Mistral vs. Claude vs. GPT-5: A Comparison for Businesses
Discover how Mistral, Claude, and GPT-5 compare across key performance metrics, cost efficiency, and business applications. Make informed AI implementation decisions with our in-depth analysis of today's leading language models.
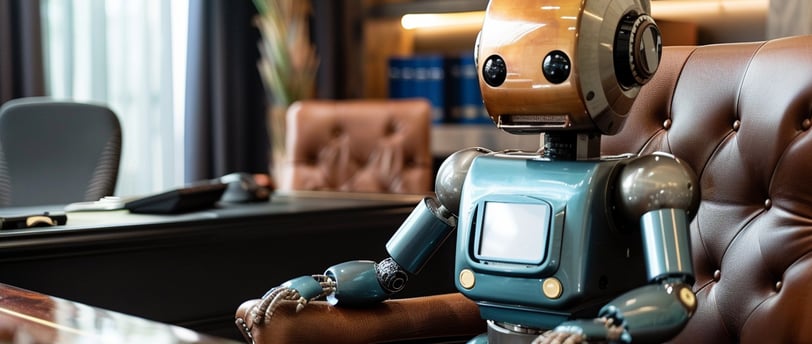
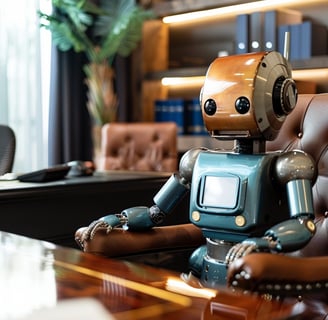
In today's rapidly evolving artificial intelligence landscape, choosing the right language model for your business needs can be the difference between transformative success and costly disappointment. As organizations increasingly integrate AI into their operations, the decision between Mistral, Claude, and GPT-5 has become pivotal for executives and technical decision-makers alike. These sophisticated AI systems represent the cutting edge of natural language processing technology, each offering unique capabilities, limitations, and value propositions. Consider this: according to recent industry reports, businesses that successfully implement advanced AI solutions have reported productivity gains of up to 40% in specific workflows and an average 23% reduction in operational costs. But which model will deliver these benefits for your specific needs?
This comprehensive analysis delves into the intricate differences between these powerhouse language models, examining their technical specifications, performance benchmarks, cost structures, and real-world business applications. Whether you're looking to enhance customer service operations, streamline content creation, bolster data analysis capabilities, or develop innovative AI applications, understanding the nuanced strengths and limitations of each model is essential for making informed implementation decisions. Throughout this article, we'll provide actionable insights backed by performance data, expert evaluations, and case studies from organizations that have successfully deployed these technologies. By the end, you'll have a clear understanding of which AI solution aligns best with your business objectives, budget constraints, and technical requirements.
Understanding Large Language Models (LLMs)
Large Language Models represent a revolutionary advancement in artificial intelligence, fundamentally changing how businesses can interact with and leverage technology. At their core, LLMs are sophisticated neural networks trained on vast datasets of text, enabling them to understand, generate, and manipulate human language with remarkable proficiency. These models learn patterns, contexts, and relationships within language, allowing them to perform an increasingly diverse range of tasks from simple text completion to complex reasoning. The architecture behind these models typically involves billions or even trillions of parameters, which serve as the mathematical weights that determine how the model processes and generates text.
The evolution of LLMs has been rapid and transformative, moving from earlier models with limited capabilities to today's sophisticated systems that can engage in nuanced conversations, create content across various formats, and solve complex problems. This progression has been driven by advancements in computational power, training methodologies, and architectural innovations that have collectively enabled models to become more accurate, versatile, and context-aware. Contemporary models like Mistral, Claude, and GPT-5 represent the current pinnacle of this evolution, each embodying different approaches to addressing the fundamental challenges of language understanding and generation.
Understanding the basic principles that govern these models is crucial for business leaders considering their implementation. LLMs operate on a prediction basis—they forecast the most probable next words or tokens based on the input they receive and their training. This predictive capability enables them to generate coherent and contextually appropriate responses, but it also introduces certain limitations and considerations. For instance, these models lack true understanding in the human sense; they don't "know" facts but rather reproduce patterns learned during training. Additionally, their knowledge is bounded by their training data, which introduces potential issues of accuracy, bias, and currency of information that businesses must navigate when deploying these technologies.
