How does AI enhance Fraud Detection in Social Security Systems?
With the sheer amount of data being generated every second, fraud detection in social security systems has become a top priority worldwide. But how can AI help enhance this process and combat fraudulent activity?

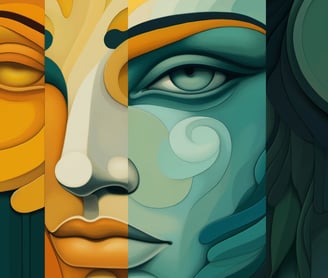
The proliferation of digital data in today's modern era has elevated the significance of fraud detection in social security systems worldwide. These indispensable systems exist to supply individuals with crucial financial assistance and safeguard their welfare, rendering them alluring targets for fraudulent endeavors. However, thanks to groundbreaking advancements in artificial intelligence, the landscape of fraud detection has undergone a dramatic transformation, showcasing immense potential for more effective identification of suspicious patterns and anomalies. [1][2]
This article delves into the remarkable impact that AI brings to enhancing fraud detection capabilities within social security systems. It not only addresses pertinent concerns but also explores the potential benefits this technology offers businesses while providing invaluable insights necessary for achieving success within this domain. Moreover, we will shed light on Datasumi—a preeminent AI-powered analytics platform—whose pivotal role assists organizations greatly in effectively combatting fraudulent activities. In the insurance sector, the integration of artificial intelligence and machine learning systems presents various opportunities for enhancing claims processing, customer service, and fraud detection[3].
Fraud in social security systems encompasses various activities, such as identity theft, false claims, and benefit misuse. These fraudulent activities not only result in financial losses for the system but also undermine the trust and confidence of beneficiaries. Traditional methods of fraud detection, relying on manual review and rule-based systems, are often inadequate to deal with the growing complexity and volume of fraudulent activities. Here is where AI technologies come into play. These cutting-edge technologies leverage data mining, statistics, and machine learning algorithms to effectively detect fraud patterns, identify anomalies, and flag suspicious transactions.[4][5]
AI and Machine Learning for Fraud Detection
AI, specifically machine learning (ML), has emerged as a powerful tool for fraud detection due to its ability to analyze vast amounts of data, identify patterns, and detect anomalies. ML algorithms can be trained on historical data to learn patterns of fraudulent behavior and then applied to new data to identify potential instances of fraud. By continuously learning and adapting to new patterns, these algorithms can improve detection accuracy over time.
Putting your faith exclusively in traditional programmed systems for detecting financial fraud isn't optimal[6].
Leveraging AI for Fraud Detection in Social Security Systems
AI technology significantly enhances fraud detection in various fields, potentially including social security systems. In the insurance sector, AI and machine learning systems can be integrated into claims processing, customer service, and fraud detection sub-sectors to improve efficiency and detect fraudulent activities more effectively[3]. Here's a breakdown based on the experiences:
Automated Fraud Detection
An instance from Michigan showcased the employment of a $47 million automated fraud detection system in 2013, which resulted in approximately 48,000 fraud accusations against unemployment insurance recipients, marking a five-fold increase. This demonstrates the potential of AI technology to automate fraud detection processes and identify suspicious activities in social security systems.[7]
Uncovering Complex Patterns
AI-powered fraud detection can reveal complex and subtle fraudulent patterns that might go unnoticed by traditional, rule-based systems. This enhancement in detection rates minimizes false negatives, ensuring that fraudulent activities do not slip through the cracks. By leveraging advanced techniques such as supervised, semi-supervised, and unsupervised learning, as well as deep neural networks and decision trees, AI models can uncover hidden patterns and relationships in the data, allowing for more accurate detection of fraud in social security systems[8].
Automation and Enhanced Quality of Work
AI systems can handle time-consuming and repetitive tasks like data collection and analysis, which allows fraud analysts to concentrate on critical tasks requiring human intervention, such as monitoring risk scores. This automation not only saves time but also augments the quality of fraud analysts' work. By integrating AI and machine learning systems into social security fraud detection, the accuracy and efficiency of the process can be greatly improved. Furthermore, AI technology has the potential to assist in identity forgery and corporate fraud detection[9].
