Machine Learning in Cybersecurity: Threat Detection and Response
The world of technology is advancing at an incredible rate, opening up endless possibilities for companies to expand and explore new horizons. One of the most exciting developments is the integration of machine learning into cybersecurity, which allows for rapid threat detection and response.
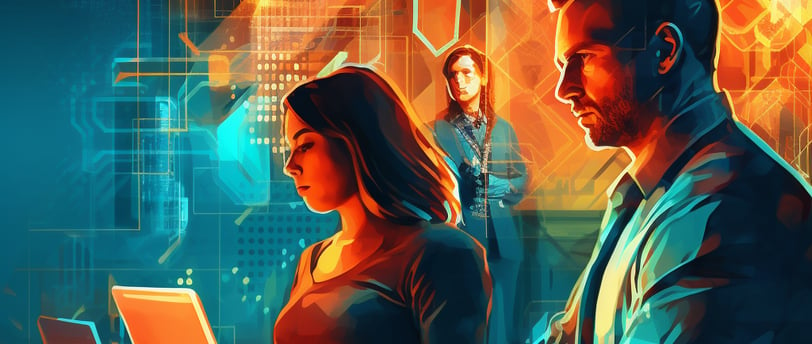
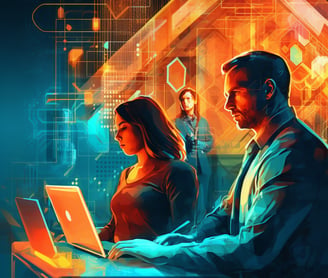
The rapid evolution of the digital world offers businesses vast opportunities for growth and innovation. Yet, this swift progress also introduces significant security challenges. Traditional cybersecurity measures are increasingly falling short in protecting sensitive data and infrastructure against sophisticated cyber threats. In response, businesses are turning to machine learning, an advanced tool in threat detection and response, to bolster their cybersecurity defenses. Machine learning's ability to analyze patterns and predict threats makes it a crucial asset in today's complex cybersecurity landscape.
As a key component of artificial intelligence (AI), machine learning is revolutionizing cybersecurity. It uses algorithms and statistical models to enable computers to learn from data, improving their decision-making and predictive capabilities without direct programming. This adaptability is particularly effective against the evolving nature of cyber threats, which are becoming more intricate and harder to detect. The following exploration delves into the intricacies of machine learning in cybersecurity, examining its critical role, benefits for businesses, and essential insights for organizational success in the digital age. The discussion also covers how Datasumi, a leading data and digital consultancy, aids businesses in leveraging machine learning to strengthen their cybersecurity strategies.
Potential Benefits of Cybersecurity
Organizations can unlock significant business benefits by integrating machine learning into their cybersecurity strategy. Let's explore some of these benefits:
1. Enhanced Threat Detection: Machine learning algorithms can analyze vast amounts of data, including network traffic, user behavior, and system logs, to detect patterns and anomalies that indicate potential threats. This enables organizations to swiftly identify and respond to cyber attacks, reducing the risk of data breaches and minimizing the impact on business operations.
2. Reduced False Positives: Traditional security systems often generate false positives, overwhelming security teams with alerts. Machine learning algorithms can learn from historical data to distinguish between genuine threats and benign activities, significantly reducing false positives and allowing security teams to focus on actual security incidents.
3. Real-time Incident Response: Machine learning algorithms can continuously monitor and analyze network traffic, providing real-time insights into potential security incidents. This enables security teams to respond promptly, mitigating the damage caused by cyber-attacks and minimizing downtime.
4. Adaptive Defense: Cyber threats evolve rapidly, requiring organizations to adapt their defense mechanisms to stay one step ahead. Machine learning models can learn from new data and adjust their detection capabilities to emerging threats. This adaptive defense approach ensures that organizations protect effectively against evolving cyber risks.
5. Operational Efficiency: Machine learning can significantly improve operational efficiency by automating the detection and response processes. Security teams can focus their expertise on analyzing and responding to sophisticated threats, while routine tasks are handled by machine learning algorithms, leading to cost savings and increased productivity.
Key Concerns in Cybersecurity
In the realm of cybersecurity, organizations are confronted with several critical issues that necessitate vigilant and forward-thinking strategies to protect their systems and data. These concerns include:
Advanced Persistent Threats (APTs): APTs represent a severe challenge in cybersecurity. They involve stealthy, long-term infiltrations into networks, where attackers maintain unauthorized access without detection. Traditional detection methods often fall short against APTs due to their sophisticated nature. Machine learning steps in as a potent tool here, aiding in the identification of unusual patterns and behaviors that might signal such threats.
Zero-Day Exploits: These are vulnerabilities in software that are not yet known to the software vendor and consequently lack immediate fixes. Attackers exploit these gaps before they are identified and patched. The agility of machine learning is crucial in these scenarios, as it can scrutinize network traffic and system behaviors to pinpoint such exploits promptly, even in the absence of existing threat signatures.
