Generative AI: Moving Beyond Predictive Analytics for Better Decision-Making
Generative AI 🤖 has revolutionized decision-making 🤔 by not just predicting outcomes 📈 but also creating new opportunities 🌱 for smarter choices 🎯. With this cutting-edge tech 💡, the possibilities are endless ☁️🚀!
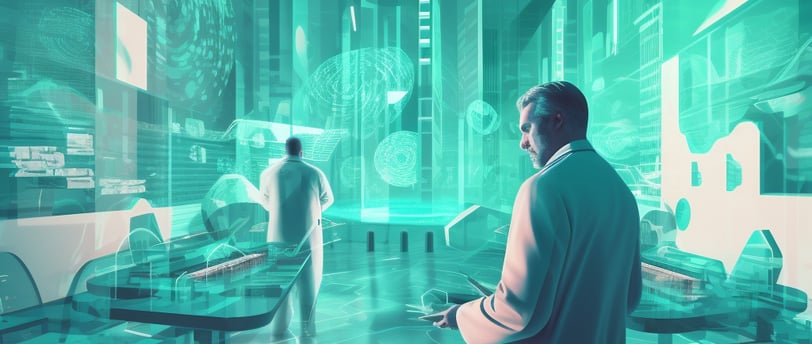
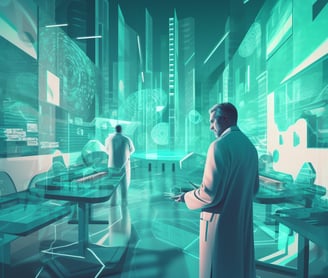
Generative AI and predictive analytics are two pivotal elements shaping the landscape of artificial intelligence in business decision-making. While predictive analytics utilizes historical data to forecast future trends, generative AI is emerging as a transformative tool, creating new, unprecedented data and insights. This paradigm shift is reshaping how businesses strategize and operate, offering a more nuanced and creative approach to data utilization and decision-making processes. The integration of these technologies reflects the dynamic and evolving nature of the modern corporate world, where data-driven insights are increasingly crucial for maintaining competitive advantage and operational efficiency.
In the contemporary business arena, data stands as a crucial asset. Predictive analytics has traditionally enabled organizations to decipher patterns in historical data for future predictions. However, the advent of generative AI marks a significant evolution, propelling businesses beyond conventional analytics. This newer technology not only predicts but also innovates, generating novel content and solutions. By exploring the intricacies of generative AI, businesses can uncover fresh opportunities for innovation and growth. As companies like Datasumi lead the way in AI solutions, the potential for leveraging generative AI in enhancing business strategy and gaining a competitive edge becomes increasingly evident.
Understanding Generative AI
Generative AI represents a significant leap forward in the realm of artificial intelligence. Unlike traditional predictive analytics, which focuses on extrapolating patterns from existing data, generative AI aims to create new data that resembles the habits and characteristics of the original dataset. It achieves this by employing deep learning models, such as generative adversarial networks (GANs) and variational autoencoders (VAEs). These models are designed to learn and simulate complex patterns, allowing businesses to generate new data points and scenarios beyond what historical data alone can offer.
Key Concerns with Generative AI
While generative AI holds immense potential, it also presents several key concerns that organizations must address. One primary concern is the ethical implications of generating synthetic data. As generative AI models learn from real-world data, there is a risk of inadvertently causing biased or discriminatory synthetic data. Businesses must ensure proper checks and balances to mitigate these risks and prevent unintended consequences.
Another concern is the computational resources required for training generative AI models. Deep learning models, particularly GANs, can be computationally intensive and demand substantial computing power. This poses challenges for organizations with limited resources or infrastructure. Overcoming these technical hurdles requires advanced hardware, efficient algorithms, and expertise in deploying and optimizing generative AI models.
GenAI Benefits for Businesses
Despite the concerns, the adoption of generative AI offers several potential benefits for businesses.
Enhanced Decision-Making: Generative AI empowers businesses to explore and evaluate many hypothetical scenarios. By generating new data points, organizations can uncover previously inaccessible insights through predictive analytics alone. This enables more informed decision-making, improving strategies, optimized operations, and better outcomes.
Innovation and Creativity: Generative AI fosters innovation by expanding the boundaries of what is possible. It enables businesses to generate novel ideas, designs, and solutions, thereby driving creativity and pushing the limits of traditional problem-solving approaches. By exploring uncharted territories, organizations can uncover untapped opportunities and gain a competitive edge in the market.
