Industry-Specific LLM Fine-Tuning is Revolutionising Healthcare, Finance, and Manufacturing
Explore how targeted LLM fine-tuning is transforming healthcare diagnostics, financial risk assessment, and manufacturing efficiency with domain-specific AI solutions that deliver unprecedented accuracy and compliance.
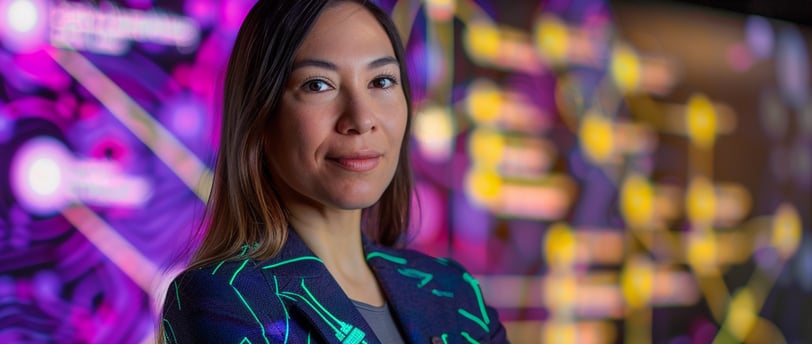
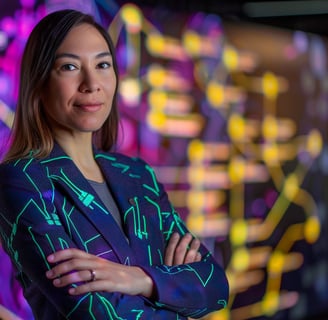
Imagine a world where AI doesn't just understand human language but comprehends the intricate terminology of an operating room, deciphers complex financial regulations, or optimizes manufacturing processes with expert precision. This isn't science fictionโit's the reality being created through industry-specific Large Language Model (LLM) fine-tuning. The era of generic AI solutions is giving way to highly specialized systems that speak the unique languages of healthcare, finance, and manufacturing. These tailored models are revolutionizing how professionals work, dramatically improving outcomes, and creating competitive advantages that were unimaginable just a few years ago. The transformation is happening now, and organizations that embrace these precision-tuned AI systems are setting new standards in their industries. This article explores how domain-specific LLM fine-tuning is redefining excellence across three critical sectors and provides insights into implementation strategies that can position your organization at the forefront of this AI revolution.
The Evolution of LLMs: From General Purpose to Industry Experts
Large Language Models have transformed dramatically since their inception, evolving from interesting research curiosities to powerful business tools. General-purpose LLMs like GPT-4, Claude, and Llama possess impressive capabilities but often lack the specialized knowledge and precision required in highly regulated and technical fields. This knowledge gap creates a significant opportunity for organizations willing to invest in fine-tuning these models for their specific industry needs. Fine-tuning is the process of adapting a pre-trained language model on a smaller, domain-specific dataset to optimize it for particular tasks and terminology. This approach leverages the foundation of general language understanding while adding layers of specialized knowledge that make these models truly useful in professional contexts.
The benefits of industry-specific fine-tuning extend far beyond simple convenience. Fine-tuned models demonstrate measurably higher accuracy when dealing with specialized terminology, follow industry-specific guidelines more consistently, and provide more relevant outputs that professionals can trust. For instance, a healthcare-tuned LLM can recognize medical abbreviations and relate symptoms to potential diagnoses with greater precision than its general-purpose counterpart. Similarly, finance-tuned models can better navigate the complex web of regulations and terminology that defines the industry. The process transforms capable but general AI systems into virtual domain experts that can augment human capabilities in meaningful ways.
Fine-tuning methodologies have advanced significantly in recent years, making the process more accessible and cost-effective for organizations of all sizes. Techniques like parameter-efficient fine-tuning allow companies to achieve impressive results without the computational resources previously required. Transfer learning approaches enable organizations to leverage existing industry-specific models as starting points, further reducing the barriers to adoption. As these techniques continue to evolve, the opportunity to create truly specialized AI assistants is becoming available to a wider range of businesses, democratizing access to this transformative technology.