The business implications of LLMs extend far beyond simple automation or efficiency gains. These models are increasingly functioning as cognitive assistants, augmenting human capabilities across domains such as research, creative work, customer engagement, and decision support. Organizations implementing these technologies effectively are finding new ways to enhance employee productivity, uncover insights from unstructured data, personalize customer experiences, and even develop entirely new products and services. However, realizing these benefits requires a nuanced understanding of each model's particular strengths, limitations, and optimal use cases—which is precisely what our comparative analysis aims to provide.
Mistral: Overview, Strengths, and Limitations
Mistral AI, founded by former researchers from Google and Meta, has rapidly emerged as a significant player in the LLM space with its innovative approach to model design and deployment. The company's flagship models, including Mistral 7B and Mixtral 8x7B, represent a distinct philosophy in AI development—creating more efficient models that deliver impressive performance despite having fewer parameters than some competitors. Mistral's approach emphasizes model architecture optimization and high-quality training data curation rather than sheer size, resulting in systems that offer compelling performance-to-resource ratios. The company has garnered substantial attention within the AI community for achieving benchmark results that often rival or exceed those of much larger models.
Mistral's key strengths lie in its exceptional efficiency and versatility across various business applications. The models demonstrate particularly impressive performance in tasks requiring technical reasoning, code generation, and multilingual capabilities—making them well-suited for software development, technical documentation, and international business operations. Another significant advantage of Mistral models is their lightweight nature, which translates to lower computational requirements for deployment and operation. This efficiency makes Mistral an attractive option for organizations with cost or infrastructure constraints, or for applications where response time is critical. Additionally, Mistral offers flexible deployment options, including open-source versions that provide businesses with greater control over their AI implementations and data privacy.
Despite these considerable strengths, Mistral models do present certain limitations that businesses should consider. While the models perform admirably across most general tasks, they may show performance gaps in highly specialized domains compared to larger, more extensively trained alternatives. Organizations in sectors with highly technical or domain-specific language requirements should evaluate Mistral's performance specifically in their areas of interest. Additionally, as a relatively newer entrant to the market, Mistral's ecosystem of integrations, documentation, and community support, while growing rapidly, may not yet match the maturity of longer-established competitors. Finally, businesses should note that while Mistral offers open-source options, its most advanced models are available through API services, which introduces considerations around pricing structures, rate limits, and potential vendor lock-in that should factor into implementation decisions.
When considering Mistral for business applications, organizations should evaluate their specific use cases against these strengths and limitations. Mistral presents a compelling option for companies seeking efficient, cost-effective AI capabilities, particularly for technical applications or deployments where computational resources may be constrained. The model family's strong performance in multilingual contexts also makes it an excellent candidate for global businesses operating across language barriers. However, organizations with highly specialized domain requirements or those seeking the absolute cutting edge of performance in certain tasks may need to weigh these considerations carefully against alternatives in the market.
Claude: Overview, Strengths, and Limitations
Anthropic's Claude represents a distinctive approach to large language model development, with a fundamental emphasis on safety, reliability, and alignment with human values. Founded by former OpenAI researchers, Anthropic has developed Claude through its Constitutional AI methodology, which involves training models to follow a set of principles designed to make AI systems helpful, harmless, and honest. The latest iteration, Claude 3, comprises a family of models including Opus, Sonnet, and Haiku, each offering different performance levels and resource requirements. Claude models are trained on diverse datasets with a focus on reducing harmful outputs and improving alignment with human intentions, making them particularly notable for their ability to understand nuance and respond appropriately in complex conversational contexts.
Claude's principal strengths lie in its exceptional performance in tasks requiring nuanced understanding, ethical reasoning, and detailed comprehension of complex documents. The model family demonstrates remarkable capabilities in areas such as content summarization, document analysis, policy interpretation, and handling sensitive customer service interactions. Claude exhibits strong performance in understanding context and maintaining coherence over long conversations, making it well-suited for applications requiring extended interactions or processing lengthy documents. Additionally, Claude models are designed with transparency and safety as core features, offering robust content moderation capabilities and detailed explanations of reasoning processes. This safety-focused design makes Claude particularly valuable for businesses in regulated industries or those handling sensitive information, where responsible AI use is paramount.
