Exploring Generative AI in Software Development
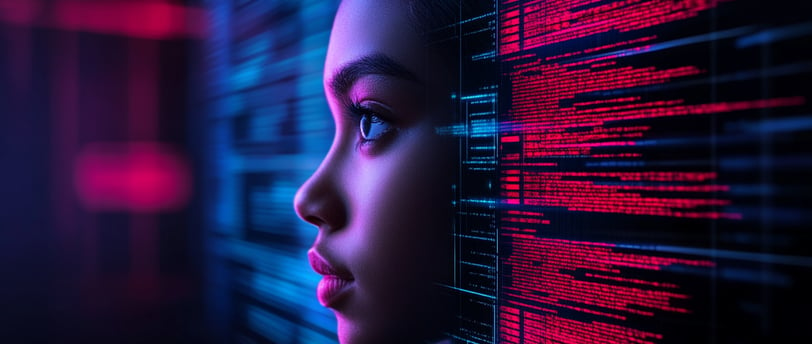
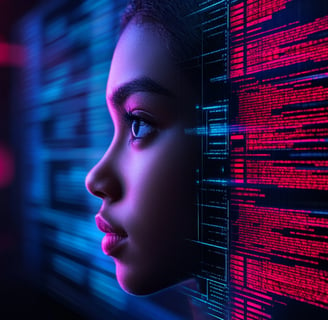
Imagine a world where software development is no longer constrained by the limitations of human coding speed and accuracy. Welcome to the era of generative AI, where artificial intelligence is redefining the landscape of software development by automating coding tasks, enhancing debugging, generating comprehensive documentation, and improving quality assurance processes. This transformative technology is not only boosting productivity but also making software development more accessible to a broader audience. In this comprehensive guide, we will explore the multifaceted impact of generative AI on software development, from code writing and debugging to documentation and quality assurance.
Code Writing and Generation
The Revolution in Software Development
Generative AI has ushered in a new era of software development by enabling the automatic generation of code snippets from natural language descriptions. This advancement brings several significant benefits that are reshaping how developers and non-developers approach coding tasks.
One of the primary advantages of generative AI in code writing is its ability to accelerate the development process. By converting human-readable instructions into executable code, these tools can drastically reduce the time developers spend on repetitive coding tasks. This efficiency allows developers to focus on more complex aspects of software development, fostering innovation and speeding up project timelines. According to a study by GitHub, developers who used generative AI tools like GitHub Copilot coded up to 55% faster than those who didn't, highlighting the significant productivity gains offered by these tools 1.
Another critical benefit is the reduction of human error. Manual coding is susceptible to mistakes that can lead to bugs and system vulnerabilities. Generative AI tools, by contrast, produce code based on predefined rules and extensive datasets. This approach minimizes the risk of errors and enhances the overall quality and reliability of the software. For instance, tools like OpenAI's Codex can suggest lines of code or entire functions based on natural language descriptions, ensuring that the generated code is contextually accurate and free from known security vulnerabilities 2.
Moreover, generative AI democratizes coding by enabling non-developers to participate in software creation. With AI-powered code generation tools, individuals without formal programming training can contribute to development projects. This inclusivity not only expands the pool of potential contributors but also encourages collaborative innovation across diverse teams. For example, GitHub Copilot allows users to generate code snippets directly within their Integrated Development Environment (IDE) from natural language prompts, making it easier for non-developers to contribute to coding tasks 1.
Real-World Applications and Tools
Several popular AI tools and platforms are leading the charge in code generation. GitHub Copilot, powered by OpenAI models, is one such tool that allows users to generate code snippets directly within their IDE from natural language prompts. Similarly, tools like TabNine and Kite leverage machine learning to suggest code completions and generate boilerplate code, significantly enhancing developer productivity. Companies are using these tools to automate routine coding tasks, such as writing unit tests, generating API documentation, and creating scaffolding for new projects. This automation not only saves time but also ensures consistency and adherence to best practices across the codebase 3.
Case Studies and Success Stories
Real-world use cases of generative AI in code writing are abundant. Companies are using these tools to automate routine coding tasks, such as writing unit tests, generating API documentation, and creating scaffolding for new projects. This automation not only saves time but also ensures consistency and adherence to best practices across the codebase. For example, a study presented at the 2021 International Conference on Intelligent User Interfaces found that generative AI provided developers with a skeletal framework to translate legacy source code into Python, making it easier to assess and fix mistakes than manually translating the source code from scratch 4.
