Data Engineers Ensuring Data Privacy and Security
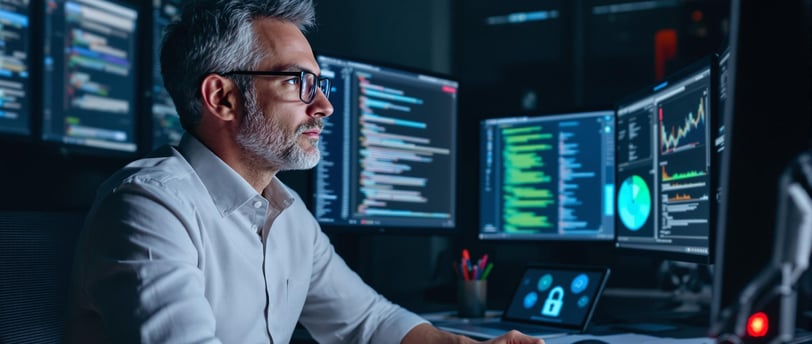
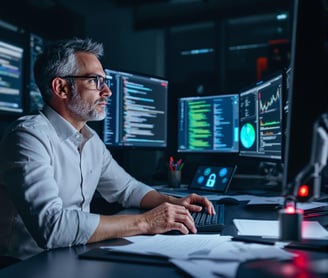
From customer insights to operational efficiencies, data drives decision-making and innovation. However, with great data comes great responsibility. Data governance is the practice of managing data as a valuable asset, ensuring its quality, security, and compliance with regulations. This comprehensive guide will explore the critical aspects of data governance, its business benefits, key elements, and best practices. We'll delve into the roles and responsibilities involved, the difference between data management and data governance, and provide real-world examples to illustrate these concepts. By the end of this article, you'll have a clear understanding of why data governance is essential and how to implement it effectively in your organization.
What is Data Governance?
Data governance is a collection of processes, roles, policies, standards, and metrics that ensure the effective and efficient use of information, enabling an organization to achieve its goals. It's about treating data as a strategic asset, ensuring it is accurate, available, consistent, and secure. Data governance provides a framework for managing data throughout its lifecycle, from creation to disposal.
The Importance of Data Governance
In an era where data breaches and privacy concerns are rampant, data governance is more important than ever. Here are a few reasons why:
Data Quality: Ensures that data is accurate, complete, and reliable. Poor data quality can lead to incorrect decisions, financial losses, and reputational damage.
Compliance: Helps organizations meet regulatory requirements such as GDPR, CCPA, and HIPAA. Non-compliance can result in hefty fines and legal consequences.
Security: Protects sensitive data from unauthorized access, breaches, and cyber-attacks. Data governance includes implementing security measures such as encryption, access controls, and monitoring.
Decision-Making: Provides a solid foundation for data-driven decision-making. With reliable data, organizations can make informed decisions that drive business growth and innovation.
Key Elements of Data Governance
Data governance encompasses several key elements that work together to ensure effective data management. Let's explore each of these elements in detail.
Data Cataloging
Effective data governance begins with knowing what data exists within an organization. A data catalog acts as a searchable index of all the data available, providing information about its format, structure, location, and usage. It adds semantic value to an otherwise unidentifiable sea of information. Think of it as a library catalog that helps users find the books they need.
Benefits: Enhances data discovery, improves data accessibility, and promotes data reuse. It saves time and resources by preventing data duplication and redundancy.
Example: A retail company uses a data catalog to manage its customer, sales, and inventory data. The catalog helps data analysts quickly find the data they need for reporting and analysis.
Data Quality
Ensuring high data quality is crucial for accurate analytics, informed decision-making, and cost-effectiveness. Data quality directly impacts the reliability of data-driven decisions. Maintaining effective data governance requires prioritizing the evaluation of key data quality attributes.
Attributes: Accuracy, completeness, freshness, and compliance with data-quality rules. For instance, ensuring that customer contact information is up-to-date and accurate.
Tools: Data profiling tools, data cleansing software, and data quality dashboards help monitor and improve data quality.