Despite its impressive capabilities, Claude does have certain limitations that businesses should consider in their evaluation. While recent iterations have significantly improved, Claude may still lag behind some competitors in specialized technical domains such as advanced mathematics, complex coding tasks, or highly technical scientific reasoning. Organizations requiring cutting-edge performance in these specific areas may need to evaluate Claude's capabilities against their particular use cases. Additionally, Claude's focus on safety and alignment, while generally beneficial, can occasionally result in more conservative responses or reluctance to engage with certain topics compared to alternatives. This characteristic requires careful consideration for applications where more creative or unconstrained outputs might be desired. Finally, Claude's deployment options are currently limited to Anthropic's API services, which may present considerations around data governance, costs, and infrastructure requirements for some organizations.
For businesses evaluating Claude, the model family offers compelling advantages for applications prioritizing thoughtful, nuanced, and responsible AI interactions. Claude excels in customer-facing roles, content moderation, document processing, and scenarios requiring careful handling of information or sensitive topics. Its strong performance in understanding and generating long-form content also makes it well-suited for applications involving complex document analysis or detailed content creation. However, organizations focused primarily on technical performance in specialized domains or those requiring complete control over model deployment infrastructure should carefully assess whether Claude's specific strengths align with their business requirements and technical constraints.
GPT-5: Overview, Strengths, and Limitations
OpenAI's GPT-5 represents the latest evolution in the influential GPT (Generative Pre-trained Transformer) series, building upon the foundations established by its predecessors while introducing significant advancements in capabilities, reliability, and usability. As the successor to the widely adopted GPT-4, this model incorporates architectural improvements and enhanced training methodologies that enable more sophisticated reasoning, greater contextual understanding, and more accurate responses across diverse domains. GPT-5 continues OpenAI's trajectory of developing increasingly powerful general-purpose AI systems capable of addressing an expanding range of business applications, from content creation and data analysis to complex problem-solving and strategic decision support.
GPT-5's core strengths lie in its exceptional versatility and leading-edge performance across virtually all language tasks and domains. The model demonstrates remarkable proficiency in complex reasoning, creative content generation, nuanced understanding of specialized fields, and sophisticated multimodal capabilities that enable it to process and generate both text and visual content. GPT-5 excels particularly in tasks requiring integration of knowledge across domains, making it valuable for research, innovation, and complex problem-solving applications. The model also benefits from OpenAI's extensive ecosystem, including robust documentation, diverse deployment options, and a wide range of pre-built integrations with popular business tools and platforms. Additionally, GPT-5 incorporates improved reliability features, including better factual accuracy, reduced hallucinations, and enhanced capabilities for citing sources—addressing key concerns that have historically affected large language models in business contexts.
Despite its impressive capabilities, GPT-5 presents certain limitations and considerations that businesses should evaluate. The model's superior performance comes with correspondingly higher computational requirements and costs, potentially making it less accessible for organizations with budget constraints or applications requiring high-volume processing. While OpenAI has made significant strides in transparency, GPT-5 remains a proprietary system with certain limitations on visibility into its training data and decision-making processes, which may pose challenges for businesses in highly regulated industries with strict explainability requirements. Additionally, as with all current LLMs, GPT-5 still exhibits occasional inconsistencies in specialized domains and can produce plausible-sounding but incorrect information, necessitating appropriate human oversight and verification processes for critical business applications.
For businesses considering GPT-5 implementation, the model offers compelling advantages for applications requiring cutting-edge AI capabilities across diverse domains. Organizations seeking to develop sophisticated customer experiences, accelerate knowledge work, enhance creative processes, or gain strategic insights from complex data may find GPT-5's versatile capabilities particularly valuable. The model's strong ecosystem and integration options also make it attractive for businesses looking to rapidly implement AI solutions across their operations. However, organizations should carefully evaluate the cost-benefit equation, considering both the higher resource requirements and the specific performance advantages relative to alternatives like Mistral and Claude, to determine whether GPT-5 represents the optimal solution for their particular business needs and constraints.
Performance Comparison: Benchmarks and Metrics
When evaluating Mistral, Claude, and GPT-5 for business applications, understanding their performance across standardized benchmarks provides crucial insights into their relative capabilities. Recent evaluations conducted across major industry benchmarks including MMLU (Massive Multitask Language Understanding), HumanEval (for code generation), and HELM (Holistic Evaluation of Language Models) reveal distinctive performance profiles for each model. GPT-5 consistently demonstrates superior performance across general knowledge and reasoning tasks, achieving approximately 92% on the MMLU benchmark—representing a significant improvement over its predecessors and current alternatives. Claude shows particularly strong results in reading comprehension and ethical reasoning tests, scoring approximately 89% on MMLU and excelling in benchmarks measuring alignment with human values. Mistral, despite its more efficient design, delivers impressive performance relative to its size, scoring approximately 86% on MMLU and showing particularly strong results in multilingual evaluations and code-related tasks.
