Mastering Multimodal AI & Integrated Intelligence
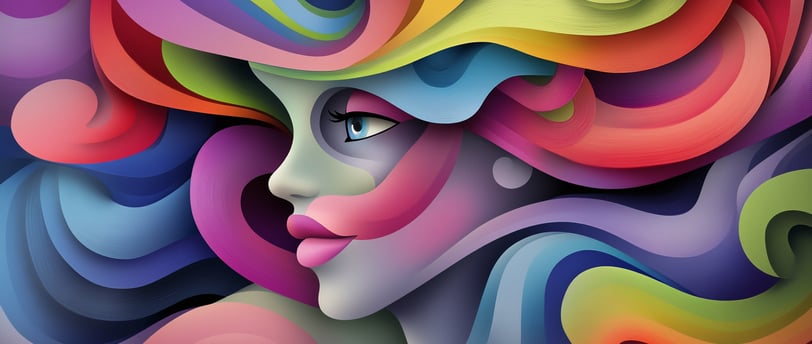
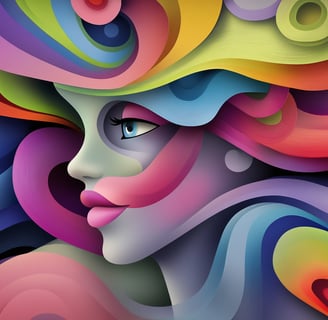
Imagine an AI system that can not only understand and generate text but also interpret images, process audio, and even create videos. This isn't a far-fetched dream but a reality made possible by multimodal AI. In this article, we will delve into the world of multimodal AI, exploring its definitions, applications, benefits, and challenges, and examining how it is reshaping various industries. We will also discuss the future potential of this technology.
Understanding Multimodal AI
Definition and Concept
Multimodal AI refers to AI systems capable of processing and integrating information from multiple modalities or types of data. These modalities can include text, images, audio, video, or other forms of sensory input1. The key to multimodal AI is its ability to combine these different data types to enhance decision-making and context understanding2.
Evolution and Historical Context
The development of multimodal AI has evolved significantly over the years. Early innovations included audio-visual speech recognition and multimedia content indexing. However, it was the advances in deep learning and data science that truly paved the way for the current state of multimodal AI. Today, these systems are used in a variety of applications, from healthcare to autonomous vehicles1.
Key Components
Multimodal AI systems typically consist of several key components:
Input Modules: These components process information from each data type, such as text, images, and audio.
Integration Module: This component combines the inputs from various modalities to create a unified representation.
Output Module: This final component delivers the results based on the integrated inputs3.
Applications of Multimodal AI
Healthcare
In healthcare, multimodal AI is used to analyze medical images alongside textual data from patient records. This integration allows for more accurate diagnostics and personalized treatment plans. For example, AI systems can process MRI scans and corresponding medical notes to detect anomalies and suggest treatment options24.
Autonomous Vehicles
Autonomous vehicles rely on multimodal AI to navigate complex environments. These systems combine visual data from cameras, audio data from microphones, and sensor data to understand and respond to their surroundings. This integration is crucial for safe and efficient autonomous driving2.
Customer Service
Multimodal AI is revolutionizing customer service through chatbots and virtual assistants that can understand and respond to both text and voice inputs. These systems can also process images, allowing customers to upload photos for better support. For example, a customer can send a photo of a damaged product to a chatbot, which then processes the image and provides a solution3.
Security Systems
Security systems use multimodal AI to analyze both video and audio data to detect threats more accurately. By integrating these modalities, security systems can better identify suspicious activities and respond in real-time3.
Content Creation
Multimodal AI is also used in content creation, where systems can generate images from text descriptions or create videos from textual inputs. This application is particularly useful in industries like advertising and entertainment, where creative content is in high demand5.
Benefits of Multimodal AI
Enhanced Accuracy and Robustness
By leveraging different modalities, multimodal AI systems can achieve higher accuracy and robustness in tasks such as image recognition, language translation, and speech recognition. The integration of different types of data helps in capturing more context and reducing ambiguities1.
