Guide to Mastering Data-Driven Decision Making
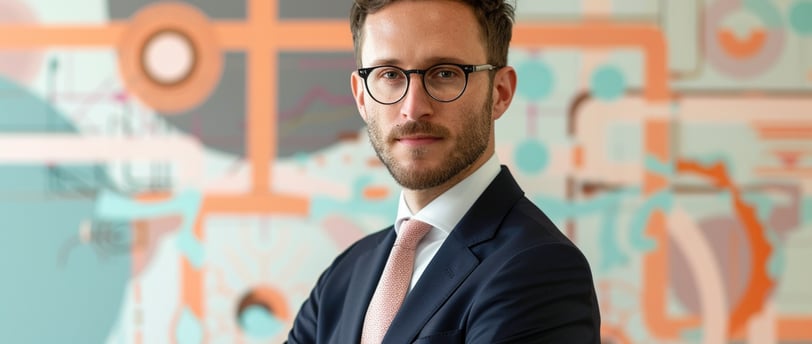
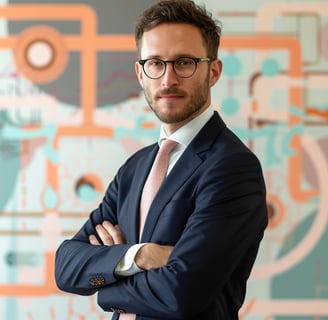
When the executive team at a Fortune 500 retail chain gathered for their quarterly strategy meeting last year, something was noticeably different. Instead of relying on industry experience and intuition as they had for decades, the room was now dominated by dashboards, visualizations, and predictive models. The shift wasn't merely aesthetic — it represented a fundamental transformation in how the company made critical decisions.
"We used to pride ourselves on gut instinct," a senior executive might have said. "Now we pride ourselves on being right."
In the span of just a few years, data-driven decision making has evolved from competitive advantage to business necessity. In an era where 90 percent of the world's data has been created in just the last two years, companies find themselves simultaneously data-rich and insight-poor. The ability to transform this torrent of information into strategic action has become the defining challenge for modern business leaders.
When Intuition Takes a Back Seat
Data-driven decision making, or DDDM as it's known in corporate corridors, represents a philosophical shift in how businesses operate. Unlike the traditional approach where experience and intuition guide major decisions, DDDM emphasizes empirical evidence and quantitative analysis. The approach isn't entirely new — companies have been collecting data for decades — but the scale, sophistication, and centrality to business strategy certainly is.
The transition hasn't been seamless. Many organizations still struggle with the cultural shift required to truly embrace data-driven approaches. After all, experienced executives who have built careers on instinct and industry knowledge don't easily cede authority to algorithms and statistical models.
Yet the evidence supporting this transition is becoming difficult to ignore. McKinsey research suggests companies that extensively use customer analytics are significantly more likely to generate above-average profits than competitors. The data revolution isn't just changing how businesses operate — it's separating market leaders from laggards.
Beyond the Spreadsheet
The advantages of data-driven decision making extend well beyond improved financial performance. In conversations with business leaders across industries, several themes consistently emerge.
First, there's the objectivity factor. Human decision making is notoriously susceptible to cognitive biases — from confirmation bias to overconfidence. Data provides a counterbalance, challenging assumptions and prevailing wisdom. When a major telecommunications provider analyzed customer complaint data rather than relying on executive summaries, they discovered that their understanding of customer pain points had been dramatically skewed by a vocal minority.
Strategic planning also benefits from the data revolution. Companies can now identify market trends and shifting customer preferences with remarkable precision, allowing them to position products and services ahead of emerging demand. This predictive capability doesn't eliminate risk, but it certainly helps quantify and manage it.
Perhaps most significantly, data has transformed customer understanding. The modern consumer leaves a digital trail that, when properly analyzed, reveals preferences and behaviors in unprecedented detail. This granular understanding allows for personalization at scale — something unimaginable just a decade ago.
The Analytics Engine Room
Behind every data-driven decision is a sophisticated analytics infrastructure. The field has evolved dramatically from simple descriptive metrics to complex predictive and prescriptive models.
Modern business analytics operates across a spectrum of sophistication. At one end, descriptive analytics answers the question "What happened?" through data aggregation and visualization. A retail chain tracking sales performance by location is employing descriptive analytics in its most basic form.
Moving up the value chain, diagnostic analytics addresses "Why did it happen?" This approach identifies causal factors and relationships, helping organizations understand the drivers behind business outcomes. When an e-commerce company investigates why conversion rates spiked during a particular campaign, they're engaged in diagnostic analysis.
The real competitive edge emerges with predictive and prescriptive analytics. Predictive models leverage historical data to forecast future trends and behaviors, while prescriptive analytics goes a step further by recommending specific actions to achieve desired outcomes. These advanced techniques, often powered by machine learning and artificial intelligence, represent the frontier of business intelligence.
From Insight to Action: The Implementation Roadmap
For organizations looking to strengthen their data-driven capabilities, the journey begins with clear business objectives. Successful analytics initiatives are anchored in specific business problems rather than abstract data exploration.
