Leveraging Artificial Intelligence in Data Integration
Discover how AI is revolutionising data integration by automating processes, enhancing data quality, and providing actionable insights. Learn about real-world use cases and the benefits of AI-driven data integration.
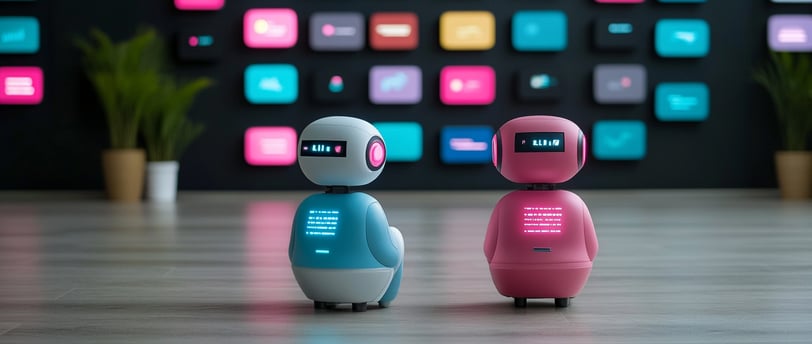
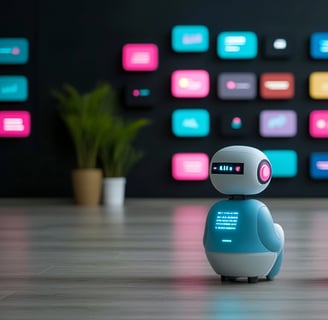
In today's data-driven world, organizations are constantly seeking ways to harness the power of their data more effectively. One of the critical challenges they face is integrating data from diverse sources to gain a unified view and make informed decisions. Traditional data integration methods often fall short, requiring significant manual effort and lacking the scalability and flexibility needed to keep pace with modern data demands. This is where artificial intelligence (AI) steps in, offering transformative solutions that automate and optimize data integration processes.
This article delves into the compelling world of AI-powered data integration. We'll explore how AI technologies are revolutionising data integration, the benefits they offer, and real-world use cases that demonstrate their impact. By the end, you'll understand why AI is becoming indispensable for organizations looking to maximize the value of their data assets.
The Evolution of Data Integration
From Manual to Automated Processes
Data integration has evolved significantly over the years. Initially, it involved manual processes where data was collected, cleaned, and merged from various sources. This approach was time-consuming, error-prone, and could not scale with the growing volume and complexity of data. As technology advanced, automated tools emerged, streamlining some aspects of data integration. However, these tools still required significant human intervention and lacked the intelligence to handle complex data scenarios.
The Advent of AI in Data Integration
The introduction of AI in data integration represents a paradigm shift. AI brings intelligence to the integration process, automating tasks that were previously manual and enhancing the accuracy and reliability of integrated data. AI-driven data integration tools leverage machine learning (ML) algorithms, natural language processing (NLP), and other advanced technologies to handle complex data challenges. These tools can learn from data patterns, adapt to changing data structures, and provide actionable insights, making data integration more efficient and effective.
Benefits of AI-Powered Data Integration
Enhanced Data Quality
One of the most significant benefits of AI-powered data integration is the improvement in data quality. AI algorithms can detect and correct errors, inconsistencies, and duplicates in data, ensuring higher accuracy and reliability. For instance, AI can identify missing values, outliers, and anomalies in data, alerting users to potential issues and suggesting corrections. This capability is crucial for organizations that rely on accurate data for decision-making and operational efficiency.
Automation and Efficiency
AI automates many of the tasks involved in data integration, reducing the need for manual intervention. This automation minimizes errors, speeds up the integration process, and frees up resources for other strategic initiatives. AI-powered tools can handle data extraction, transformation, and loading (ETL) processes, data mapping, and transformation, and even real-time data integration, ensuring that data is always up-to-date and ready for analysis.
Scalability and Flexibility
AI-driven data integration solutions are highly scalable, capable of handling large volumes of data from diverse sources. They can adapt to changing data structures and integration requirements, ensuring seamless integration and performance optimization. This scalability is essential for organizations that need to integrate data from various sources, including databases, cloud platforms, IoT devices, and social media feeds.
Real-Time Data Integration
AI enables real-time data integration, providing up-to-date insights and enabling timely decision-making. AI solutions can process and integrate data as it is generated, ensuring that organizations have access to the latest information. This capability is particularly valuable in fast-paced industries where real-time data is critical for operational efficiency and competitive advantage.
Cost and Resource Optimization
By automating complex data integration tasks, AI reduces the costs and resource requirements associated with data integration. AI-powered solutions can streamline the integration process, making it more accessible to organizations of all sizes. This cost optimization allows even smaller organizations with limited resources to undertake comprehensive data integration initiatives, leveling the playing field in the data-driven landscape.
Real-World Use Cases of AI-Powered Data Integration
Healthcare
In the healthcare industry, AI-powered data integration is transforming patient care and operational efficiency. For example, Mayo Clinic uses AI-enhanced data warehousing to integrate patient records from various sources, enabling comprehensive and accurate medical research. This integration allows healthcare providers to gain a holistic view of patient data, improving diagnosis, treatment, and overall patient outcomes1.
