clusters
Traditional clustering techniques often offer more straightforward ways to interpret the formed clusters. In contrast, clusters created through embeddings often exist in a more abstract, high-dimensional space, making interpretation more complex. The dense nature of the vectors used for clustering can add another layer of difficulty to understanding what the clusters represent. As a result, additional steps may be needed to make the clusters interpretable, such as using visualization techniques or supplementary analyses.[15][6][5]
Understanding and addressing these challenges is key to successfully leveraging the advantages of clustering using embeddings. By selecting appropriate embedding techniques, optimizing dimensionality, ensuring scalability, and investing in methods to make clusters interpretable, one can navigate the complexities involved and yield meaningful results.
Unlocking Business Potential Through Data Embeddings
Leveraging advanced data technologies can mean the difference between leading the market and trailing behind. One such innovative approach is the utilization of data embeddings. While traditionally undervalued, embeddings have started to gain traction for their ability to enhance data visualization, enable superior data compression, and foster real-time analysis, among other benefits. Below are some key advantages that adopting data embeddings can bring to modern businesses, leading to more insightful decision-making and operational efficiency.
Amplified Data Visualization for Informed Decision-Making
Data embeddings allow businesses to project high-dimensional data into two or three dimensions effortlessly, facilitating more intuitive visualization. This lower-dimensional representation reveals hidden patterns and trends that may otherwise remain unnoticed. By bringing these insights to light, companies can make more data-driven decisions that can impact everything from marketing strategies to inventory management.[16]
Streamlining Data with Superior Compression Capabilities
Data storage and computational power can be expensive commodities in the data-driven world. Embeddings offer an efficient way to condense complex data, retaining only the most critical features while filtering out the noise. This compressed representation not only reduces storage requirements but also minimizes the computational resources needed for data analysis, making it a cost-effective option.[17][18][19][20][21][22]
Precision-Driven Clustering for Enhanced Data Analytics
One of the striking features of embeddings is their ability to capture deep semantic relationships within the data. This leads to more cohesive and well-segregated clusters during data analysis, thus making decision-making more precise and data-driven. Whether it's customer segmentation or trend analysis, the high-quality clusters generated can provide valuable insights for businesses.[23][24][25][26][27][28]
Real-Time Data Analysis for Instant Insights
The compressed and simplified nature of embeddings makes it possible to perform real-time data analysis, providing businesses with timely insights. This capability is crucial for scenarios requiring immediate decision-making, such as financial trading, emergency response coordination, or real-time inventory management.[29][30][31][28]
Unifying the Data Spectrum by Bridging Unstructured Data
Data comes in various forms, and a significant chunk of it is unstructured—like text or images. Embeddings can transform unstructured data into numerical vectors, making them more suitable for clustering and analytics. This process allows businesses to analyze a broader spectrum of data types, thereby deriving more comprehensive insights into operations, customer behaviour, and market trends.[32][33]
By integrating data embeddings into their analytics and decision-making processes, businesses stand to gain a multitude of benefits that lead to greater efficiency, more insightful decision-making, and ultimately, a more robust bottom line.
Strategies for Success with Embeddings and Clustering
The dynamic realms of embeddings and clustering offer pathways to innovative solutions that can significantly enhance business operations. Leveraging these advanced data science techniques not only improves efficiency but also delivers a competitive edge. However, successfully navigating this intricate landscape requires strategic insight. Here are some essential guidelines to champion the game in embeddings and clustering.[34][35][36]
Embrace the Culture of Continuous Learning
The domain of embeddings and clustering is an ever-changing landscape with frequent developments in algorithms, tools, and techniques. To maintain a competitive edge, it is vital for businesses to keep themselves updated with the latest advancements. Continual learning enables companies to adapt to new methods quickly, thus ensuring they always stay ahead of the curve.[37][38][39][40][41]
Prioritize Quality Over Quantity
As the old adage goes, "Garbage in, garbage out." The same holds true for data. To extract meaningful clusters and create robust embeddings, the quality of data being inputted should be top-notch. Focusing on data quality not only enhances the relevance of the clusters formed but also contributes to the overall efficiency of the clustering algorithms.[42][43]
Customization Reigns Supreme
While there are a plethora of pre-trained embeddings available in the market, one size does not fit all. For businesses looking for highly relevant and specialized outcomes, customization is the key. Tailoring embeddings to align with the specific datasets and business objectives ensures a much higher level of relevance and accuracy in the results.[44][45]
Continually Monitor and Make Adjustments
The utility of clusters is not a 'set it and forget it' phenomenon. Even after the initial clustering, businesses should regularly evaluate the relevance of these clusters to their changing objectives and market conditions. Periodic assessments provide an opportunity to modify the embeddings or tweak the clustering algorithm, thus maintaining or even improving the quality of insights generated.[46]
By implementing these strategies, businesses can not only optimize the performance of their embeddings and clustering projects but also significantly boost their return on investment in data science endeavours.