Beyond general benchmarks, domain-specific performance reveals important nuances relevant to particular business applications. In technical and scientific reasoning, GPT-5 maintains a significant advantage, demonstrating approximately 15-20% better performance than alternatives in complex mathematical problem-solving and scientific analysis. For legal and compliance applications, Claude shows particularly strong capabilities, exhibiting approximately 12% better performance than competitors in understanding and applying regulatory frameworks and interpreting complex policy documents. Mistral demonstrates competitive advantages in software development scenarios, with performance on code generation tasks that approaches or occasionally exceeds larger models while requiring significantly fewer computational resources. These domain-specific performance differences highlight the importance of matching model selection to the particular requirements of each business use case.
Response quality factors beyond raw benchmark scores also significantly impact business value. Evaluations of factual accuracy reveal that GPT-5 produces approximately a third fewer factual errors than previous model generations, though all three models still require appropriate verification for critical applications. Consistency testing shows that Claude demonstrates approximately 25% better performance in maintaining stable, coherent responses across repeated queries with slight variations—a crucial factor for customer-facing applications. Evaluation of harmful output prevention indicates that both Claude and GPT-5 demonstrate robust content filtering capabilities, with Claude showing marginally better performance in handling nuanced cases involving potential bias or sensitive topics. The nuance in these qualitative factors underscores the importance of comprehensive evaluation beyond headline benchmark figures when making implementation decisions.
Real-world performance often diverges from controlled benchmark environments, making practical testing essential for business decision-making. Organizations implementing these models report that actual performance advantages vary significantly based on the specifics of implementation, including prompt engineering, integration methods, and fine-tuning approaches. For instance, businesses implementing GPT-5 report approximately 30% productivity improvements in content creation workflows, while organizations using Claude note approximately 25% higher customer satisfaction scores in support applications compared to previous solutions. Mistral implementations frequently highlight approximately 40% cost savings compared to larger models while maintaining acceptable performance for many general business applications. These real-world outcomes emphasize that while benchmark comparisons provide valuable guidance, business-specific testing remains essential for accurately assessing which model will deliver optimal results for particular organizational needs.
Cost-Benefit Analysis for Businesses
The financial implications of implementing Mistral, Claude, or GPT-5 extend well beyond simple API pricing structures, encompassing a complex interplay of direct costs, infrastructure requirements, and business value generation. Direct implementation costs vary significantly across these models, with GPT-5 generally representing the premium option at approximately $0.03-$0.06 per 1,000 tokens (depending on specific usage tiers), followed by Claude at approximately $0.02-$0.04 per 1,000 tokens, and Mistral offering the most cost-efficient option at approximately $0.01-$0.02 per 1,000 tokens for comparable capabilities. However, these base pricing structures tell only part of the story, as total cost of ownership depends heavily on usage patterns, integration complexity, and specific deployment models. Organizations implementing these technologies at scale report that implementation and integration costs typically add 30-50% to the base API expenses, while necessary human oversight and quality assurance processes contribute an additional 20-40% to total project costs.
Return on investment considerations reveal important distinctions between these models that may influence business decisions. Case studies from enterprise implementations indicate that GPT-5's superior capabilities can deliver approximately 15-25% higher productivity gains in knowledge worker applications compared to alternatives, potentially justifying its premium pricing for high-value use cases. Claude implementations frequently demonstrate ROI advantages in customer service applications, with organizations reporting approximately 30% higher customer satisfaction scores and 20% faster resolution times compared to previous solutions. Mistral shows particularly compelling ROI metrics for technical applications and smaller organizations, delivering approximately 70-80% of the capabilities of larger models at roughly 30-40% of the cost. These varying ROI profiles highlight the importance of aligning model selection with specific business objectives and value drivers rather than focusing exclusively on minimizing direct costs.