Data Classification
Data classification involves organizing and categorizing data based on its sensitivity, value, and criticality. It's a crucial part of data governance that enhances data protection and reduces risks.
Categories: Public, internal, confidential, and restricted. Each category has specific handling and protection requirements.
Example: A healthcare organization classifies patient data as confidential, ensuring it is protected with encryption and access controls.
Data Lineage
Data lineage provides a better understanding of data sources and consumption. It captures relevant metadata and events throughout the data’s lifecycle, offering an end-to-end view of how data flows across an organization’s data estate.
Benefits: Enhances data traceability, improves data quality, and supports compliance and auditing.
Example: A financial institution uses data lineage to track the flow of transaction data from source systems to reporting dashboards, ensuring data accuracy and compliance.
Data Security
Data security is a critical aspect of data governance that protects data from unauthorized access, breaches, and cyber-attacks. It involves implementing security measures such as encryption, access controls, and monitoring.
Measures: Encryption at rest and in transit, role-based access controls, and continuous monitoring and auditing.
Example: An e-commerce company implements encryption to protect customer credit card information, ensuring compliance with PCI DSS standards.
Data Auditing
Data auditing involves reviewing and examining data management processes, policies, and controls to ensure they are effective and compliant. It helps identify gaps and areas for improvement in data governance.
Benefits: Enhances compliance, improves data quality, and supports continuous improvement.
Example: A manufacturing company conducts regular data audits to ensure its data management practices comply with industry regulations and standards.
Who Oversees Data Governance?
Data governance is a collaborative effort involving various roles and responsibilities. Here are the key players:
Chief Data Officer (CDO): The most senior executive responsible for data security, accessibility, and usability. The CDO sets the vision and strategy for data governance.
Data Owners: Individuals or teams responsible for the technical administration of data sets. They make decisions on data access and usage.
Data Stewards: Responsible for implementing data governance programs and ensuring data is managed appropriately. They monitor compliance and escalate issues if they arise.
Data Governance Committees: Create relevant policies, resolve disputes, and oversee data governance initiatives.
Data Management vs. Data Governance
While data management and data governance are closely related, they serve different purposes. Data management focuses on the technical aspects of data lifecycle management, including data ingestion, integration, organization, transformation, and persistence. In contrast, data governance defines organizational policies, frameworks, and tools to ensure that data-related requirements are aligned with the business strategy.
Data Management: Deals with the operational aspects of delivering on the data strategy. It includes data architecture, data modeling, and database administration.
Data Governance: Involves defining data ownership, roles, and responsibilities, and enforcing policies and procedures throughout the organization. It includes data quality, security, privacy, auditing, and risk management.
What Does a Good Data Governance Solution Look Like?
A good data governance solution should include the following components:
Centralized Data Catalog: A searchable index of all data assets, providing information about data format, structure, location, and usage.
Unified Data Access Controls: Role-based access controls that ensure only authorized personnel can access sensitive data.
Data Auditing: Tools and processes for reviewing and examining data management practices to ensure compliance and effectiveness.
Data Quality Management: Tools and processes for monitoring and improving data quality, including data profiling, cleansing, and validation.
Data Lineage: Tools and processes for tracking data flow across the organization, enhancing data traceability and supporting compliance.
Data Discovery: Tools and processes for making data easily discoverable for analytics, AI, or ML use cases, promoting data reuse and innovation.
Data Sharing and Collaboration: Tools and processes for securely sharing data within and outside the organization, fostering collaboration and innovation.
Data Cleanrooms: Secure environments for privacy-safe collaboration, allowing organizations to share data without compromising privacy or security.
Real-World Examples of Data Governance
Let's look at some real-world examples of data governance in action:
Financial Services: A bank implements a data governance framework to comply with regulatory requirements such as Basel III and GDPR. The framework includes data classification, access controls, and regular audits.
Healthcare: A hospital uses data governance to protect patient data and ensure compliance with HIPAA. The governance program includes data encryption, access controls, and monitoring.
Retail: A retail company employs data governance to improve data quality and enhance customer insights. The program includes data profiling, cleansing, and a centralized data catalog.