History of NLP
Embark on a 📚 journey through the fascinating history of Natural Language Processing (NLP) 🗨️ and its transformative impact on the 🌐 business world. Uncover the 💡 benefits, 🚧 challenges, and 🌟 opportunities it presents. See how Datasumi 🚀 is revolutionizing industries by harnessing the immense power of NLP. ✨
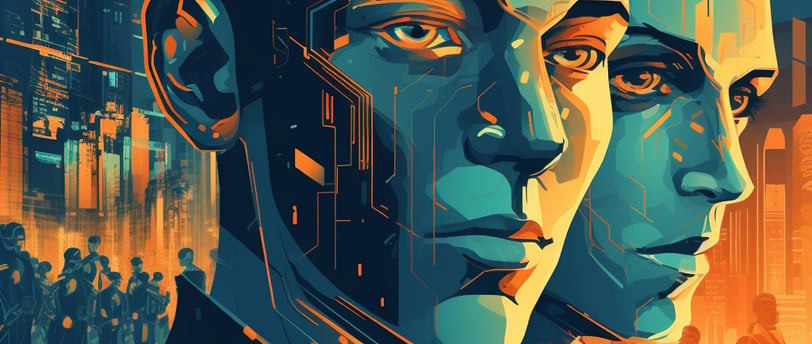
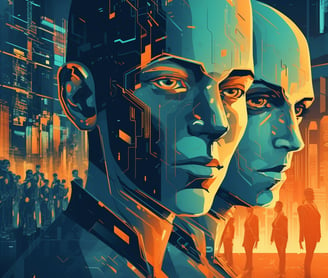
Throughout history, language has been a powerful means of communication and connection between individuals. Over time, it has evolved alongside humanity itself. Within the realm of artificial intelligence, a specialized field is dedicated to this fundamental aspect of human existence: Natural Language Processing. [1][2]Understanding the development and impact of NLP is not only an academic pursuit but also sheds light on how business and technology interact with one another to bring about transformative changes.
The Birth and Childhood of NLP
NLP was conceived in a world still getting used to the idea of machines that could compute numbers faster than any human. It was the 1950s when the very first attempts at machine translation began. The ambitious goal was straightforward: translate Russian texts to English using a computer.[3][4] This maiden voyage, funded by the U.S. government amidst the Cold War tensions, was optimistic. The thought was simple: If a machine could follow mathematical rules, why not linguistic ones? Yet, language is not just a set of rules. It's nuanced, cultural, and deeply contextual. The early machine translation projects ran into this hard truth. By the 1960s, the ALPAC (Automatic Language Processing Advisory Committee) [5][6]report concluded that machine translation was more expensive, less accurate, and slower than human translation.
Rise of The Machines
The road from there to our present world, where virtual assistants like Siri, Alexa, and Google Assistant understand and respond to our commands, was neither straightforward nor easy. The 1970s and 1980s saw AI winters – periods of skepticism and reduced funding. However, these were also times of foundational research. Rule-based systems made way for statistical methods in the 1990s. The volume of data available for training exploded, and machine learning algorithms became the new vogue.[7][8][9]
Why does this history matter?