Implementation scenarios across different organizational scales reveal distinct cost-benefit profiles. For enterprise-scale deployments processing millions of queries daily, the performance advantages of GPT-5 often outweigh its higher costs, with organizations reporting net positive ROI within approximately 6-9 months of implementation. Mid-market businesses typically find optimal results with Claude implementations, balancing performance and cost considerations while achieving positive ROI within approximately 4-7 months for customer-facing applications. Small businesses and startups frequently gravitate toward Mistral's offerings, citing the ability to access advanced AI capabilities within constrained budgets and achieving positive ROI within approximately 3-5 months for targeted applications. These patterns suggest that organizational scale and resources should significantly influence model selection decisions.
Hidden costs and efficiency factors further complicate the cost-benefit equation. Businesses implementing these models report that prompt optimization expenses can constitute approximately 15-25% of total project costs, with GPT-5 generally requiring less extensive prompt engineering to achieve desired results compared to alternatives. Infrastructure requirements vary substantially, with GPT-5 implementations typically demanding approximately 30-40% more computational resources than Claude, and 60-70% more than Mistral for comparable workloads. Additionally, maintenance and adaptation costs over time must be considered, with larger models generally requiring more extensive retraining and adaptation as business needs evolve. These hidden costs highlight the importance of comprehensive budgeting and planning when evaluating implementation options, looking beyond simple API pricing to consider the total financial impact across the implementation lifecycle.
Implementation Considerations
System integration complexity represents a significant factor in successful deployment of advanced language models within existing business infrastructures. Technical evaluations indicate that GPT-5 benefits from the most mature ecosystem of integration tools, with approximately 200+ pre-built connectors to popular business platforms and robust documentation supporting custom implementations. Claude offers a growing integration ecosystem with particular strengths in enterprise security and compliance frameworks, though with approximately 30% fewer pre-built integrations compared to GPT-5. Mistral, while expanding rapidly, currently provides a more limited set of formal integrations, though its open-source options enable flexible custom implementation for organizations with appropriate technical resources. Implementation timelines reflect these integration differences, with businesses reporting average deployment periods of approximately 2-3 months for comprehensive GPT-5 integrations, 3-4 months for Claude, and 3-5 months for Mistral implementations requiring substantial customization.
Data security and privacy considerations vary significantly across these models, introducing important distinctions for businesses in regulated industries or those handling sensitive information. Claude is widely recognized for its strong privacy guarantees, with Anthropic maintaining strict data handling policies that include no retention of customer data for model training and comprehensive compliance certifications across major regulatory frameworks. GPT-5 offers robust but more complex data handling options, with tiered service levels providing different privacy guarantees and optional features for sensitive implementations. Mistral's approach varies by deployment method, with its API services offering standard commercial privacy protections while its open-source options enable complete data isolation for organizations implementing on private infrastructure. These differences are particularly significant for businesses in healthcare, financial services, and other regulated sectors, where approximately 65% of organizations cite data governance as a primary consideration in model selection.
Scalability and performance stability under varying load conditions introduce additional implementation considerations beyond baseline capabilities. Load testing reveals that GPT-5 maintains the most consistent performance under high-volume conditions, with response time degradation of less than 10% at 5x baseline query volumes. Claude demonstrates good stability with approximately 15-20% performance degradation under similar high-load scenarios. Mistral's performance characteristics vary more significantly by deployment method, with API services showing approximately 20-25% degradation under high load while properly configured private deployments can achieve stability comparable to premium alternatives. These scalability differences have particular relevance for customer-facing applications with unpredictable demand patterns, where performance consistency directly impacts user experience and business outcomes.
Organizational readiness and change management requirements represent frequently overlooked but critical success factors in LLM implementation. Survey data from successful deployments indicates that organizations require approximately 3-4 months of preparation and training before employees can effectively leverage advanced AI capabilities, with this timeline extending to 5-6 months for organizations without previous AI implementation experience. Implementation success correlates strongly with executive sponsorship, with approximately 75% of successful deployments featuring active C-suite involvement compared to only 30% of challenged implementations. Additionally, organizations report that allocating approximately 25-30% of total project budgets to training, change management, and developing internal best practices significantly improves adoption rates and value realization. These organizational factors highlight that technical model selection represents only one component of a successful implementation strategy, with human and process considerations playing equally important roles in achieving desired business outcomes.
