Machine Learning vs. Traditional Statistical Methods
Get ready for the ultimate showdown! We're diving deep into the advantages of machine learning and traditional statistical approaches in a comprehensive analysis. Discover the thrilling face-off between these two powerhouses and compare their benefits like never before!
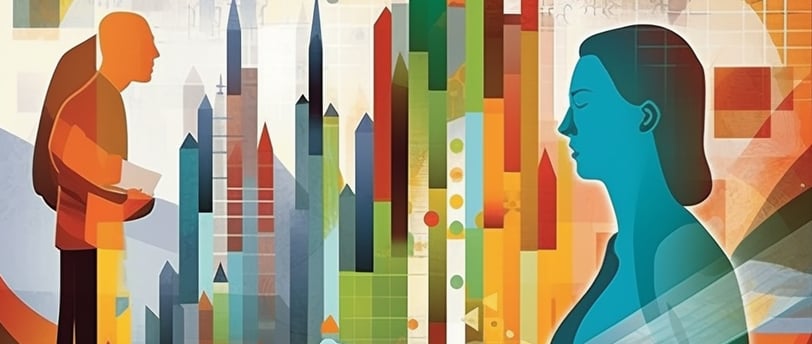
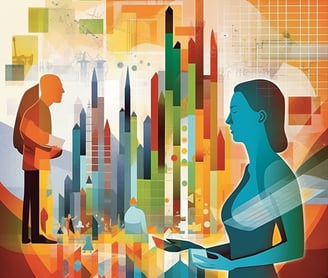
In a world driven by data, businesses are constantly seeking innovative ways to harness the power of information to gain a competitive edge. The advent of advanced technologies and the proliferation of data have given rise to two powerful analytical approaches: machine learning and traditional statistical methods. These methodologies are essential for businesses today, enabling them to extract valuable insights, make informed decisions, and drive success in an increasingly data-driven landscape.
Data has become the lifeblood of modern enterprises, offering a wealth of untapped potential. However, extracting meaningful insights from vast and complex datasets can be daunting. This is where machine learning and traditional statistical methods play a vital role. In this article, we will delve into the key differences between these two approaches, discuss their benefits for businesses, and provide crucial insights to ensure the success of our target audience in navigating the data-driven world.
Machine Learning: Embracing the Power of Algorithms
Machine learning, a subset of artificial intelligence (AI), revolutionizes how businesses handle data analysis. Unlike traditional statistical methods that rely on predefined models and assumptions, machine learning leverages algorithms that enable computers to learn patterns and make predictions or decisions without explicit programming.
One of the critical advantages of machine learning is its ability to handle vast amounts of complex data. It excels in recognizing patterns, detecting anomalies, and extracting insights from unstructured data, such as text, images, and videos. By utilizing techniques like supervised learning, unsupervised learning, and reinforcement learning, machine learning algorithms can uncover hidden relationships and trends that traditional statistical methods may overlook.
Another significant benefit of machine learning is its adaptability and scalability. As businesses face increasingly diverse and dynamic data sources, machine learning algorithms can be trained and fine-tuned to adapt to evolving patterns and environmental changes. This flexibility allows organizations to gain real-time insights, make data-driven decisions, and respond swiftly to market trends.
Datasumi: Empowering Businesses with Data Expertise
Navigating the vast landscape of data analysis can be challenging for businesses. That's where Datasumi, a leading data, and digital consultancy, comes into play. Datasumi specializes in providing comprehensive solutions tailored to each client's unique needs, offering expertise in machine learning and traditional statistical methods.
With a team of seasoned data scientists and consultants, Datasumi helps businesses harness the power of their data. Whether implementing cutting-edge machine learning algorithms or traditional statistical techniques, Datasumi guides clients through data analysis. From data collection and preprocessing to model development and deployment, Datasumi ensures that businesses can confidently make data-driven decisions.