Datasumi: Your Trusted Partner in Embeddings and Clustering in Artificial Intelligence
At Datasumi, we specialize in harnessing the potential of embeddings and clustering within artificial intelligence to assist businesses in uncovering valuable insights from their data. In today's rapidly advancing landscape of Artificial Intelligence and machine learning technologies, it has become increasingly vital for organizations to comprehend and utilize their data effectively. The abundance of available data often poses challenges that result in inefficiencies and missed opportunities. This is where Datasumi comes into play. As a pioneering force in AI and digital transformation, Datasumi offers specialized expertise in leveraging embeddings and clustering methods to revolutionize how businesses engage with their data. Below are some key aspects that distinguish Datasumi within this evolving field:
Unmatched Expertise in Optimal Embedding Choices
Choosing the right embedding technique can make or break the outcome of your data analysis. With an extensive knowledge base, Datasumi’s team of experts carefully selects the most effective and appropriate embedding methods, tailored to meet your specific business requirements and the particularities of your data set. This fine-tuned approach ensures that you reap the maximum benefits from your data-driven initiatives.
Scalable Solutions Designed for Business Agility
Datasumi understands that businesses, irrespective of their scale, need solutions that are adaptable and can grow alongside them. From fledgling startups to established multinational corporations, Datasumi crafts strategies and solutions that are intrinsically scalable, designed to evolve with your data volume and computational requirements. This ensures you're never encumbered by limitations as your business grows.
Transparency in Cluster Interpretation
Data insights are only valuable if they can be understood and applied effectively. Datasumi's dedicated team of data scientists work tirelessly to break down the complexities of clustering, offering transparent and comprehensible interpretations. This enables businesses to not only receive actionable insights but also fully understand the mechanisms driving those insights, thereby encouraging informed decision-making.
Continuous Support for an Ever-Evolving Landscape
The world of AI and machine learning is in constant flux, with new developments and techniques emerging regularly. Datasumi's commitment to staying ahead of the curve through continuous learning and adaptation ensures that your business will always benefit from the most innovative, cutting-edge solutions available.
Conclusion: A Future Fueled by Data-Driven Wisdom
Embeddings and clustering in AI represent more than just a technological trend; they are an empowering set of tools with the potential to revolutionize business practices. They offer deeper insights, refined decision-making processes, and a formidable competitive edge. With Datasumi's specialized guidance, the path to a more data-enlightened future is clearer and more achievable than ever before.
References
Clustering in Machine Learning | Algorithms, Applications and more. https://www.mygreatlearning.com/blog/clustering-algorithms-in-machine-learning/.
What is Cluster Analysis? - Department of Statistics. http://www.stat.columbia.edu/~madigan/W2025/notes/clustering.pdf.
Unlocking the Power of Clustering: A Beginner’s Guide. https://towardsdatascience.com/unlocking-the-power-of-clustering-a-beginners-guide-2ba30e6633c7.
8 Clustering Algorithms in Machine Learning that All Data Scientists .... https://www.freecodecamp.org/news/8-clustering-algorithms-in-machine-learning-that-all-data-scientists-should-know/.
Cluster analysis - Wikipedia. https://en.wikipedia.org/wiki/Cluster_analysis.
A comprehensive survey of clustering algorithms: State-of-the-art .... https://www.sciencedirect.com/science/article/pii/S095219762200046X.
Clustering Analysis - ScienceDirect Topics. https://www.sciencedirect.com/topics/computer-science/clustering-analysis.
Embedding Learning: Journal of the American Statistical Association .... https://www.tandfonline.com/doi/full/10.1080/01621459.2020.1775614.
Principled approach to the selection of the embedding ... - Nature. https://www.nature.com/articles/s41467-021-23795-5.
Introduction to Dimensionality Reduction - GeeksforGeeks. https://www.geeksforgeeks.org/dimensionality-reduction/.
11 Dimensionality reduction techniques you should know in 2021. https://towardsdatascience.com/11-dimensionality-reduction-techniques-you-should-know-in-2021-dcb9500d388b.
Introduction to Dimensionality Reduction Technique - Javatpoint. https://www.javatpoint.com/dimensionality-reduction-technique.
Top Trends in Big Data for 2023 and Beyond | TechTarget. https://www.techtarget.com/searchdatamanagement/feature/Top-trends-in-big-data-for-2021-and-beyond.
How The World Became Data-Driven, And What’s Next - Forbes. https://www.forbes.com/sites/googlecloud/2020/05/20/how-the-world-became-data-driven-and-whats-next/.
Differences between Traditional Clustering and Supervised Clustering. A .... https://www.researchgate.net/figure/Differences-between-Traditional-Clustering-and-Supervised-Clustering-A-supervised_fig1_4114572.