Data collection and preparation — often the least glamorous but most critical component — comes next. Companies must identify relevant data sources, implement robust collection mechanisms, and ensure data quality through cleansing and validation. In an age of increasing privacy regulation, compliance considerations must be paramount.
The analysis phase employs various techniques to extract insights, from statistical analysis to data visualization. Advanced organizations are increasingly leveraging machine learning algorithms to identify patterns too complex for human analysts to discern.
Perhaps the most overlooked aspect of data-driven decision making is the communication of insights. Even the most sophisticated analysis provides little value if key stakeholders can't understand its implications. Effective data storytelling — presenting insights in a compelling, accessible narrative — bridges the gap between analysis and action.
The final step, implementation, transforms insights into tangible business outcomes. This requires clear ownership, measurable objectives, and regular performance monitoring. The most successful organizations treat implementation as an iterative process, continuously refining strategies based on real-world results.
The Human Element
As businesses race to develop their analytical capabilities, many are discovering an uncomfortable truth: technology alone isn't enough. The human element remains essential.
"The most sophisticated algorithm can't replace judgment," as one technology executive put it. "Data informs decisions; it doesn't make them."
This perspective highlights an important distinction. Data-driven doesn't mean data-dictated. The goal isn't to remove human judgment from the equation but to ensure that judgment is informed by the best available evidence.
The most successful organizations are fostering data literacy across their workforce, not just among dedicated analysts. When frontline employees understand how to interpret and apply data in their daily decisions, the impact of analytics expands exponentially.
Looking Forward
As data volumes continue to grow exponentially and analytical techniques become increasingly sophisticated, the gap between data leaders and laggards will likely widen. Organizations that fail to develop robust data capabilities risk falling permanently behind.
Yet the future of data-driven decision making isn't just about more data or more advanced algorithms. The real frontier lies in integration — embedding analytics into the fabric of organizational processes and culture.
In the coming years, we may stop talking about "data-driven decision making" altogether. Not because it's become less important, but because it's become so fundamental to business that distinguishing it no longer makes sense. In this future, data isn't just how we make decisions — it's how we make everything.
Conclusion
In the fast-paced world of modern business, data-driven decision making has become an essential tool for success. By leveraging analytics, businesses can gain valuable insights, optimize operations, and make informed decisions that drive growth and profitability. Embracing a data-driven culture and investing in the right skills and capabilities is crucial for organizations to stay competitive and achieve sustainable success. As the landscape of data analytics continues to evolve, businesses must remain agile and adaptable, continuously refining their strategies to harness the power of data effectively.
FAQ Section
Q: What is data-driven decision making?
A: Data-driven decision making (DDDM) is a strategic approach that involves collecting and analyzing data to guide business decisions. It emphasizes the use of empirical evidence and quantitative analysis to minimize biases and make informed choices based on facts.
Q: What are the benefits of data-driven decision making?
A: DDDM offers numerous advantages, including objective and evidence-based decisions, improved strategic planning, enhanced operational efficiency, better customer understanding, real-time adaptability, and risk mitigation.
Q: How can businesses leverage analytics for decision making?
A: Businesses can leverage analytics by extracting insights from data, using descriptive, diagnostic, predictive, and prescriptive analytics, and integrating analytics into the decision-making process.
Q: What are the steps to implement data-driven decision making?
A: The steps include defining business objectives, collecting and preparing data, analyzing insights, communicating findings, and implementing data-driven decisions.
Q: What are some common challenges in implementing DDDM?
A: Common challenges include inaccurate or inconsistent data, resistance to change, difficulty integrating data tools, and concerns about data privacy.
Q: How can businesses overcome resistance to change in DDDM implementation?
A: Businesses can overcome resistance to change by fostering a culture of transparency and collaboration, involving stakeholders in the implementation process, and providing support and resources to facilitate the transition.
Q: What is the role of advanced analytics in data-driven decision making?
A: Advanced analytics techniques, such as predictive modeling, machine learning, and artificial intelligence, provide more sophisticated insights and support data-driven decision making by enabling businesses to forecast future outcomes and automate decision-making processes.
Q: How can businesses ensure data quality and integrity in DDDM?
A: Businesses can ensure data quality and integrity by implementing data governance practices, investing in data quality management tools, and fostering a culture of data quality.
Q: What are some future trends in data analytics for business?
A: Future trends include the rise of artificial intelligence (AI) and machine learning (ML), increasing importance of data privacy and security, and the democratization of data.
Q: How can businesses adapt to the evolving landscape of data analytics?
A: Businesses can adapt by continuously learning, innovating, and refining their analytics processes based on feedback and evolving business requirements.
Additional Resources
WGU's Guide to Data-Driven Decision Making
Explore WGU's comprehensive guide to understand the fundamentals of data-driven decision making and its applications in various industries1.
iWeb's Insights on Leveraging Analytics for Business Success
Dive into iWeb's detailed article to learn how leveraging analytics can drive business success and improve operational efficiency2.
Medium's Blog on Business Analytics
Read Medium's blog post to gain insights into the role of business analytics in data-driven decision making and its impact on business outcomes5.