Creating Custom Generative Models
Discover the art of crafting bespoke generative models in AI, and how it can benefit your business. Furthermore, gain insights into how Datasumi can assist you in overcoming intricate digital complexities.
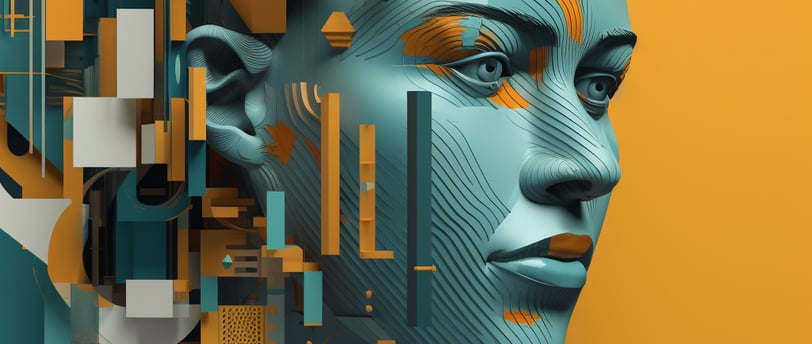
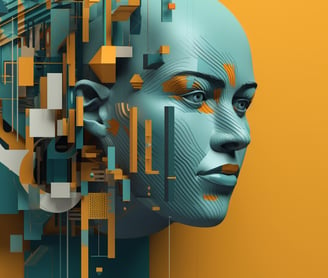
Generative models are like the wizards of the artificial intelligence domain, conjuring new data instances out of thin air—or, more accurately, from patterns and structures in existing data. While not new, these models are gaining momentum as businesses recognize their transformative potential in myriad sectors, from design and content generation to fraud detection. Creating custom generative models tailored to the specific needs of an industry or a problem stands at the frontier of this evolution. This article deeply delves into the universe of custom generative models, the challenges that abound, the unparalleled advantages they offer to businesses, and the guiding hand of Datasumi in this intricate journey.
The Magic Behind Generative Models
In the world of machine learning and artificial intelligence, the concept of generative models stands as a tour de force, capturing the attention of both experts and novices alike. While many models work to identify and classify existing data, generative models go a step further—by recreating the data itself. To appreciate the potential and versatility of these models, it is crucial to delve into their fundamental characteristics and operational mechanisms.
At the heart of any generative model is an intrinsic understanding of how data is formed. Unlike their discriminative counterparts, which focus on distinguishing among various categories of data, generative models seek to emulate the process that originally generated the data. They accomplish this by modeling the underlying statistical distribution that represents the data. The result is the capability to spawn new data points that are virtually indistinguishable from the original set. To draw an analogy, imagine a chef who not only can identify the ingredients in a complex dish but can also replicate the dish flawlessly, knowing its 'culinary DNA.'
Some of the most prevalent generative models in current practice are Generative Adversarial Networks (GANs), Variational Autoencoders (VAEs), and Restricted Boltzmann Machines (RBMs). Each of these models brings unique strengths to the table and is suited to particular types of applications. GANs, for example, excel at generating highly realistic images and are frequently employed in tasks such as image synthesis and style transfer. VAEs, on the other hand, are particularly adept at learning latent representations in an unsupervised manner, making them ideal for tasks like anomaly detection. Lastly, RBMs are often used in recommendation systems and feature extraction processes, owing to their ability to identify and recreate complex, non-linear relationships between variables.
Generative models serve as a cornerstone in the modern landscape of machine learning technologies. By understanding how data is created and by simulating that creation process, these models open up new avenues for research, application, and innovation. Whether it's GANs generating lifelike digital art or VAEs uncovering hidden structures in datasets, the magic behind generative models continues to fascinate and expand the boundaries of what is computationally possible.
Exploring the Challenges and Considerations in Custom Generative Modeling
The burgeoning field of custom generative models holds immense potential for a variety of applications, from simulating realistic data to creating advanced content. However, like any evolving technology, it comes with its own set of challenges that businesses and developers must navigate carefully. These challenges range from ensuring high data quality to ethical considerations and the computational expenses involved. Here's an in-depth look at some of the most pressing issues that stakeholders should consider when diving into the world of custom generative models.
The Crucial Role of Data Quality
At the heart of any generative model lies the data that trains it. The old adage, "garbage in, garbage out," gains an elevated level of importance in this context. The quality of data being fed into generative models dictates the accuracy and reliability of the outputs. Therefore, it is imperative for businesses to not only source high-quality, reliable data but also to preprocess it effectively to eliminate any noise or inconsistencies.
The Risk of Overfitting
Overfitting is a well-known obstacle in machine learning, and it is particularly relevant in the context of custom generative models. These models are often tailored to solve specific tasks or problems, which makes them susceptible to becoming too specialized to the training data. As a result, they can fail to generalize to new, unseen data. Balancing the model's specificity and adaptability is a delicate act that developers need to get right.