Challenges and Risks
Despite its advantages, AI in fraud detection also presents certain challenges. For instance, social fraud, encompassing phishing and social engineering, proves difficult to combat with AI as these threats are not automated. This highlights that while AI can significantly aid in fraud detection, human intervention and oversight remain crucial. In response to the challenges posed by social fraud, researchers are continuously exploring new approaches and technologies to enhance AI-powered fraud detection systems.[10]
In addition, AI-supported banking fraud and corporate fraud pose further challenges to the effectiveness of AI in detection. In the case of AI-supported banking fraud, AI may actually facilitate identity forgery, including through advancements in voice synthesis technology. This can make fraud easier to commit as AI techniques can be used to convincingly forge an individual's identity.[11]
The examples and risks outlined above may have parallel applications in fraud detection within social security systems, where AI can automate processes, uncover complex fraud schemes, and augment the efficiency and effectiveness of fraud detection efforts. However, it's crucial to address the potential limitations and ensure a balanced approach to maximize the benefits of AI in enhancing fraud detection in social security systems.[12][13]
Insights for Success
Artificial Intelligence (AI) significantly elevates fraud detection in Social Security Systems by enabling centralized data analysis, real-time fraud detection, and predictive modeling to anticipate fraudulent activities. Through automation, it streamlines the fraud detection process, relieving analysts from mundane tasks, and enhancing pattern recognition to identify fraudulent schemes swiftly. AI's role in reducing operational costs is notable, as it minimizes false positives and accelerates fraud investigations. Furthermore, AI aids in ensuring compliance with regulatory and internal business policies, thereby not only advancing technological integration but also bolstering security, efficiency, and cost-effectiveness in managing social welfare resources. Here are insights and examples based on your request:
Centralized Data Analysis
AI and advanced analytics allow the amalgamation of diverse data into a centralized dataset. This centralized approach facilitates a holistic analysis to identify anomalies and hidden patterns indicative of fraud, which is significantly more efficient compared to manual methods.[14]
Real-Time Fraud Detection
AI transforms the paradigm of fraud detection from reactive to proactive. It enables real-time fraud detection, thus reducing the latency traditionally seen where chargebacks appear six to eight weeks post fraud occurrence. Advanced AI technologies can detect fraud attacks in less than a second, a capability beyond the reach of rule-based systems.[12]
Predictive Modeling
Employing predictive modeling based on historical data, AI helps in anticipating fraudulent activities before they occur. This preemptive approach is pivotal in enhancing the efficacy of fraud detection systems, enabling them to evolve with emerging fraud patterns.[15][16]
Automation and Efficiency
AI significantly automates the fraud detection process, relieving fraud analysts from time-consuming and repetitive tasks. This automation not only accelerates fraud detection but also enhances the quality of work, allowing analysts to focus on critical tasks that necessitate human intervention.[17]
Enhanced Pattern Recognition
Through machine learning (ML), a subset of AI, fraud detection systems improve both accuracy and efficiency in real-time environments. ML, especially when applied continuously, has proven to be a robust approach towards combating financial crimes by enhancing pattern recognition capabilities.[18][19]
Cost Reduction
By minimizing false positives and improving the precision of fraud detection, AI helps in reducing the costs associated with fraud detection and investigation. This, in turn, has a domino effect on reducing fraud losses and overall operational costs. Additionally, AI and machine learning have the potential to adapt and learn from new fraud patterns, making it a valuable tool for staying ahead of evolving fraud techniques and mitigating potential risks in a proactive manner.[20]
Compliance and Regulatory Adherence
AI-based fraud prevention systems are instrumental in ensuring compliance with internal business policies, regulatory agency guidelines, and distribution partner agreements. This aspect is particularly crucial for digital businesses operating in high technology industries with stringent export regulations. To summarize, AI and machine learning play a vital role in fraud detection by enhancing accuracy, automating processes, improving pattern recognition, reducing costs, and ensuring compliance with regulations.[21][22]
The integration of AI in fraud detection within Social Security Systems is not just a leap towards advanced technological adoption but a significant stride towards enhanced security, efficiency, and cost-effectiveness in managing social welfare resources. The integration of AI in fraud detection within Social Security Systems is not just a leap towards advanced technological adoption but a significant stride towards enhanced security, efficiency, and cost-effectiveness in managing social welfare resources.
How Datasumi Can Help
Datasumi, a leading AI analytics consultancy, offers cutting-edge solutions for fraud detection in social security systems. With its robust machine learning algorithms and advanced analytics capabilities, Datasumi can assist social security agencies in combating fraud effectively. Datasumi's platform integrates with existing systems, enabling seamless data integration and real-time monitoring. Its anomaly detection algorithms and predictive analytics models empower agencies to identify fraudulent activities and take proactive measures. Moreover, Datasumi's user-friendly interface and visualization tools facilitate efficient collaboration between human analysts and AI systems, ensuring optimal fraud detection outcomes.
Conclusion
The integration of Artificial Intelligence (AI) in fraud detection within Social Security Systems heralds a transformative shift towards more secure, efficient, and cost-effective management of social welfare resources. By leveraging centralized data analysis, real-time fraud detection, predictive modeling, and automation, AI not only accelerates the fraud detection process but also significantly enhances its accuracy. The consequent reduction in operational costs and improved regulatory compliance are additional boons, underscoring the profound potential of AI in combating fraud. The insights gleaned from various examples and implementations emphasize the invaluable role of AI in transcending traditional fraud detection paradigms, thereby fostering a more robust and reliable social security infrastructure.