It's straightforward: knowing the evolution helps understand its applications and potential pitfalls. Rule-based systems had a deterministic approach, making them suitable for tasks with clear-cut linguistic rules. In contrast, modern NLP, driven by deep learning, can understand sentiments, nuances, and sarcasm. For businesses, this means an unprecedented level of consumer insight drawn from structured feedback forms, tweets, blog posts, and reviews.[10][11][12][13][10][11]
The history of NLP is vast and rich. Here's an expanded overview of its development:
The 1950s - The Beginnings
The Turing Test (1950): Proposed by Alan Turing, this test was designed to determine whether a machine could demonstrate human-like intelligence. The idea was that if a machine could have a conversation with a human without the human realizing they were talking to a machine, it would pass the test.[14][15]
The Georgetown Experiment (1954): One of the earliest experiments in NLP, where more than 60 Russian sentences were automatically translated into English.[16][17]
1960s - Rule-based Systems
ELIZA (1966): Developed by Joseph Weizenbaum at MIT, ELIZA was a computer program that emulated a Rogerian psychotherapist, giving an illusion of understanding.[18][19]
SYSTRAN (1969): The first operational machine translation system was developed to translate Russian texts into English during the Cold War.[20][21]
1970s - Theoretical Underpinnings
Chomsky's Theories: Noam Chomsky's ideas on transformational grammar significantly influenced early NLP.[22][23]
MARGIE (1975): A system that represented language as semantic networks.[24][25][26][27]
The 1980s - Statistical Models and Corpora
Hidden Markov Models: These statistical tools have become widely used in many NLP applications.[28][29]
The Brown Corpus: One of the first and most widely used text corpora in linguistic studies, containing one million English words.[30][31]
1990s - Probabilistic Models and Machine Learning
Decision Trees and Maximum Entropy Models: Used for various NLP tasks.[32][33][34]
IBM's Candide (1994): One of the first data-driven machine translation systems based on statistical models.[35][36][37]
WordNet (1998): A lexical database for English, linking words into semantic relations.[38][39][40]
2000s - The Rise of Machine Learning and Deep Learning
Maximum Entropy and Conditional Random Fields (CRF): Became famous for tasks such as part-of-speech tagging and named entity recognition.[41][42]
Stanford Parser (2006): A statistical parser using machine learning to predict sentence structure structures.[43][44][45]
Neural Networks (The late 2000s): Deep learning was applied to NLP with better computational resources and datasets.[46][47][48]
2010s - Dominance of Deep Learning
Word Embeddings and Word2Vec (2013): Mikolov et al. introduced methods to convert words into vectors, capturing semantic meanings.[49][50]
Seq2Seq and Neural Machine Translation (2014): Sequence-to-sequence models started dominating machine translation tasks.[51][52][53]
BERT (2018) and Transformers: Introduced by Google, BERT (Bidirectional Encoder Representations from Transformers) sets new standards for various NLP tasks. Transformer architectures became the backbone of many subsequent models.[54][55][56]
GPT (Generative Pre-trained Transformer): Introduced by OpenAI, GPT models showcased the power of large-scale language models in generating coherent and contextually relevant text.[57][58][59]
The 2020s and Beyond
The 2020s saw the emergence and increasing influence of even larger models, such as GPT-3 and GPT-4, demonstrating the power of scaling and the potential of large language models in diverse applications.[60][59]
Throughout its history, NLP has evolved from rule-based systems to sophisticated deep learning architectures, paralleling the overall trajectory of artificial intelligence research. The future holds much promise, with potential breakthroughs like commonsense reasoning, cross-lingual understanding, and more human-like conversational agents.[61][62][63]
Pressing Issues & The Business World
However, the path is not without its thorns. One primary concern is the ethical use of NLP. As businesses use NLP tools for sentiment analysis, chatbots, or even recruitment processes, there's a lurking danger of biases. Machine learning models are only as good as the data they are trained on. If historical data is biased, the model will reproduce those biases.[64][65][66]
Moreover, data privacy regulations are becoming increasingly stringent. GDPR in Europe and CCPA in California are just the starting points. Businesses employing NLP must be vigilant about user data rights, usage permissions, and transparency in AI decisions.[67][68][69]
NLP's Promise to Business
Despite challenges, the benefits are immense. Customer service, for one, has been revolutionized. Chatbots powered by advanced NLP can handle multiple customer queries simultaneously, reducing wait times and improving user experience. In finance, NLP algorithms scour the news, predict market movements, and even help in fraud detection. Healthcare, legal, and e-commerce – the applications are vast and transformative.[70][71][72][73]
Beyond specific tasks, the strategic insights that NLP offers can guide businesses. Understanding consumer sentiment in real-time allows for agile strategy shifts. Spotting a negative trend early could mean the difference between a PR disaster and a minor hiccup.[74][75][76]
Introducing Datasumi: Your Trusted Partner in Navigating the NLP Landscape
In an era where digital transformation is no longer an option but a necessity, finding the right expert navigator to guide your journey through the maze of Natural Language Processing (NLP) and AI is crucial. Datasumi is the ally that has successfully guided myriad businesses through this labyrinth, leveraging its extensive expertise in AI, machine learning, and digital solutions. Committed to helping businesses implement AI and fully harness its potential, Datasumi crafts tailored strategies that amplify efficiency, minimize costs, and enhance competitive advantage.