Ethical Implications to Consider
Custom generative models have opened a Pandora’s box of ethical concerns. The technology's ability to generate deepfakes or content that closely mimics reality can have severe implications, including misinformation and identity theft. This raises questions about the ethical responsibilities that businesses and developers carry when employing these models. Stringent guidelines and use-case restrictions may need to be implemented to safeguard against ethical pitfalls.
Computational Costs and Resource Requirements
Generative models, especially Generative Adversarial Networks (GANs), are notoriously resource-intensive. Training and running these models require substantial computational power, often involving the use of specialized hardware like GPUs. This creates a barrier for small to medium-sized businesses that may lack the necessary computational resources. Companies must evaluate the cost-to-benefit ratio and determine whether the investment in additional hardware and energy consumption is justified by the expected outcomes.
By addressing these challenges head-on, businesses can harness the full potential of custom generative models while minimizing risks and optimizing returns. The field is evolving rapidly, and those who can adeptly navigate these hurdles will be best positioned to reap the benefits.
Exploring the Transformative Power of Custom Generative Models in Various Industries
The adoption of artificial intelligence (AI) and machine learning (ML) technologies is increasingly shaping the competitive landscape across a myriad of industries. Among the cutting-edge technologies within this realm, custom generative models stand out for their versatility and ability to create value across multiple sectors. These models can generate new, unique data from an initial set of variables, thus paving the way for numerous innovative applications. From content creation to simulation and personalized user experiences, the potential advantages for businesses are manifold. Here's a more detailed look at how different industries can benefit from implementing custom generative models.
Unleashing Creative Potential through Content Creation
Companies operating in the fields of entertainment and design can harness the power of custom generative models to create unparalleled and unique content. Whether it's generating captivating graphics, composing novel musical pieces, or authoring engaging written material, these models serve as versatile tools that can amplify the creative process. By automating part of the content generation process, businesses can not only accelerate their production timelines but also discover innovative styles and formats.
Addressing Data Scarcity through Data Augmentation
In industries where acquiring sufficient data is either too costly or logistically challenging, custom generative models offer a promising solution. These models can generate supplementary data that can be crucial for training other machine learning models. For example, in the medical sector, generative models can produce synthetic data that mimics the properties of real patient data, thus expanding the dataset available for training diagnostic algorithms.
Accelerating Product Development with Simulation and Testing
Industries with stringent safety requirements, such as automotive and aerospace, stand to gain significantly from the simulation capabilities of custom generative models. These models can create realistic simulations for a range of testing scenarios, including aerodynamic performance or crash resistance. The benefit here is twofold: it saves both time and resources that would otherwise be spent on physical testing, while also allowing for more exhaustive and intricate virtual assessments.
Crafting Personalized User Experiences
Businesses in the e-commerce and entertainment sectors can employ custom generative models to enhance user engagement and satisfaction. By analyzing existing user data, these models can tailor content, recommendations, or even user interfaces to individual preferences and behaviors. This level of personalization not only heightens user satisfaction but can also contribute to increased retention rates and, ultimately, higher revenue.
The versatility and applicability of custom generative models make them an invaluable asset for businesses seeking to innovate and maintain a competitive edge. Through creative content generation, data augmentation, realistic simulation, and the crafting of personalized experiences, these models offer a spectrum of opportunities for value creation across diverse sectors.
Harnessing the Power of Generative Models & Achieving Business Success
Navigating the intricate landscape of generative models can be a daunting task, but it’s one that offers immense rewards for those willing to put in the effort. The utilization of Artificial Intelligence (AI), including generative models, has become a crucial determinant of success in various industries. To harness this potential, businesses must adopt strategies that are grounded in expertise, ethics, and adaptability. Below are some key insights for leveraging generative models to achieve enduring success.[1][2][3]
The Importance of Strategic Collaboration
To unlock the full potential of generative models, it is imperative to engage with industry experts who have a deep understanding of AI and its applications. Partnering with specialized firms like Datasumi, which boasts a proven track record in AI and digital solutions, allows your business to benefit from invaluable insights and high-level technical expertise. These collaborations can not only help in tailor-fitting solutions to your unique business needs but also in accelerating the realization of your business objectives.[4][5][1][6][7]
Maintain an Ethical Compass
As generative models gain traction, it's increasingly crucial to establish and adhere to a clear ethical framework. The potential misuse of these models is an ongoing concern that can have far-reaching implications. Whether it's the creation of deepfakes or the dissemination of misinformation, the ethical dimensions of AI should not be overlooked. By staying vigilant and maintaining a robust ethical stance, businesses can mitigate risks and operate with integrity.[8][2][9][1][10]
Commit to Continued Learning
The AI landscape is ever-evolving, with advancements happening at breakneck speed. To stay competitive, it's essential that your teams receive regular training and stay updated on the latest breakthroughs and best practices in the domain. Ongoing training not only enhances proficiency but also encourages a culture of continuous learning and improvement. This ensures that your organization remains at the forefront of technological advancements.[11][12][13][14]
A Realistic Approach to Limitations
While generative models have an impressive range of capabilities, it's essential to understand that they are not a one-size-fits-all solution to every problem. Knowing their limitations allows for more realistic goal-setting and more effective deployment. Whether it's issues related to data quality, computational requirements, or interpretability, a nuanced understanding helps in making informed decisions and optimizing returns.[15][16][17][18][19]
By incorporating these insights into your strategic planning, you can pave the way for a more effective and ethical application of generative models. From making informed collaborations to investing in employee training and ethical governance, these pointers serve as a comprehensive roadmap for businesses looking to exploit and generative models.
