How can I automate Data Governance?
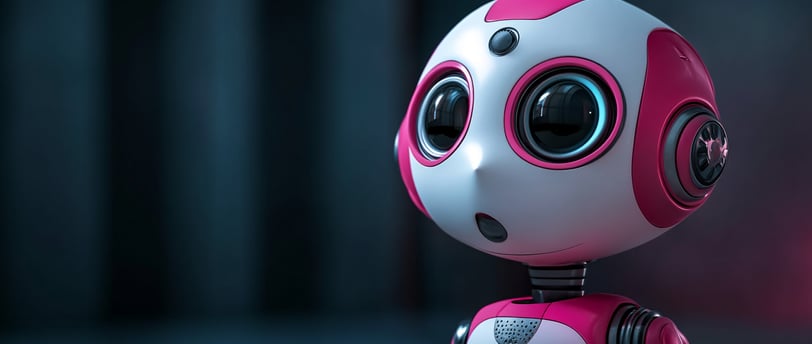
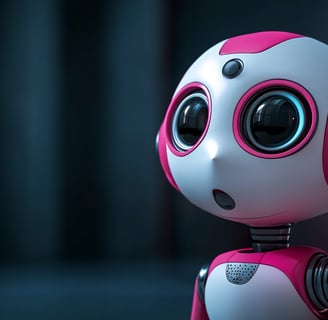
In today’s data-driven world, organizations are inundated with vast amounts of information, making effective data governance more critical than ever. The traditional methods of managing data quality and ensuring compliance are no longer sufficient. Enter the era of AI-driven data governance automation—a transformative approach that promises to revolutionize how organizations handle their data. This article will delve into the world of AI-driven data governance, exploring how automation and AI technologies are enhancing data quality, streamlining compliance, and driving strategic decision-making.
Understanding Data Governance and Its Challenges
The Evolution of Data Governance
Data governance has evolved significantly from simple data management practices to complex frameworks that ensure data quality, security, and compliance. Traditional data governance involved manual processes such as data entry, validation, and reporting. However, with the exponential growth of data, these manual methods have become inefficient and error-prone. The advent of AI and automation has introduced new possibilities for managing data more effectively1.
Key Challenges in Data Governance
Data Quality Issues: One of the primary challenges in data governance is maintaining high data quality. Data inconsistencies, inaccuracies, and incompleteness can lead to poor decision-making and operational inefficiencies. Traditional methods of data quality management often involve manual efforts that are time-consuming and prone to human error1.
Compliance and Regulatory Requirements: Organizations today must comply with an increasing number of data protection regulations, such as the General Data Protection Regulation (GDPR) in Europe and the California Consumer Privacy Act (CCPA) in the United States. These regulations impose stringent requirements on how data is collected, stored, and processed, making compliance a critical aspect of data governance1.
Data Silos and Fragmentation: Data silos, where data is isolated within departments, hinder comprehensive data governance. Fragmented data across various systems makes it challenging to maintain a unified and consistent data governance framework1.
AI-Driven Data Quality Management
Machine Learning for Data Quality
AI and machine learning (ML) algorithms significantly enhance data quality management by automating data cleansing, validation, and enrichment processes. These algorithms can identify and rectify anomalies, duplicates, and errors in data sets with high precision, ensuring that data quality remains consistent and reliable1.
Predictive Analytics: Predictive analytics can proactively identify potential data quality issues before they impact operations. By analyzing historical data patterns, AI systems can forecast and prevent data quality problems, ensuring continuous data reliability1.
Natural Language Processing (NLP): NLP techniques enable the extraction and normalization of unstructured data from diverse sources. This capability is essential for maintaining data quality across various data formats and enhancing the overall data governance framework1.
Automated Data Contracts
Definition and Purpose
Automated data contracts are self-executing agreements that define the terms and conditions for data usage and sharing. These contracts are enforced by code, ensuring that data governance policies are adhered to without manual intervention1.
Smart Contracts and Blockchain: Smart contracts, often implemented on blockchain platforms, offer a secure and transparent way to automate data governance policies. They ensure data integrity and compliance by automatically executing predefined rules and conditions1.