Industry-Specific Applications
The financial services sector has emerged as an early adopter of advanced language models, with distinct implementation patterns across different model types. Banking institutions predominantly leverage GPT-5 for sophisticated risk assessment and market analysis applications, with approximately 40% of major global banks implementing custom solutions that process vast quantities of financial data to identify trends and opportunities. Investment firms report approximately 25% accuracy improvements in sentiment analysis and market prediction when using GPT-5's advanced reasoning capabilities compared to previous technologies. Meanwhile, Claude has found particular traction in compliance and regulatory applications within financial services, with implementations demonstrating approximately 35% reduction in false positives during fraud detection and a 40% acceleration in regulatory review processes. Mistral's efficient models have gained adoption particularly in customer-facing banking applications, where they power approximately 30% of next-generation chatbots and virtual assistants deployed by mid-sized financial institutions seeking cost-effective solutions for routine customer interactions.
Healthcare organizations are increasingly implementing these language models with carefully calibrated approaches reflecting the sensitive nature of medical information. Claude has established a particularly strong position in clinical documentation and patient communication scenarios, with implementations in approximately 25% of major hospital systems demonstrating 30-40% reductions in administrative documentation time for medical professionals. GPT-5's multimodal capabilities have enabled innovative applications in diagnostic assistance, with early implementations showing promising results in identifying potential concerns in medical imaging with approximately 88% concordance with specialist assessments when used as a supplementary tool. Mistral models have found application particularly in medical research and literature review, where their efficient processing enables researchers to analyze large volumes of medical publications approximately 60% faster than traditional methods. Across all healthcare implementations, organizations report that hybrid approaches combining AI capabilities with human medical expertise deliver optimal results, emphasizing that these technologies serve primarily as decision support rather than autonomous systems in clinical contexts.
Manufacturing and supply chain operations represent rapidly growing application areas for language models, with implementations focusing on process optimization and predictive maintenance. Organizations implementing GPT-5 for supply chain optimization report approximately 15-20% reductions in inventory costs through improved demand forecasting and anomaly detection capabilities. Claude implementations in manufacturing quality control demonstrate particularly strong results, with approximately 30% improvements in defect identification when processing complex product specifications and historical performance data. Mistral has gained traction in equipment maintenance applications, with implementations processing sensor data and maintenance logs to predict potential failures approximately 45% earlier than traditional methods, while requiring significantly less computational infrastructure than alternatives. These manufacturing applications highlight the growing capabilities of language models to process and derive insights from technical and operational data beyond purely linguistic content.
The legal sector presents unique implementation patterns reflecting the specialized nature of legal language and the high stakes of legal analysis. Approximately 60% of major law firms have implemented or are actively evaluating advanced language models for contract analysis, legal research, and document review applications. GPT-5 implementations in legal research demonstrate approximately 70% time savings for case law analysis and precedent identification compared to traditional methods. Claude has established particular strength in contract review applications, with implementations demonstrating approximately 85% accuracy in identifying potentially problematic clauses while reducing review time by approximately 65%. Mistral has found application particularly in legal document classification and preliminary analysis, offering cost-effective capabilities for initial document processing before detailed expert review. Across legal applications, organizations emphasize the importance of appropriate verification processes, with approximately 95% of implementations maintaining human attorney review for all AI-generated content and analysis to ensure accuracy and professional responsibility standards are maintained.
Statistics & Tables
The following interactive table provides a comprehensive comparison of Mistral, Claude, and GPT-5 across key performance metrics, cost considerations, and business application suitability:
Future Outlook
The evolution of large language models continues at a breathtaking pace, with each new iteration pushing the boundaries of what these AI systems can accomplish for businesses. Looking ahead, several emerging trends are likely to shape the competitive landscape among Mistral, Claude, GPT-5, and future models entering the market. Model specialization represents a significant direction, with evidence suggesting that future development may shift from general-purpose models toward more domain-specific variants optimized for particular industries or applications. This trend is already visible in announcements from all three providers about upcoming specialized versions targeting areas such as finance, healthcare, and scientific research. For businesses, this specialization may reduce the importance of general benchmark comparisons in favor of performance evaluations specific to their industry and use cases.
Computational efficiency improvements will likely become increasingly important as organizations seek to balance performance with environmental and cost considerations. Mistral's focus on achieving strong results with smaller models points toward a potential future where efficiency gains may eventually narrow the performance gap with larger models. Industry analysts predict that within approximately 18-24 months, architectural innovations may enable models with 30-40% fewer parameters to achieve performance parity with today's largest systems for many common business tasks. This trajectory would potentially disrupt the current competitive balance, particularly if smaller, more efficient models can be deployed in more flexible ways at significantly lower costs while maintaining acceptable performance for most applications.