Artificial intelligence has emerged as a transformative tool in the field of fraud detection for social security systems, offering improved efficiency and accuracy when it comes to identifying fraudulent activities. By utilizing techniques such as data integration, anomaly detection, predictive analytics, and real-time monitoring, AI systems have the capability to enhance accuracy in detecting fraudulent behavior while reducing false positive results. This not only ensures greater operational efficiency but also helps safeguard the interests of beneficiaries. It is crucial for businesses and organizations involved in fraud detection to recognize and embrace AI's potential as a strategic investment. Datasumi offers advanced solutions for fraud detection through its AI-powered analytics platform, enabling social security agencies to protect their systems effectively. Leveraging these intelligent technologies allows organizations to proactively tackle fraudsters' attempts while preserving the integrity of their social security systems.
References
Digital technology and social change: the digital transformation of .... https://www.tandfonline.com/doi/full/10.31887/DCNS.2020.22.2/mhilbert.
FRAUD IN THE DIGITAL AGE - IFAC. https://www.ifac.org/system/files/uploads/IAASB/Technology%20Fraud%20Roundtable%20-%20Covernote%20and%20Agenda.pdf.
Dhieb, Najmeddine, et al. A Secure AI-Driven Architecture for Automated Insurance Systems: Fraud Detection and Risk Measurement. 1 Jan. 2020, https://scite.ai/reports/10.1109/access.2020.2983300.
Fraud Hotline - Social Security Administration. https://oig-files.ssa.gov/fraud-advisory/Fraud.pdf.
FAQ | Office of the Inspector General - Social Security Administration. https://oig.ssa.gov/fraud-reporting/faq/.
Padala, Siddesh, Venkatsai, et al. Machine Learning: The New Language for Applications. 1 Dec. 2019, https://scite.ai/reports/10.11591/ijai.v8.i4.pp411-421.
Fraud detection system with 93% failure rate gets IT companies sued. https://www.theregister.com/2017/03/08/fraud_detection_system_with_93_failure_rate_gets_it_companies_sued/.
Shen, Hongda, and Eren Kurshan. Deep Q-network-based adaptive alert threshold selection policy for payment fraud systems in retail banking. 15 Oct. 2020, https://scite.ai/reports/10.1145/3383455.3422563.
King, C., Thomas, et al. Artificial Intelligence Crime: An Interdisciplinary Analysis of Foreseeable Threats and Solutions. 1 Jan. 2021, https://scite.ai/reports/10.1007/978-3-030-81907-1_13.
Importance & Working of AI in Fraud Detection - HyperVerge. https://hyperverge.co/blog/what-is-ai-in-fraud-detection/.
Artificial Intelligence Crime: An Interdisciplinary Analysis of .... https://link.springer.com/article/10.1007/s11948-018-00081-0.
Financial Fraud: A Review of Anomaly Detection ... - ScienceDirect. https://www.sciencedirect.com/science/article/pii/S0957417421017164.
Artificial Intelligence and Fraud Detection | SpringerLink. https://link.springer.com/chapter/10.1007/978-3-030-75729-8_8.
Artificial intelligence approaches and mechanisms for big data .... https://www.ncbi.nlm.nih.gov/pmc/articles/PMC8053021/.
AI-Based Modeling: Techniques, Applications and Research Issues Towards .... https://www.ncbi.nlm.nih.gov/pmc/articles/PMC8830986/.
Definition of Predictive Modeling - Gartner Information Technology Glossary. https://www.gartner.com/en/information-technology/glossary/predictive-modeling.
How AI is revolutionising fraud detection - SourceSecurity.com. https://www.sourcesecurity.com/insights/ai-revolutionising-fraud-detection-co-1619680890-ga.1619681736.html.
Understand 3 Key Types of Machine Learning - Gartner. https://www.gartner.com/smarterwithgartner/understand-3-key-types-of-machine-learning.
Machine Learning for Fraud Detection: Use Cases & Guidelines - Itransition. https://www.itransition.com/machine-learning/fraud-detection.
Financial Fraud: A Review of Anomaly Detection Techniques and Recent .... https://www.sciencedirect.com/science/article/pii/S0957417421017164.
Full article: Banking on AI: mandating a proactive approach to AI .... https://www.tandfonline.com/doi/full/10.1080/17521440.2020.1760454.
How Artificial Intelligence Will Change the Way We Manage Compliance .... https://bankingjournal.aba.com/2021/07/how-artificial-intelligence-will-change-the-way-we-manage-compliance/.