Healthcare: Precision Medicine Meets Precision AI
The healthcare industry presents unique challenges that make it particularly ripe for specialized LLM applications. Medical professionals work with complex terminology, interpret vast amounts of research, and navigate strict regulatory requirements while delivering personalized patient care. General AI models often struggle with the nuanced language of medicine, potentially leading to dangerous misinterpretations or overly simplified responses. Healthcare-specific fine-tuning addresses these challenges directly by training models on medical literature, clinical notes, treatment protocols, and regulatory documentation. The resulting models speak the language of medicine fluently, understanding context and implications that would elude their general-purpose counterparts.
In clinical settings, fine-tuned LLMs are already transforming workflow efficiency and treatment quality. Physicians use these systems to quickly summarize patient records, highlighting relevant medical history and potential drug interactions before consultations. Radiologists leverage specialized models to draft preliminary reports based on imaging studies, significantly reducing documentation time while maintaining accuracy. Medical researchers employ healthcare-tuned LLMs to rapidly analyze research papers and identify promising treatment approaches for challenging cases. These applications demonstrate tangible benefits including reduced administrative burden, more time for direct patient care, and improved treatment decision support.
The regulatory landscape of healthcare adds another dimension where specialized fine-tuning proves invaluable. Models can be trained to understand HIPAA requirements, clinical trial protocols, and medical coding standards with remarkable precision. This specialized knowledge helps ensure compliance while speeding up documentation processes that traditionally create bottlenecks in healthcare delivery. Organizations like Mayo Clinic and Cleveland Clinic have pioneered the development of proprietary fine-tuned models that understand their specific protocols and documentation standards, creating competitive advantages while improving patient outcomes.
Case studies demonstrate the transformative impact of healthcare-specific LLMs. A major hospital network implemented a fine-tuned model for emergency department triage notes, reducing documentation time by 38% while improving the accuracy of initial assessments. Another healthcare system deployed a specialized model for medication reconciliation, decreasing potential prescription errors by 27% compared to their previous process. These real-world examples highlight how targeted AI capabilities directly translate to improved clinical outcomes and operational efficiency when models truly understand healthcare's unique requirements and terminology.
Finance: Navigating Complexity with AI Precision
The financial sector operates in an environment defined by regulatory complexity, market volatility, and the need for absolute precision in document processing and risk assessment. These characteristics create perfect conditions for specialized LLM applications that can navigate regulatory frameworks and financial terminology with expert-level understanding. Financial institutions are increasingly fine-tuning LLMs on regulatory filings, financial statements, investment research, and internal documentation to create AI assistants that speak the language of finance fluently. These specialized models understand the difference between terms like "principal" in a loan versus a securities context and can navigate the nuances of financial regulations across different jurisdictions.
Risk assessment and regulatory compliance represent particularly promising applications for fine-tuned financial models. Banks and investment firms use specialized LLMs to analyze loan applications, flagging potential compliance issues and assessing risk factors with greater consistency than traditional methods. Compliance departments leverage these models to stay current with evolving regulations, automatically comparing internal policies against new requirements and identifying potential gaps. These applications deliver tangible efficiency gains while potentially reducing the likelihood of costly compliance violations. The models' ability to process and contextualize vast amounts of financial information enables more consistent decision-making across large organizations.
Customer service in financial institutions has also been transformed through specialized fine-tuning. Banks deploy models that understand product offerings, account features, and common customer concerns with exceptional accuracy. These AI assistants can handle increasingly complex queries without human intervention, from explaining fee structures to walking customers through application processes for specific financial products. The specialized knowledge embedded in these models creates more satisfying customer experiences while reducing operational costs. Financial institutions report significantly higher customer satisfaction scores when AI assistants provide accurate, contextually appropriate responses to financial queries.
Leading financial organizations have documented compelling results from their fine-tuning initiatives. A global investment bank reduced document review time for certain compliance processes by 65% after implementing a specialized LLM trained on their regulatory framework and internal policies. A commercial lending institution decreased the time required for initial loan risk assessment by 48% while simultaneously improving the accuracy of their risk categorization. These efficiency gains translate directly to competitive advantages in an industry where speed and accuracy significantly impact bottom-line results. As more financial institutions recognize these benefits, specialized LLM fine-tuning is becoming a critical component of digital transformation strategies throughout the sector.