Datasumi: Navigating the Complex World of Custom Generative Models
Datasumi stands as a beacon for businesses venturing into custom generative models. Their vast experience and expertise in AI and digital solutions make them recognize the intricacies of designing models tailored to specific needs. They assist in model creation and provide comprehensive guidance in data preprocessing, ensuring optimal performance.[20][1][16]
Moreover, Datasumi places a strong emphasis on ethical AI practices. They help businesses navigate potential pitfalls, ensuring that the power of generative models is harnessed responsibly.[21][22] In a landscape that's both promising and fraught with challenges, having a trusted partner like Datasumi can make all the difference. Their guidance can pave the way for not just the success of the AI domain.
Conclusion
Custom generative models are carving out an exciting space in the AI realm. Their potential applications span a gamut of industries and offer unprecedented advantages. However, as with all powerful tools, they come with challenges. By recognizing these challenges, staying updated with the latest in the field, and forging partnerships with experts like Datasumi, businesses can harness the transformative power of these models, driving innovation and growth in a responsible and ethically grounded manner.
References
Generative AI: What Is It, Tools, Models, Applications and Use Cases. https://www.gartner.com/en/topics/generative-ai.
What is ChatGPT, DALL-E, and generative AI? | McKinsey. https://www.mckinsey.com/featured-insights/mckinsey-explainers/what-is-generative-ai.
How to Make Generative AI Greener - Harvard Business Review. https://hbr.org/2023/07/how-to-make-generative-ai-greener.
Economic potential of generative AI | McKinsey - McKinsey & Company. https://www.mckinsey.com/capabilities/mckinsey-digital/our-insights/the-economic-potential-of-generative-ai-the-next-productivity-frontier.
Generative AI - Accenture. https://www.accenture.com/us-en/insights/generative-ai.
Generative AI models – the risks and potential rewards in business - KPMG. https://kpmg.com/xx/en/home/insights/2023/04/generative-ai-models-the-risks-and-potential-rewards-in-business.html.
Unlocking The Power Of Generative AI: Exploring Business Models And .... https://www.forbes.com/sites/forbesbusinesscouncil/2023/05/03/unlocking-the-power-of-generative-ai-exploring-business-models-and-dispelling-fears/.
How Generative AI Is Changing Creative Work - Harvard Business Review. https://hbr.org/2022/11/how-generative-ai-is-changing-creative-work.
Why open-source generative AI models are an ethical way ... - Nature. https://www.nature.com/articles/d41586-023-01295-4.
Managing the Risks of Generative AI - Harvard Business Review. https://hbr.org/2023/06/managing-the-risks-of-generative-ai.
Keeping Up With A Rapidly Changing Technology Landscape. https://nuvalence.io/insights/keeping-up-with-a-rapidly-changing-technology-landscape/.
The Ever-Evolving Landscape: Two Trends For IT Leaders Heading ... - Forbes. https://www.forbes.com/sites/forbestechcouncil/2022/02/01/the-ever-evolving-landscape-two-trends-for-it-leaders-heading-into-2022/.
Generative AI Landscape: Current and Future Trends | eWEEK. https://www.eweek.com/artificial-intelligence/generative-ai-landscape/.
Artificial intelligence is evolving all by itself - AAAS. https://www.science.org/content/article/artificial-intelligence-evolving-all-itself.
Four essential questions for boards to ask about generative AI - McKinsey. https://www.mckinsey.com/capabilities/quantumblack/our-insights/four-essential-questions-for-boards-to-ask-about-generative-ai.
What is generative AI, what are foundation models, and why do they .... https://www.ibm.com/blog/what-is-generative-ai-what-are-foundation-models-and-why-do-they-matter/.
LLMOps: The Future of MLOps for Generative AI - Fiddler AI. https://www.fiddler.ai/blog/llmops-the-future-of-mlops-for-generative-ai.
Generative AI for Enterprises - Deloitte US. https://www2.deloitte.com/us/en/pages/consulting/articles/generative-ai-for-enterprises.html.
Generative models - OpenAI. https://openai.com/research/generative-models.
Data Science Consultancies: Leveraging Data for Strategic Decision .... https://www.datasumi.com/data-science-consultancies-leveraging-data-for-strategic-decision-making.
AI Ethics | IBM. https://www.ibm.com/topics/ai-ethics.
From Principles to Practice: Putting AI Ethics into Action. https://www.mckinsey.com/featured-insights/in-the-balance/from-principles-to-practice-putting-ai-ethics-into-action.