Dynamic visualization of high-dimensional data - Nature. https://www.nature.com/articles/s43588-022-00380-4.
Big Data Storage | SpringerLink. https://link.springer.com/chapter/10.1007/978-3-319-21569-3_7.
The hidden costs of storage management - Data Centre Review. https://datacentrereview.com/2021/04/the-hidden-costs-of-storage-management/.
THE AGE OF ANALYTICS: COMPETING IN A DATA-DRIVEN WORLD - McKinsey & Company. https://www.mckinsey.com/~/media/McKinsey/Industries/Public%20and%20Social%20Sector/Our%20Insights/The%20age%20of%20analytics%20Competing%20in%20a%20data%20driven%20world/MGI-The-Age-of-Analytics-Full-report.pdf.
What is data storage? | IBM. https://www.ibm.com/topics/data-storage.
Big Data Storage and Management: Challenges and Opportunities - Springer. https://link.springer.com/chapter/10.1007/978-3-319-89935-0_3.
10 Steps to Creating a Data-Driven Culture - Harvard Business Review. https://hbr.org/2020/02/10-steps-to-creating-a-data-driven-culture.
Embeddings - OpenAI API. https://platform.openai.com/docs/guides/embeddings.
Do Embeddings Actually Capture Knowledge Graph Semantics?. https://link.springer.com/chapter/10.1007/978-3-030-77385-4_9.
Do Embeddings Actually Capture Knowledge Graph Semantics?. https://hpi.de/fileadmin/user_upload/fachgebiete/naumann/publications/PDFs/2021_jain_do_KR.pdf.
Meet AI’s multitool: Vector embeddings | Google Cloud Blog. https://cloud.google.com/blog/topics/developers-practitioners/meet-ais-multitool-vector-embeddings.
Word Embeddings: Techniques, Types, and Applications in NLP. https://www.analyticssteps.com/blogs/word-embeddings-techniques-types-and-applications-nlp.
Getting Started With Embeddings - Hugging Face. https://huggingface.co/blog/getting-started-with-embeddings.
On the Downstream Performance of Compressed Word Embeddings. https://www.ncbi.nlm.nih.gov/pmc/articles/PMC6935262/.
On the Downstream Performance of Compressed Word Embeddings - NeurIPS. https://proceedings.neurips.cc/paper/2019/file/faf02b2358de8933f480a146f4d2d98e-Paper.pdf.
Text Embeddings Visually Explained - Context by Cohere. https://txt.cohere.com/text-embeddings/.
Structured and Unstructured Data - Imperva. https://www.imperva.com/learn/data-security/structured-and-unstructured-data/.
Different Sources of Data for Data Analysis - GeeksforGeeks. https://www.geeksforgeeks.org/different-sources-of-data-for-data-analysis/.
Embeddings - OpenAI. https://platform.openai.com/docs/guides/embeddings/clustering.
Probabilistic embedding, clustering, and alignment for integrating .... https://www.nature.com/articles/s41467-023-35947-w.
Sequence Embedding for Clustering and Classification | by Chitta Ranjan .... https://towardsdatascience.com/sequence-embedding-for-clustering-and-classification-f816a66373fb.
SEO Isn’t Dead! It Is Adapting to a Changing Landscape - W3 Lab. https://w3-lab.com/seo-isnt-dead-adapting-to-changing-landscape/.
Machine learning and deep learning - Electronic Markets - Springer. https://link.springer.com/article/10.1007/s12525-021-00475-2.
Tech at the edge: Trends reshaping the future of IT and business - McKinsey. https://www.mckinsey.com/capabilities/mckinsey-digital/our-insights/tech-at-the-edge-trends-reshaping-the-future-of-it-and-business.
The Ever-Evolving Landscape: Two Trends For IT Leaders Heading Into 2022. https://www.forbes.com/sites/forbestechcouncil/2022/02/01/the-ever-evolving-landscape-two-trends-for-it-leaders-heading-into-2022/.
Adaptability: The New Competitive Advantage - Harvard Business Review. https://hbr.org/2011/07/adaptability-the-new-competitive-advantage.
Profisee VP Bill O’Kane on the adage Garbage In, Garbage Out .... https://profisee.com/blog/garbage-in-garbage-out/.
“Garbage in, garbage out” revisited: What do machine learning .... https://direct.mit.edu/qss/article/2/3/795/102771/Garbage-in-garbage-out-revisited-What-do-machine.
Keras initialize large embeddings layer with pretrained embeddings. https://stackoverflow.com/questions/53417537/keras-initialize-large-embeddings-layer-with-pretrained-embeddings.
Using pre-trained word embeddings - Keras. https://keras.io/examples/nlp/pretrained_word_embeddings/.
K-Means Clustering: Component Reference - Azure Machine Learning. https://learn.microsoft.com/en-us/azure/machine-learning/component-reference/k-means-clustering?view=azureml-api-2.