Benefits of Automated Data Contracts: Automated data contracts ensure the consistent application of data governance policies, significantly reducing the risk of human error and non-compliance. By embedding data governance rules directly into the code of smart contracts, organizations can automate the enforcement of data policies. This ensures that all data transactions adhere to predefined guidelines, thus maintaining data integrity and regulatory compliance1.
Implementing AI-Driven Data Governance
Integrating AI into Existing Systems
Organizations should adopt a phased approach to integrating AI into their existing data governance frameworks. This involves identifying critical data quality issues and implementing AI solutions to address them incrementally1.
Developing and Deploying Smart Contracts
To implement automated data contracts, organizations must collaborate with legal, IT, and data management teams to define contract parameters. Deploying smart contracts on blockchain platforms requires careful planning and alignment with organizational policies1.
Continuous Monitoring and Improvement
AI-driven data quality management and automated data contracts require continuous monitoring and refinement. Organizations should establish feedback loops to assess the effectiveness of these technologies and make necessary adjustments1.
Case Studies
Financial Services
A leading financial services firm implemented AI-driven data quality management to enhance its data governance framework. The use of machine learning algorithms reduced data errors by 40%, improving decision-making processes and regulatory compliance1.
Healthcare
A healthcare organization deployed automated data contracts to manage patient data sharing among various stakeholders. Blockchain-based smart contracts ensured data integrity and compliance with HIPAA regulations, enhancing patient trust and operational efficiency1.
Conclusion
The integration of AI-driven data quality management and automated data contracts represents a significant advancement in data governance. These technologies offer solutions to longstanding challenges, providing organizations with the tools to ensure data accuracy, compliance, and efficiency. By embracing these innovations, organizations can foster a robust data governance ecosystem that supports strategic decision-making and regulatory adherence. The future of data governance is here, and it is powered by AI.
FAQ Section
What is AI-driven data governance?
AI-driven data governance is the use of artificial intelligence technologies to manage and govern data more effectively. This includes automating data quality management, ensuring compliance with regulations, and improving overall data integrity and security.
How does AI enhance data quality?
AI enhances data quality by automating processes such as data cleansing, validation, and enrichment. Machine learning algorithms can identify and correct anomalies, duplicates, and errors in data sets, ensuring higher data quality and reliability.
What are automated data contracts?
Automated data contracts are self-executing agreements that define the terms and conditions for data usage and sharing. They are enforced by code, typically using smart contract technology on blockchain platforms, to ensure data governance policies are adhered to without manual intervention.
How do smart contracts ensure compliance?
Smart contracts ensure compliance by embedding data governance rules directly into the code. This automates the enforcement of data policies, ensuring that all data transactions adhere to predefined guidelines, thus maintaining data integrity and regulatory compliance.
What are the benefits of AI-driven data governance?
The benefits of AI-driven data governance include improved data quality, enhanced compliance, increased operational efficiency, and reduced costs associated with manual data governance tasks. It also provides a more secure and transparent data management framework.
How can organizations implement AI-driven data governance?
Organizations can implement AI-driven data governance by adopting a phased approach, integrating AI into existing data governance frameworks, developing and deploying smart contracts, and continuously monitoring and improving their data governance practices.
What are some challenges in traditional data governance?
Challenges in traditional data governance include maintaining high data quality, complying with regulatory requirements, and managing data silos and fragmentation. Traditional methods often involve manual processes that are time-consuming and prone to human error.
How does predictive analytics help in data governance?
Predictive analytics helps in data governance by proactively identifying potential data quality issues before they impact operations. By analyzing historical data patterns, AI systems can forecast and prevent data quality problems, ensuring continuous data reliability.
What role does NLP play in data governance?
NLP techniques enable the extraction and normalization of unstructured data from diverse sources. This capability is essential for maintaining data quality across various data formats and enhancing the overall data governance framework.
How do automated data contracts improve efficiency?
Automated data contracts improve efficiency by automating routine governance tasks, such as data validation, access control, and logging without manual intervention. This allows IT and data governance teams to focus on more complex and value-added activities.