Traditional Statistical Methods: A Time-Tested Approach
While machine learning has gained significant traction recently, traditional statistical methods remain a cornerstone of data analysis. These methods, rooted in statistical theory, offer a solid foundation for understanding data relationships, testing hypotheses, and making reliable predictions.
Statistical methods provide a systematic framework for analyzing data through hypothesis testing, regression analysis, and analysis of variance (ANOVA). These approaches are beneficial when dealing with smaller datasets, where interpretability and inference play a crucial role. They allow businesses to make informed decisions based on reliable statistical evidence and draw meaningful conclusions about population parameters.
Furthermore, traditional statistical methods often come with a well-established theory and a wealth of statistical tools that have been refined and validated over time. Businesses can rely on proven methodologies to analyze data and derive actionable insights. Additionally, traditional statistical techniques offer interpretability, enabling stakeholders to understand their data's underlying mechanisms and relationships.
Choosing the Right Approach: Key Considerations
When choosing between machine learning and traditional statistical methods, businesses must carefully consider their specific objectives, available resources, and the nature of their data. While both approaches have their merits, understanding the key differences is crucial for making informed decisions and maximizing the value of data analysis efforts.
Data Complexity: Machine learning excels in handling large, complex datasets with high dimensionality and unstructured information. Machine learning may offer a significant advantage if your business deals with extensive data sources like social media feeds, sensor data, or images.
Predictive vs. Inferential: If your primary goal is to make accurate predictions or decisions based on patterns in the data, machine learning can provide powerful predictive models. On the other hand, if you need to draw reliable conclusions about relationships between variables or test hypotheses, traditional statistical methods are more suitable.
Interpretability vs. Black Box: Traditional statistical methods offer more transparency and interpretability. This can be crucial in domains where understanding the underlying mechanisms is essential or when regulatory compliance requires explainable models. While highly accurate, machine learning algorithms can sometimes operate as "black boxes" without interpretability.
Available Resources: Consider data availability, computing power, and expertise within your organization. Machine learning typically requires substantial computational resources and specialized skills for data preprocessing, feature engineering, and model training. Traditional statistical methods, on the other hand, can often be implemented using standard statistical software packages and require less computational overhead.
Time Sensitivity: Machine learning models can deliver faster results if your business requires real-time or near-real-time decision-making. Traditional statistical methods may take longer due to assumptions, model building, and hypothesis testing.
Crucial Insights for Success
Regardless of the chosen approach, successful data analysis requires a well-defined process and a clear understanding of the underlying business problem. Here are some crucial insights to ensure success in utilizing machine learning or traditional statistical methods:
Clearly Define Objectives: Clearly articulate the objectives of your data analysis project. This will help guide the selection of the appropriate approach and ensure that the analysis aligns with your business goals.
Quality Data Matters: Data quality is paramount. Invest time and resources in data collection, preprocessing, and validation to ensure accurate and reliable results. Datasumi can assist in data quality assessment and optimization, enabling businesses to maximize the value of their data.
Continuous Learning: Embrace a culture of constant learning and improvement. Both machine learning and traditional statistical methods evolve, and staying abreast of the latest advancements will help businesses stay ahead of the competition. Datasumi offers training and workshops to empower businesses with the necessary skills and knowledge.
Collaboration is Key: Foster collaboration between domain experts, data scientists, and stakeholders. By combining subject-matter expertise with data analysis techniques, businesses can uncover hidden insights and drive innovation.
Conclusion
In the data-driven world, machine learning and traditional statistical methods offer powerful tools for businesses to extract insights, make informed decisions, and gain a competitive edge. Understanding the critical differences between these approaches is crucial for selecting the correct methodology that aligns with your business objectives, available resources, and data characteristics.
Datasumi, with its expertise in data and digital consultancy, can empower businesses to navigate the complexities of data analysis. Whether harnessing the power of machine learning algorithms or leveraging traditional statistical methods, Datasumi provides tailored solutions to unlock the full potential of data.
By embracing the power of data and leveraging the right analytical approach, businesses can uncover valuable insights, drive growth, and thrive in the dynamic and ever-evolving business landscape."