Manufacturing: Optimizing the Factory Floor with Specialized AI
Manufacturing environments present unique challenges where specialized language models are creating remarkable efficiency improvements. The sector combines technical terminology, complex procedures, regulatory requirements, and the need for precise communication across diverse teams. General-purpose LLMs often lack the specialized vocabulary and contextual understanding required to provide meaningful assistance in these environments. By contrast, manufacturing-specific fine-tuned models trained on technical documentation, quality standards, maintenance procedures, and operational logs can become virtual domain experts that bridge communication gaps and optimize processes throughout the production lifecycle.
Predictive maintenance represents one of the most valuable applications of specialized LLMs in manufacturing. Models fine-tuned on equipment documentation, historical maintenance records, and failure reports can identify potential issues before they cause costly downtime. Maintenance technicians use these systems to troubleshoot complex equipment, with the AI providing targeted recommendations based on specific error codes and observed behaviors. This application dramatically reduces mean time to repair and helps prevent catastrophic failures through early intervention. The financial impact is substantial, with some manufacturers reporting maintenance cost reductions exceeding 30% after implementing these systems.
Quality control processes have been similarly transformed through specialized fine-tuning. Models trained on quality standards, defect patterns, and production specifications help identify potential quality issues and recommend corrective actions. These systems analyze quality testing data, customer feedback, and production parameters to identify correlations that might elude human analysts. The result is more consistent product quality and fewer costly defects reaching customers. Manufacturers implementing these systems report defect rate reductions between 15-25% while simultaneously reducing quality assurance staffing needs. The combination of improved quality and reduced costs creates compelling ROI for these implementations.
Supply chain optimization through specialized LLMs is emerging as another high-value application. Models fine-tuned on supplier documentation, logistics data, and inventory management principles help identify bottlenecks and recommend process improvements. These systems can predict potential supply disruptions based on historical patterns and external factors, enabling proactive mitigation strategies. Manufacturing operations using these tools report inventory reductions averaging 18% while maintaining or improving fulfillment rates. The integration of these specialized LLMs with existing manufacturing systems creates intelligent workflows that continuously optimize operations while adapting to changing conditions.
Statistics & Tables: Industry Impact Comparison
The following interactive table provides a comprehensive comparison of LLM fine-tuning metrics across healthcare, finance, and manufacturing sectors, showcasing the differential impact and adoption patterns by industry.
Conclusion
Industry-specific LLM fine-tuning represents a pivotal advancement in artificial intelligence, transforming general-purpose models into specialized tools that deliver exceptional value across healthcare, finance, and manufacturing sectors. The transition from generic AI solutions to precision-tuned systems marks a crucial evolution โ from interesting technological demonstrations to mission-critical business applications that directly impact operational performance and competitive positioning. Organizations that embrace these technologies are not merely implementing new software; they are fundamentally transforming their operational capabilities and building foundations for sustained competitive advantage in increasingly complex regulatory and market environments.
The implementation patterns and success metrics detailed in this article demonstrate compelling returns across all three industries, though with notable variations in adoption rates and application priorities. Financial services has emerged as the early leader in implementation scale, driven by clear ROI opportunities in document processing and risk assessment. Healthcare shows particularly strong results in documentation efficiency and compliance applications, while manufacturing demonstrates significant value through predictive maintenance and quality control implementations. These patterns reflect the unique challenges and opportunities in each sector, illustrating how fine-tuning adapts general AI capabilities to specific industry requirements.
The path forward for organizations considering industry-specific fine-tuning begins with strategic use case identification, focusing initially on applications that demonstrate clear value while building institutional expertise. Successful implementations typically follow a progressive development model, beginning with targeted applications before expanding to more mission-critical functions. This approach builds organizational confidence while developing the technical and governance frameworks necessary for broader deployment. Organizations should establish clear evaluation metrics that connect model performance to business outcomes, ensuring that implementation priorities align with strategic objectives and demonstrating value to stakeholders throughout the organization.
As fine-tuning technologies continue to advance, the distance between AI capabilities and human expertise in specialized domains will narrow significantly. However, the most successful implementations will not simply replace human judgment but augment it in powerful ways that combine the consistency and scalability of AI with the contextual understanding and ethical reasoning of human experts. The organizations that thrive in this new environment will be those that thoughtfully integrate these technologies into their operations, building new workflows that leverage both human and artificial intelligence to deliver exceptional results. The era of precision AI has arrived โ and through careful implementation of industry-specific fine-tuning, organizations across healthcare, finance, and manufacturing can transform this technological opportunity into sustainable competitive advantage.