What sets Datasumi apart is its holistic approach towards NLP and AI implementation. The firm believes that simply installing an NLP algorithm isn't sufficient; it must be a solution integrated harmoniously into a company's existing workflows. This means assessing your industry’s unique challenges and opportunities, identifying gaps that NLP can fill, and then developing a bespoke solution tailored to your specific needs.
Education is another cornerstone of Datasumi's approach. An NLP solution is only as good as a team that understands how to use it. That's why part of Datasumi's comprehensive service includes extensive employee training, ensuring that your staff understands the new tools at their disposal and knows how to use them effectively to drive the business forward.
Compliance is another aspect that Datasumi takes seriously. With a deep understanding of regulatory landscapes, including Government, PCI, HIPAA, and other industry-specific requirements, the team ensures that all implemented solutions fully comply with the latest standards and regulations. This attention to detail safeguards your business against potential legal pitfalls and instills stakeholder confidence.
Datasumi is more than a service provider; it's a strategic partner committed to guiding you through the complex NLP and AI adoption journey. With its in-depth expertise, custom-tailored solutions, commitment to training, and focus on compliance, Datasumi offers a full-spectrum approach that empowers businesses to optimize their operations and achieve a robust return on investment.
Concluding Insights
The story of NLP is a testament to human ambition, perseverance, and our never-ending quest to understand and replicate intelligence. For businesses, it offers a toolkit that, if used wisely, can drive growth, efficiency, and customer satisfaction. The past has been fascinating; the present is dynamic, and the future? With partners like Datasumi and the relentless march of innovation, the future looks promisingly intelligent.
References
Artificial Intelligence and the Loss of Humanity – Berkeley Political .... https://bpr.berkeley.edu/2020/11/15/artificial-intelligence-and-the-loss-of-humanity/.
The brief history of artificial intelligence: The world has changed .... https://ourworldindata.org/brief-history-of-ai.
A Brief History of Natural Language Processing - DATAVERSITY. https://www.dataversity.net/a-brief-history-of-natural-language-processing-nlp/.
Natural language processing - Wikipedia. https://en.wikipedia.org/wiki/Natural_language_processing.
Language And Culture Relationship - A Detailed Guide - TLD. https://thelanguagedoctors.org/what-is-the-language-and-culture-relationship/.
3.4 Language, Society, and Culture – Communication in the Real World. https://open.lib.umn.edu/communication/chapter/3-4-language-society-and-culture/.
What Are Virtual Assistants and What Can You Do With Them?. https://www.pcmag.com/news/what-are-virtual-assistants-and-what-can-you-do-with-them.
The man who helped invent virtual assistants thinks they’re doomed .... https://www.technologyreview.com/2019/03/13/136661/virtual-assistants-thinks-theyre-doomed-without-a-new-ai-approach/.
Alexa, Siri, Google Assistant: How The Top Smart Assistants ... - Forbes. https://www.forbes.com/sites/johnkoetsier/2020/08/08/alexa-siri-google-assistant-how-the-top-smart-assistants-stack-up/.
Evolution and natural selection review (article) | Khan Academy. https://www.khanacademy.org/science/high-school-biology/hs-evolution/hs-evolution-and-natural-selection/a/hs-evolution-and-natural-selection-review.
What Evolution Can Teach Us About Innovation - Harvard Business Review. https://hbr.org/2021/09/what-evolution-can-teach-us-about-innovation.
The influence of evolutionary history on human health and disease - Nature. https://www.nature.com/articles/s41576-020-00305-9.
Evolution and Society | National Academies. https://www.nationalacademies.org/evolution/evolution-and-society.
Turing Test - TechTarget. https://www.techtarget.com/searchenterpriseai/definition/Turing-test.
Computing Machinery and Intelligence - Wikipedia. https://en.wikipedia.org/wiki/Computing_Machinery_and_Intelligence.
The Georgetown-IBM Experiment Demonstrated in January 1954. https://link.springer.com/chapter/10.1007/978-3-540-30194-3_12.