Risk Mitigation: Generative AI can help organizations identify and mitigate potential risks by simulating various scenarios. By generating synthetic data that captures different risk factors, businesses can proactively develop strategies to minimize adverse outcomes. This proactive risk management approach allows for more effective contingency planning and enables organizations to navigate uncertainties more resiliently.
Personalization and Customer Experience: Generative AI can be leveraged to create personalized customer experiences. Businesses can generate tailored recommendations, product variations, or even hyper-personalized marketing campaigns by analyzing customer preferences and behaviors. This level of personalization enhances customer satisfaction, boosts loyalty, and drives revenue growth.
Insights Crucial for Success
To effectively utilize generative AI, businesses need to focus on several key areas. Firstly, the quality of training data is paramount. The accuracy and reliability of generative AI models are heavily dependent on the comprehensiveness, representativeness, and unbiased nature of the training data. Ensuring high-quality data is crucial for generating precise and dependable outcomes. Secondly, there must be a collaborative effort between data scientists and domain experts. The intricate understanding of business context provided by domain experts can guide data scientists in creating meaningful synthetic data and extracting relevant insights. This interdisciplinary cooperation is essential to tailor generative AI solutions to an organization's specific needs and objectives.
Furthermore, it's vital to approach generative AI models as evolving tools. Continuous improvement through regular training and refinement is necessary to enhance their accuracy and relevance. As business environments and requirements change, these models should adapt accordingly, ensuring they remain effective and applicable. Additionally, ethical considerations are crucial in the deployment of generative AI. Organizations must vigilantly monitor synthetic data to avoid biases or discriminatory patterns. Implementing comprehensive governance frameworks and embracing diverse perspectives can help ensure fairness and accountability in the use of generative AI technologies.
How Datasumi Can Help?
Datasumi, recognized as a leader in AI solutions, offers an extensive array of tools and services that empower organizations to effectively harness generative AI. At the core of its offerings are advanced generative AI models, such as Generative Adversarial Networks (GANs) and Variational Autoencoders (VAEs). These models are trained on diverse datasets from various industries, enabling organizations to create high-quality synthetic data and gain valuable insights tailored to their specific needs. Additionally, Datasumi places a strong emphasis on the preparation and curation of training data. They assist businesses in refining their datasets through data cleansing, bias identification, and ensuring representativeness, thus building a solid foundation for reliable and effective generative AI models.
On the technical side, Datasumi provides scalable infrastructure solutions and optimization services to facilitate the computationally demanding tasks associated with generative AI. Leveraging cloud computing and advanced algorithms, they enable organizations to efficiently train and deploy generative AI models, irrespective of their resource constraints. Beyond the technical aspects, Datasumi is also deeply committed to ethical AI practices. The company aids businesses in establishing governance frameworks that address ethical concerns related to generative AI, ensuring responsible and unbiased use. This focus on ethical AI frameworks reinforces Datasumi’s dedication to upholding the highest standards of fairness and accountability in the application of generative AI technologies.
Conclusion
Generative AI represents a paradigm shift in the world of analytics, offering businesses the ability to move beyond predictive analytics and unlock new possibilities for decision-making. By generating synthetic data and exploring hypothetical scenarios, organizations can gain deeper insights, drive innovation, and mitigate risks more effectively. However, adopting generative AI requires careful consideration of ethical concerns, collaboration between data scientists and domain experts, and continuous model improvement. Datasumi, with its advanced AI models, data preparation expertise, infrastructure support, and ethical AI frameworks, stands as a trusted partner for businesses seeking to leverage generative AI and achieve transformative results. Embracing generative AI is crucial to better decision-making and a competitive advantage in today's data-driven world.
References
Folio3. (n.d.). Leverage Generative AI-Integrated Analytics For Decision Making. Retrieved from https://www.folio3.ai
SS&C Blue Prism. (n.d.). Generative AI vs. Predictive AI: Understanding the Difference. Retrieved from https://www.blueprism.com
TechTarget. (n.d.). Generative AI vs. predictive AI: Understanding the differences. Retrieved from https://www.techtarget.com
AIMunch. (n.d.). Predictive Analytics vs Generative AI: 10 Differences Explanation. Retrieved from https://www.aimunch.com
Pecan AI. (n.d.). Comparing Predictive and Generative AI: What Your Business Needs to Know. Retrieved from https://www.pecan.ai
Deloitte Finland. (n.d.). Generative AI deciphered. Retrieved from https://www2.deloitte.com
TDWI. (n.d.). Insight Accelerator | Using Generative AI to Improve Operational Efficiency and Data-Driven Decision-Making. Retrieved from https://tdwi.org