Improved Contextual Understanding
Multimodal AI systems can provide more detailed and nuanced perceptions of the environment or situation by ingesting and processing data from multiple sources. This improved contextual understanding is crucial for applications like autonomous vehicles and healthcare diagnostics2.
Versatility and Adaptability
Multimodal AI systems are versatile and adaptable, capable of handling a wide range of tasks and applications. This versatility makes them suitable for various industries, from customer service to content creation35.
Challenges and Limitations
Data Integration and Processing
One of the main challenges of multimodal AI is the integration and processing of diverse data types. Ensuring that the system can effectively combine and interpret data from different modalities requires advanced algorithms and significant computational resources2.
Privacy and Bias Concerns
Like any AI system, multimodal AI models can be subject to privacy and bias issues. Collecting and processing diverse datasets from multiple modalities can raise concerns about data privacy and the potential for biased outcomes5.
Technical Complexity
Building and training multimodal AI systems is technically complex. It requires expertise in various fields, including natural language processing, computer vision, and audio processing. Additionally, the development of these systems demands versatile and adaptable annotation tools, which traditional platforms often lack3.
Future Potential
Advancements in Generative AI
The future of multimodal AI lies in the advancements of generative AI, which can produce text, images, video, audio, and other outputs. Generative AI systems may use multimodal training data to develop the ability to input one type of data and output another type of data, enhancing their versatility6.
Integration with Emerging Technologies
As technology advances, multimodal AI is expected to integrate with emerging technologies like augmented reality (AR) and virtual reality (VR). This integration could lead to more immersive and interactive experiences, such as virtual assistants that can understand and respond to both visual and auditory inputs in real-time7.
Personalized and Context-Aware Applications
The ability of multimodal AI to understand and respond to diverse inputs makes it ideal for personalized and context-aware applications. For example, future AI systems could provide personalized recommendations based on a user's preferences, behaviors, and real-time data inputs5.
Conclusion
Multimodal AI represents a significant leap forward in the field of artificial intelligence. By integrating and processing multiple types of data, these systems can provide more accurate, context-aware, and versatile solutions. From healthcare to autonomous vehicles, customer service to content creation, the applications of multimodal AI are vast and growing. As we continue to advance this technology, we can expect even more innovative and personalized AI experiences in the future.
Embrace the future of AI by exploring the possibilities of multimodal AI and its potential to revolutionize your industry.
Frequently Asked Questions (FAQ)
What is multimodal AI? Multimodal AI refers to AI systems that can process and integrate information from multiple modalities, such as text, images, audio, and video.
How does multimodal AI differ from traditional AI? Traditional AI systems typically focus on a single data type, while multimodal AI integrates and analyzes several different forms of data to generate more comprehensive insights.
What are the key components of a multimodal AI system? The key components include input modules for processing different data types, an integration module for combining these inputs, and an output module for delivering results.
What are some applications of multimodal AI in healthcare? Multimodal AI is used in healthcare for analyzing medical images alongside textual data from patient records, leading to more accurate diagnostics and personalized treatment plans.
How does multimodal AI enhance autonomous vehicles? Autonomous vehicles use multimodal AI to combine visual data from cameras, audio data from microphones, and sensor data to understand and respond to their surroundings safely.
What are the benefits of multimodal AI in customer service? Multimodal AI enhances customer service through chatbots and virtual assistants that can understand and respond to both text and voice inputs, providing personalized support.
What are the challenges of implementing multimodal AI systems? Challenges include data integration and processing, privacy and bias concerns, and the technical complexity of building and training these systems.
How does multimodal AI improve contextual understanding? By ingesting and processing data from multiple sources, multimodal AI systems can provide more detailed and nuanced perceptions of the environment or situation.
What is the future potential of multimodal AI? The future of multimodal AI lies in advancements in generative AI, integration with emerging technologies like AR and VR, and the development of personalized and context-aware applications.
What are some examples of multimodal AI in content creation? Multimodal AI is used in content creation to generate images from text descriptions or create videos from textual inputs, enhancing creativity and versatility.
Additional Resources
Author Bio
Alex Turner is a data scientist and AI enthusiast with a background in computer science and engineering. He has been working in the field of artificial intelligence for over a decade, focusing on the development and implementation of multimodal AI systems.