The Georgetown-IBM Experiment: MT's First Major Debut. https://www.unitedlanguagegroup.com/blog/translation/georgetown-ibm-experiment-mt-first-major-debut.
The ELIZA Effect: Joseph Weizenbaum and the Emergence of Chatbots. https://academic.oup.com/book/39707/chapter/339718866.
ELIZA - Wikipedia. https://en.wikipedia.org/wiki/ELIZA.
The history of machine translation in a nutshell - ACL Anthology. https://aclanthology.org/www.mt-archive.info/10/Hutchins-2014.pdf.
Machine translation - Wikipedia. https://en.wikipedia.org/wiki/Machine_translation.
Noam Chomsky's Language Theory - Study.com. https://study.com/learn/lesson/noam-chomskys-language-theory-overview-explanation-examples.html.
(PDF) Chomsky's Generative Transformational Grammar and Its .... https://www.researchgate.net/publication/335230901_Chomsky's_Generative_Transformational_Grammar_and_Its_Implications_on_Language_Teaching.
Semantic networks - ScienceDirect. https://www.sciencedirect.com/science/article/pii/0898122192901355.
Semantic Networks - Cambridge University Press & Assessment. https://www.cambridge.org/core/books/cambridge-handbook-of-systemic-functional-linguistics/semantic-networks/797034B35D9DEADB359B266B413C53A6.
Semantic Networks shared meaning-revised - University of Illinois .... http://jdiesnerlab.ischool.illinois.edu/publications/Semantic_Networks_Diesner_Carley_2011.pdf.
Semantic network - Wikipedia. https://en.wikipedia.org/wiki/Semantic_network.
A New Algorithm for Hidden Markov Models Learning Problem - arXiv.org. https://arxiv.org/pdf/2102.07112.
Statistical Inference in Hidden Markov Models Using. https://www.ncbi.nlm.nih.gov/pmc/articles/PMC4867884/.
CoRD | The Freiburg-Brown corpus of American English (FROWN) - Helsinki. https://varieng.helsinki.fi/CoRD/corpora/FROWN/background.html.
Brown Corpus - Wikipedia. https://en.wikipedia.org/wiki/Brown_Corpus.
Natural Language Processing for Information Extraction - arXiv.org. https://arxiv.org/pdf/1807.02383.pdf.
A Maximum Entropy Approach to Natural Language Processing. https://courses.csail.mit.edu/6.891-nlp/J1996-1002.pdf.
Maximum Entropy Model - ScienceDirect. https://www.sciencedirect.com/topics/computer-science/maximum-entropy-model.
IBM100 - Pioneering Machine-Aided Translation. https://www.ibm.com/ibm/history/ibm100/us/en/icons/translation/.
Statistical Machine Translation: IBM Models 1 and 2 - Stanford University. https://web.stanford.edu/class/archive/cs/cs224n/cs224n.1162/handouts/Collins_annotated.pdf.
The Candide System for Machine Translation - ACL Anthology. https://aclanthology.org/H94-1028/.
WordNet: An Electronic Lexical Database - Google Books. https://books.google.com/books/about/WordNet.html?id=Rehu8OOzMIMC.
A Semantic Network of English: The Mother of All WordNets - JSTOR. https://www.jstor.org/stable/30200461.
WordNet. https://wordnet.princeton.edu/.
Conditional random field - Wikipedia. https://en.wikipedia.org/wiki/Conditional_random_field.
Maximum-entropy Markov model - Wikipedia. https://en.wikipedia.org/wiki/Maximum-entropy_Markov_model.
Stanford Parser [linguisticsweb.org]. http://linguisticsweb.org/doku.php?id=linguisticsweb:tutorials:automaticannotation:stanford_parser.
The Stanford Natural Language Processing Group. https://nlp.stanford.edu/software//srparser.html.
The Stanford Natural Language Processing Group. https://nlp.stanford.edu/projects/stat-parsing.shtml.
1 Recent Trends in Deep Learning Based Natural Language Processing. https://arxiv.org/pdf/1708.02709.pdf.
Neural Networks for NLP - Devopedia. https://devopedia.org/neural-networks-for-nlp.