Frequently Asked Questions
What is industry-specific LLM fine-tuning?
Industry-specific LLM fine-tuning is the process of adapting pre-trained large language models on specialized datasets relevant to particular sectors like healthcare, finance, or manufacturing. This customization enables the models to understand domain-specific terminology, regulations, and workflows with significantly higher accuracy than general-purpose models.
How much data is typically needed for effective industry-specific fine-tuning?
Effective industry-specific fine-tuning typically requires between 1,000-10,000 high-quality, domain-relevant examples. The exact volume depends on the complexity of the domain and the specificity of the tasks the model will perform, with highly specialized use cases sometimes requiring fewer but more targeted examples.
What ROI can companies expect from implementing fine-tuned LLMs?
Companies implementing fine-tuned LLMs typically see ROI within 4-12 months depending on the industry, with financial services experiencing the fastest returns (4-7 months) due to high transaction volumes and automation potential. Healthcare and manufacturing generally see positive returns within 7-12 months with significant long-term value creation.
How do fine-tuned LLMs address regulatory compliance in healthcare?
Fine-tuned healthcare LLMs address regulatory compliance by incorporating HIPAA requirements, clinical guidelines, and institutional protocols directly into their training data. This enables them to automatically flag potential compliance issues, properly handle PHI, and ensure documentation meets regulatory standards while reducing human oversight requirements.
What are the primary challenges in implementing fine-tuned LLMs in manufacturing?
The primary challenges in implementing fine-tuned LLMs in manufacturing include integrating them with existing operational technology systems, ensuring data security on the factory floor, training models to understand highly specialized equipment terminology, and developing appropriate human-AI collaboration workflows for maintenance and quality control processes.
How do financial institutions ensure security when implementing fine-tuned LLMs?
Financial institutions ensure security when implementing fine-tuned LLMs through strict access controls, on-premises deployment options, comprehensive audit logging, regular security assessments, and fine-tuning specifically on security policies and data handling protocols. Many also implement advanced encryption and tokenization for sensitive financial data processing.
What technical expertise is required to implement industry-specific LLM fine-tuning?
Implementing industry-specific LLM fine-tuning typically requires a team with expertise in machine learning engineering, domain knowledge in the target industry, data engineering for dataset preparation, prompt engineering, and MLOps for deployment and monitoring. Organizations often combine internal domain experts with external AI specialists for optimal results.
How can smaller organizations with limited budgets approach LLM fine-tuning?
Smaller organizations can approach LLM fine-tuning by utilizing parameter-efficient techniques like LoRA or adapter-based methods, starting with smaller base models, focusing on narrow high-value use cases, leveraging open-source models, and considering specialized AI vendors who offer industry-specific solutions with lower implementation barriers.
What metrics should be tracked to measure the success of fine-tuned LLM implementations?
Success metrics for fine-tuned LLM implementations should include task-specific accuracy compared to human performance, time savings for key processes, error reduction rates, user adoption and satisfaction metrics, and financial indicators like cost reductions and revenue impacts. Organizations should establish industry-relevant benchmarks before deployment.
How frequently should industry-specific models be updated or retrained?
Industry-specific models should typically be evaluated quarterly and updated as needed based on performance drift, regulatory changes, terminology evolution, and feedback collection. Healthcare and financial models often require more frequent updates (every 3-6 months) due to regulatory changes, while manufacturing models may remain stable longer if processes don't change.
Additional Resources
For readers interested in exploring industry-specific LLM fine-tuning in greater depth, the following resources provide valuable insights:
Enterprise LLM Integration Strategies - Comprehensive guide to integrating fine-tuned LLMs into existing enterprise architectures across various industries.
Domain Adaptation Methods for LLMs - In-depth exploration of techniques for adapting language models to specific industry domains with optimal performance.
Comprehensive LLM Evaluation Methodologies - Framework for rigorously assessing the performance of fine-tuned models against industry-specific requirements.
Medical LLMs: Development, Evaluation, and Clinical Applications - Specialized resource focused on healthcare applications of fine-tuned language models.
Financial Services AI Implementation Roadmap - Strategic guide for financial institutions implementing AI technologies including fine-tuned LLMs.