Retail
Retailers are leveraging AI-driven data integration to optimize inventory management, personalize customer experiences, and enhance supply chain operations. Walmart, for instance, uses AI-driven ETL processes to manage and analyze large volumes of sales data, optimizing inventory management and enhancing customer experience. This integration allows Walmart to gain real-time insights into customer preferences and market trends, enabling more effective inventory management and personalized marketing strategies1.
Finance
In the finance sector, AI-powered data integration is revolutionizing risk management, fraud detection, and customer service. Banks and financial institutions use AI to integrate data from various sources, including transaction records, customer profiles, and market data. This integration enables more accurate risk assessments, faster fraud detection, and personalized customer service, improving overall operational efficiency and customer satisfaction.
The Future of AI-Powered Data Integration
The future of AI-powered data integration looks promising, with several emerging trends and technologies set to further enhance its capabilities. Here are some key trends to watch:
Federated Learning
Federated learning is an emerging technology that enables data integration without moving data from its original source. This approach addresses data privacy and security concerns by allowing data to remain in its original location while still enabling integration and analysis. Federated learning is particularly valuable in industries with strict data privacy regulations, such as healthcare and finance2.
Edge Computing
Edge computing is another trend gaining traction in AI-powered data integration. Edge computing involves processing data at the edge of the network, closer to where it is generated. This approach reduces latency, improves real-time data integration, and enhances operational efficiency. Edge computing is particularly valuable in IoT environments, where real-time data processing is critical for operational efficiency and decision-making.
Explainable AI
As AI becomes more integrated into data integration processes, there is a growing need for explainable AI. Explainable AI refers to AI systems that can explain their decisions and actions in a way that is understandable to humans. This transparency is crucial for building trust in AI-driven data integration solutions and ensuring that they are used ethically and responsibly.
Conclusion
AI-powered data integration is transforming how organizations manage and leverage their data assets. By automating processes, enhancing data quality, and providing actionable insights, AI is enabling organizations to gain a competitive edge in the data-driven era. As AI technologies continue to evolve, we can expect even more innovative solutions that will further enhance data integration capabilities, driving operational efficiency and strategic growth.
Embrace the power of AI in data integration and unlock the full potential of your data assets. The future of data integration is here, and it's powered by AI.
FAQ Section
What is AI-powered data integration? AI-powered data integration uses artificial intelligence technologies to automate and optimize the process of combining data from diverse sources. It enhances data quality, improves efficiency, and provides actionable insights.
How does AI enhance data quality in integration processes? AI algorithms can detect and correct errors, inconsistencies, and duplicates in data, ensuring higher accuracy and reliability. AI can also identify missing values, outliers, and anomalies, alerting users to potential issues and suggesting corrections.
What are the benefits of AI-powered data integration? Benefits include enhanced data quality, automation and efficiency, scalability and flexibility, real-time data integration, and cost and resource optimization. AI-driven data integration solutions streamline the integration process, making it more accessible and efficient.
How does AI enable real-time data integration? AI solutions can process and integrate data as it is generated, ensuring that organizations have access to the latest information. This capability is particularly valuable in fast-paced industries where real-time data is critical for operational efficiency and competitive advantage.
What is federated learning, and how does it benefit data integration? Federated learning enables data integration without moving data from its original source, addressing data privacy and security concerns. This approach is particularly valuable in industries with strict data privacy regulations, such as healthcare and finance.
How does edge computing enhance AI-powered data integration? Edge computing involves processing data at the edge of the network, closer to where it is generated. This approach reduces latency, improves real-time data integration, and enhances operational efficiency, particularly in IoT environments.
What is explainable AI, and why is it important in data integration? Explainable AI refers to AI systems that can explain their decisions and actions in a way that is understandable to humans. This transparency is crucial for building trust in AI-driven data integration solutions and ensuring that they are used ethically and responsibly.
What are some real-world use cases of AI-powered data integration? Real-world use cases include healthcare, where AI-enhanced data warehousing improves patient care and medical research; retail, where AI-driven ETL processes optimize inventory management and enhance customer experience; and finance, where AI integrates transaction records for improved operational efficiency and customer satisfaction.
How does AI-powered data integration benefit the healthcare industry? AI-powered data integration in healthcare enables comprehensive and accurate medical research, improves diagnosis and treatment, and enhances overall patient outcomes. It allows healthcare providers to gain a holistic view of patient data, improving operational efficiency and patient care.
How does AI-powered data integration optimize retail operations? AI-powered data integration in retail optimizes inventory management, personalizes customer experiences, and enhances supply chain operations. It enables real-time insights into customer preferences and market trends, improving inventory management and marketing strategies.
Additional Resources
AI in Data Integration: Types, Challenges, and Key AI Techniques Explore the role of AI and ML in data integration and learn how AI in integration transforms how enterprises manage and leverage their valuable data assets3.
How Artificial Intelligence is Revolutionizing Data Integration Discover how AI enhances data lineage, improves data transformation, and enables intelligent data governance in data integration processes4.
Leveraging AI in ETL and Data Warehousing Learn how AI enhances ETL processes by automating complex tasks, improving data quality, and optimizing performance in data warehousing1.