Improving the Reliability of Deep Neural Networks in NLP: A Review .... https://www.sciencedirect.com/science/article/abs/pii/S0950705119305428.
word2vec Explained: Deriving Mikolov et al.’s Negative-Sampling Word .... https://arxiv.org/pdf/1402.3722.pdf.
word2vec Explained: deriving Mikolov et al.'s negative-sampling word .... https://arxiv.org/abs/1402.3722.
Understanding and Improving Sequence-to-Sequence Pretraining for Neural .... https://arxiv.org/abs/2203.08442.
Introduction to Seq2Seq Translators with PyTorch - Paperspace Blog. https://blog.paperspace.com/seq2seq-translator-pytorch/.
Seq2Seq Explained | Papers With Code. https://paperswithcode.com/method/seq2seq.
BERT: Pre-training of Deep Bidirectional Transformers for Language .... https://research.google/pubs/pub47751/.
[1810.04805] BERT: Pre-training of Deep Bidirectional Transformers for .... https://arxiv.org/abs/1810.04805.
Understanding BERT — (Bidirectional Encoder Representations from .... https://towardsdatascience.com/understanding-bert-bidirectional-encoder-representations-from-transformers-45ee6cd51eef.
GPT-2 - Wikipedia. https://en.wikipedia.org/wiki/GPT-2.
arXiv:1810.04805v2 [cs.CL] 24 May 2019. https://arxiv.org/pdf/1810.04805.pdf.
GPT-3 - Wikipedia. https://en.wikipedia.org/wiki/GPT-3.
Large Language Models and GPT-4 Explained | Towards AI - Medium. https://pub.towardsai.net/large-language-models-and-gpt-4-architecture-and-openai-api-d8f1c070e0fc.
How Deep Learning Revolutionized NLP - Springboard. https://www.springboard.com/blog/data-science/nlp-deep-learning/.
Deep Learning: A Comprehensive Overview on Techniques, Taxonomy .... https://link.springer.com/article/10.1007/s42979-021-00815-1.
The future of natural language processing. https://www.future-processing.com/blog/the-future-of-natural-language-processing/.
What is Natural Language Processing? | IBM. https://www.ibm.com/topics/natural-language-processing.
Ethical principles in machine learning and artificial intelligence .... https://www.nature.com/articles/s41599-020-0501-9.
The six main subsets of AI: (Machine learning, NLP, and more) - Akkio. https://www.akkio.com/post/the-five-main-subsets-of-ai-machine-learning-nlp-and-more.
Data protection in the EU - European Commission. https://commission.europa.eu/law/law-topic/data-protection/data-protection-eu_en.
GDPR and CCPA Overview: Privacy Changes and Your Role in Data Protection. https://www.securitymetrics.com/blog/gdpr-and-ccpa-overview-privacy-changes-and-your-role-data-protection.
A guide to GDPR data privacy requirements - GDPR.eu. https://gdpr.eu/data-privacy/.
AI customer service for higher customer engagement - McKinsey & Company. https://www.mckinsey.com/capabilities/operations/our-insights/the-next-frontier-of-customer-engagement-ai-enabled-customer-service.
AI Chatbots for Customer Service: Benefits, Challenges, and Solutions. https://www.linkedin.com/advice/3/what-benefits-challenges-using-ai-chatbots-customer.
The benefits of improving customer experience in your business - Qmatic. https://www.qmatic.com/blog/the-benefits-of-improving-customer-experience-in-your-business.
Improving the Customer Service Experience - Harvard Business Review. https://hbr.org/sponsored/2021/09/improving-the-customer-service-experience.
11 NLP Applications & Examples in Business - MonkeyLearn. https://monkeylearn.com/blog/natural-language-processing-applications/.
25 NLP tasks at a glance. - Medium. https://medium.com/@miranthaj/25-nlp-tasks-at-a-glance-52e3fdff32e2.
NLP vs. NLU vs. NLG: the differences between three natural ... - IBM. https://www.ibm.com/blog/nlp-vs-nlu-vs-nlg-the-differences-between-three-natural-language-processing-concepts/.