Debugging and Error Detection
Transforming the Debugging Landscape
Generative AI is revolutionizing the landscape of debugging and error detection in software development. Traditionally, debugging has been a time-consuming and complex process, often requiring extensive manual intervention to identify and resolve code errors. However, advancements in AI algorithms are transforming this critical aspect of software engineering.
AI-powered debugging tools leverage machine learning models and natural language processing to detect anomalies and errors within the codebase. These tools can analyze vast amounts of code and identify patterns that are indicative of bugs or potential issues. By doing so, they significantly reduce the time and effort required for manual debugging. Moreover, AI algorithms can suggest potential fixes, providing developers with actionable insights to correct errors efficiently.
Several AI tools have emerged as frontrunners in the realm of debugging and error detection. For instance, DeepCode uses machine learning to analyze code repositories and provide real-time feedback on potential issues. Similarly, Microsoft’s IntelliCode leverages AI to offer intelligent code recommendations and error detection, enhancing the overall coding experience. These tools are built on sophisticated technologies such as neural networks and deep learning algorithms, which enable them to learn from vast datasets and continually improve their accuracy 3.
Enhancing Efficiency and Reliability
The integration of generative AI in debugging offers numerous advantages. One of the primary benefits is the reduction in debugging time. AI-driven tools can rapidly scan and analyze code, identifying issues that might take human developers hours or even days to detect. This accelerated error detection not only speeds up the development process but also allows developers to focus on more complex and creative aspects of coding.
Additionally, AI-powered debugging increases the reliability of software. By identifying and fixing errors early in the development cycle, these tools help ensure that the final product is robust and free from critical bugs. This leads to higher-quality software and a better user experience. For example, Gartner predicts that 80% of software engineers will have to reskill to fit into new roles created when generative AI takes over more programming functions, highlighting the significant impact of AI on the debugging process 5.
Case Studies and Success Stories
The application of generative AI in debugging and error detection is a game-changer for software development. By harnessing the power of advanced AI algorithms, developers can achieve greater efficiency, reliability, and accuracy in their coding endeavors. For instance, Caylent, an AWS cloud consulting partner, uses AI to write most of its code in specific cases, highlighting the potential of AI in automating debugging tasks 5.
Generating Documentation
Streamlining the Documentation Process
Generative AI has significantly reshaped the landscape of software development, particularly in the realm of documentation. Traditionally, creating comprehensive and accurate documentation has been a labor-intensive task, often neglected due to time constraints or the sheer volume of work required. However, with the advent of generative AI, documentation can be automatically generated from code, streamlining the process and enhancing overall project efficiency.
AI-driven documentation tools analyze the codebase to produce detailed and precise documentation, ensuring that every aspect of the project is thoroughly covered. These tools, such as OpenAI Codex and GitHub Copilot, utilize advanced natural language processing algorithms to interpret code and translate it into human-readable documentation. This not only saves developers a substantial amount of time but also ensures that the documentation is consistently up-to-date with the latest code changes 3.
Enhancing Project Management and Collaboration
The benefits of AI-generated documentation extend beyond time savings. One of the key advantages is the improvement in project management. With accurate and comprehensive documentation readily available, project managers can better understand the current state of the project, identify potential issues, and allocate resources more effectively. This leads to more informed decision-making and smoother project execution.
Moreover, generative AI fosters better team collaboration. By providing clear and precise documentation, team members can quickly get up to speed on different parts of the codebase, reducing the learning curve for new developers and facilitating more efficient knowledge transfer. This is particularly beneficial for distributed teams, where communication barriers can often impede collaboration. For example, Intuit used its generative AI tools to improve standardization of code and documentation for product development teams across personas, highlighting the potential of AI in enhancing collaboration 6.
Case Studies and Success Stories
The integration of generative AI in the documentation process represents a significant advancement in software development. By automating the creation of accurate and comprehensive documentation, AI not only alleviates the burden on developers but also enhances project management and team collaboration. As AI technology continues to evolve, we can expect even more sophisticated tools that will further streamline the documentation process and contribute to the overall success of software projects. For instance, PwC's firm-wide GenAI deployment has included software development, with gratifying results achieved in areas such as generating product features automatically and fast, accurate user stories, highlighting the potential of AI in automating documentation tasks 7.