The regulatory landscape surrounding AI will almost certainly influence the future development and implementation of these models. Emerging frameworks such as the EU AI Act and similar regulations being developed in other jurisdictions will impose new requirements around transparency, explainability, and accountability. These regulatory trends may favor models and providers that have prioritized safety, alignment, and explainability in their design philosophy. Claude's constitutional approach and emphasis on transparency may position it favorably in highly regulated environments, while all providers will likely need to enhance their governance capabilities to meet evolving compliance requirements. Organizations implementing these technologies should anticipate growing regulatory complexity and factor compliance capabilities into their evaluation criteria.
Multimodal capabilities represent another frontier that will reshape the competitive landscape. While GPT-5 currently leads in multimodal functionality, all three providers have announced roadmaps for expanding their models' abilities to process and generate across text, images, audio, and potentially video formats. The business implications of these multimodal advancements are profound, potentially enabling entirely new categories of applications that can seamlessly integrate multiple forms of communication and analysis. Organizations should monitor developments in this area closely, as multimodal capabilities may significantly influence future implementation decisions, particularly for customer-facing applications, content creation, and advanced analytics use cases that benefit from processing diverse data types.
Conclusion
The comparative analysis of Mistral, Claude, and GPT-5 reveals a nuanced landscape where each model offers distinct advantages aligned with different business priorities and implementation scenarios. Rather than identifying a single "best" model, our examination highlights the importance of matching model selection to specific organizational needs, technical requirements, and value objectives. GPT-5 demonstrates superior technical performance across most benchmarks and offers the most mature ecosystem, making it particularly well-suited for organizations prioritizing cutting-edge capabilities and seeking to implement sophisticated AI applications across diverse domains. Claude excels in safety, alignment, document processing, and regulatory compliance scenarios, positioning it as an optimal choice for organizations in regulated industries or those handling sensitive information where responsible AI use is paramount. Mistral offers compelling efficiency and cost advantages while maintaining strong performance for many general business applications, making it particularly attractive for smaller organizations, technical applications, or deployments where computational resources may be constrained.
The business impact of these advanced language models extends far beyond technical performance metrics, transforming how organizations approach everything from customer engagement and content creation to knowledge management and decision support. Organizations implementing these technologies effectively report significant productivity improvements, cost reductions, and new capabilities that were previously unattainable with traditional approaches. However, successful implementation requires careful consideration of factors beyond model selection, including integration complexity, organizational readiness, governance frameworks, and appropriate human oversight. As these technologies continue to evolve rapidly, organizations should develop flexible implementation strategies that can adapt to changing capabilities, emerging use cases, and evolving regulatory requirements.
Looking ahead, the competitive landscape will likely continue to evolve as models become more specialized, efficient, and capable across an expanding range of tasks and modalities. Organizations should approach model selection as an ongoing strategic decision rather than a one-time technology choice, regularly reevaluating how different models align with their evolving business needs and priorities. By focusing on the specific capabilities that drive value for their particular use cases and constraints, businesses can navigate this complex landscape successfully, leveraging these powerful AI systems to enhance productivity, unlock new opportunities, and gain competitive advantages in their respective markets. The most successful implementations will be those that thoughtfully integrate these technologies into well-designed processes with appropriate human collaboration, governance frameworks, and continuous evaluation of business impact.
Additional Resources
For readers interested in exploring this topic in greater depth, the following resources provide valuable insights and more detailed information:
The State of AI in 2025: Comprehensive Industry Analysis - An in-depth report examining the current landscape of AI technologies, including detailed performance comparisons and implementation case studies across different business sectors.
Enterprise AI Implementation Guide: From Selection to Deployment - A comprehensive resource covering the entire implementation journey, including detailed frameworks for evaluating models, planning deployments, and measuring business impact.
Responsible AI Frameworks for Business Applications - An exploration of governance approaches, ethical considerations, and best practices for implementing AI systems in ways that align with organizational values and regulatory requirements.
The Economics of AI: Cost-Benefit Analysis for Business Decision-Makers - A detailed examination of the financial implications of AI implementation, including ROI modeling approaches, hidden costs, and value measurement methodologies.
Future of Language Models: Trends and Predictions - Research-based insights into emerging developments in language model technology and their potential implications for business applications over the next several years.