Insider Threats: Insider threats come from within an organization, often involving authorized individuals who misuse their access to compromise systems. Traditional security systems, which typically rely on predefined rules, struggle to detect subtle anomalies indicative of such threats. However, machine learning algorithms are adept at discerning irregular patterns and deviations in user behavior, thereby enabling early detection and response to insider threats.
Malware Detection: The continuous evolution of malware poses a significant challenge, especially as traditional, signature-based antivirus solutions often fail to catch new or modified strains of malware. Machine learning comes to the rescue by analyzing file characteristics and behaviors, thus significantly improving the detection and identification of new and complex malware forms.
Each of these areas illustrates the dynamic and ever-changing landscape of cybersecurity threats, and the essential role machine learning plays in providing more effective and adaptive defense mechanisms.
Insights Crucial for Success
To effectively integrate machine learning in cybersecurity, organizations must pay attention to several key factors:
Firstly, the success of machine learning models in cybersecurity heavily depends on the quality and quantity of data. These models require extensive, diverse datasets covering a wide range of cyber threats and attack methods for effective training and accurate threat prediction. Therefore, organizations must focus on gathering and curating comprehensive data that reflects various aspects of cybersecurity threats.
Secondly, feature engineering and model selection are critical in the machine learning process. Feature engineering involves identifying and transforming the most relevant data attributes for use in machine learning models. This step is crucial for extracting informative characteristics that can differentiate between normal and malicious activities. Additionally, selecting the appropriate machine learning algorithm is vital. Each algorithm has its own strengths and weaknesses, and choosing the right one for specific cybersecurity challenges is essential. This process also involves rigorous evaluation and validation of the models to ensure they are fit for purpose.
Finally, a collaborative approach between human experts and machine learning algorithms is paramount. While machine learning algorithms are adept at processing vast amounts of data and identifying complex patterns, human analysts bring in-depth contextual understanding and domain-specific knowledge. This synergy enhances the overall effectiveness of cybersecurity threat detection and response, leveraging the strengths of both human insight and machine learning capabilities.
How Datasumi Can Help
Datasumi, recognized for its expertise in data and digital consultancy, plays a pivotal role in empowering businesses to utilize machine learning in their cybersecurity efforts. Their comprehensive services begin with developing a data strategy, ensuring organizations have access to high-quality data essential for training effective machine learning models. Datasumi's proficiency extends to data collection, cleansing, and transformation, thereby aiding businesses in compiling extensive datasets that accurately reflect the variety of cyber threats in the digital world.
Moving beyond data preparation, Datasumi offers tailored machine learning model development. Their deep knowledge in this field allows them to guide businesses in selecting and fine-tuning the most suitable algorithms for their specific cybersecurity requirements, ensuring these models perform optimally. Furthermore, Datasumi's role in model evaluation and validation is crucial. They employ stringent testing methods to ensure the models are effective, accurate, and robust, instilling confidence in the solutions they deploy. In addition to these technical aspects, Datasumi emphasizes the importance of human-machine collaboration in cybersecurity. By integrating the strengths of human analysts with advanced machine learning algorithms, they help organizations establish effective processes and workflows, ensuring a synergistic approach to cybersecurity that leverages the best of both worlds.
Conclusion
As the digital landscape evolves, so do the threats that businesses face. Machine learning has emerged as a game-changing technology in cybersecurity, offering advanced capabilities for threat detection and response. By harnessing the power of machine learning, organizations can enhance their cybersecurity posture, detect threats in real time, and respond swiftly to mitigate potential damage. Datasumi, with its expertise in data analysis and digital consultancy, can guide businesses in leveraging machine learning effectively to fortify their defense against cyber threats. With the right approach and strategic collaboration, organizations can safeguard their data, systems, and reputation in an increasingly complex and challenging cybersecurity landscape."
References
Journal of Big Data. (n.d.). Cybersecurity data science: an overview from a machine learning perspective. Journal of Big Data. Retrieved from https://journalofbigdata.springeropen.com
CrowdStrike. (n.d.). Machine Learning (ML) in Cybersecurity: Use Cases. Retrieved from https://www.crowdstrike.com
Built In. (n.d.). How Machine Learning in Cybersecurity Works. Retrieved from https://builtin.com
NTT. (n.d.). AI and machine learning for threat detection. Retrieved from https://services.global.ntt
PECB. (n.d.). Machine Learning in Cybersecurity: A Proactive Approach. Retrieved from https://pecb.com
EC-Council University. (n.d.). Protecting Against Threats with Machine Learning in Cybersecurity. Retrieved from https://www.eccu.edu
Tuijin Jishu/Journal of Propulsion Technology. (2023). AI in Cybersecurity: Threat Detection and Response with Machine Learning. DOI: https://doi.org/10.52783/tjjpt.v44.i3.237