Enhancing Quality Assurance Processes
Automating Testing Procedures
Quality assurance (QA) is a cornerstone of effective software development, ensuring that products meet predefined standards and function as intended. With the advent of generative AI, QA processes have seen significant enhancements, transforming how testing procedures are conducted and how potential issues are identified. AI-driven QA tools are at the forefront of this revolution, offering automation capabilities that streamline and elevate the entire QA lifecycle.
One of the primary advantages of incorporating generative AI into QA is the automation of testing procedures. Traditional manual testing is often time-consuming and prone to human error. AI tools, however, can execute repetitive tests swiftly and with higher accuracy. For instance, AI-powered test automation frameworks can generate test cases, simulate user interactions, and validate outcomes without manual intervention. This not only accelerates the testing phase but also ensures comprehensive coverage, as AI can systematically explore various test scenarios that might be overlooked by human testers. Additionally, AI-driven QA tools are adept at identifying potential issues before they escalate into significant problems. By leveraging machine learning algorithms, these tools can analyze vast amounts of data to detect patterns and anomalies indicative of bugs or performance bottlenecks. Predictive analytics further enhance this capability by forecasting potential areas of concern based on historical data, allowing developers to proactively address issues. Tools like Selenium, Appium, and TestComplete are some examples where AI-driven features enhance defect detection and resolution processes 3.
Ensuring High Standards of Quality
The impact of generative AI on QA extends beyond mere automation and issue detection. It also plays a crucial role in ensuring that software meets high standards of quality. AI tools can continuously monitor software performance, providing real-time feedback and insights. This continuous integration and continuous delivery (CI/CD) pipeline integration ensure that software remains robust and reliable throughout its lifecycle. Not only does this lead to more stable and high-performing applications, but it also fosters a culture of continuous improvement within development teams. For example, a full 94% of respondents in a survey by MIT Technology Review say they’re using generative AI for software development in some capacity, highlighting the widespread adoption of AI in QA processes 8.
Case Studies and Success Stories
In conclusion, the integration of generative AI in QA processes represents a paradigm shift in software development. By automating testing procedures, identifying potential issues early, and ensuring adherence to quality standards, AI-driven QA tools significantly enhance the overall development lifecycle, leading to more reliable and efficient software solutions. For instance, the introduction of generative AI coding assistants has raised expectations of improving the efficiency of software development, with engineering organizations that are using such tools seeing efficiency improvements of about 10% to 15% on average, highlighting the significant impact of AI on QA processes 6.
Democratizing Software Development
Making Software Development Accessible
Generative AI is revolutionizing the landscape of software development by making it more accessible to a wider audience, including individuals without formal coding expertise. Through the use of natural language processing (NLP) and machine learning, generative AI platforms enable users to create software applications by simply describing their requirements in everyday language. This accessibility is opening new doors for innovation and creativity, allowing a more diverse group of people to contribute to the tech industry.
The implications of this shift are profound. By lowering the barriers to entry, generative AI is fostering inclusivity within the tech sector. People from various backgrounds, including those traditionally underrepresented in technology, now have the opportunity to bring their unique perspectives and ideas to life. This democratization of software development is not only enriching the pool of innovation but also ensuring that technology solutions are more reflective of the diverse needs of society. For example, PwC's GenAI deployment has achieved gratifying results in areas such as generating product features automatically and fast, accurate user stories, highlighting the potential of AI in making software development more accessible 7.
Accelerating Technological Advancement
Moreover, the broader participation facilitated by generative AI is likely to accelerate the pace of technological advancement. With more individuals able to experiment and iterate on software projects, we can expect a surge in novel applications and solutions. This dynamic environment promotes a culture of continuous improvement and rapid prototyping, which is essential for addressing complex, real-world problems in innovative ways.
Future Trends and Innovations
Looking ahead, the role of AI in making technology more accessible is expected to expand. Future trends may include more sophisticated AI models that understand and generate code with greater accuracy, as well as enhanced collaborative tools that bridge the gap between developers and non-developers. As generative AI continues to evolve, it holds the promise of transforming the software development process into a more inclusive, collaborative, and innovative endeavor. For instance, Gartner predicts that 80% of software engineers will have to reskill to fit into new roles created when generative AI takes over more programming functions, highlighting the significant impact of AI on the future of software development 5.