Multimodal GenAI in Healthcare: Elevating Patient Experience and Clinical Insights
Transforming healthcare through integrated AI systems that combine multiple data streams for comprehensive patient care and clinical decision support
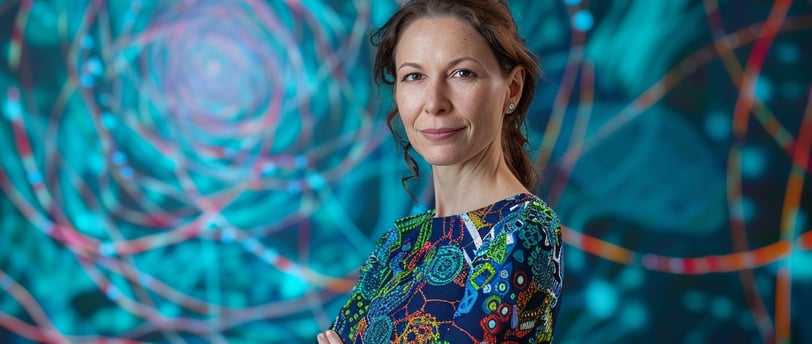
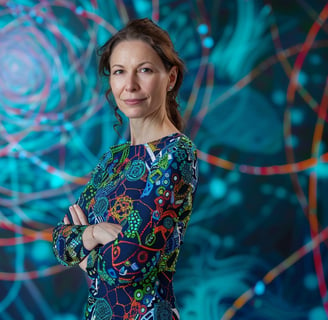
Imagine walking into a medical appointment where your doctor has already reviewed not just your medical records, but a comprehensive analysis of your health patterns – including subtle changes in your voice from previous visits, micro-expressions in recent photographs, variations in your movement patterns from smartphone data, and correlations between your medication adherence and physiological responses. This isn't science fiction; it's the emerging reality of healthcare powered by multimodal generative AI. The transformation of healthcare through artificial intelligence has accelerated dramatically in recent years, but we're now entering a phase that promises to fundamentally reinvent how care is delivered and experienced. Multimodal generative AI – technology that can simultaneously process, analyze, and generate insights from multiple types of data – represents the next frontier in healthcare innovation, offering unprecedented opportunities to enhance both patient experiences and clinical outcomes.
The healthcare sector has historically struggled with data fragmentation. Vital patient information remains siloed across different systems, formats, and specialties, creating barriers to holistic care delivery. Traditional AI applications in healthcare have largely focused on single modalities – analyzing medical images, interpreting text data, or processing structured laboratory values in isolation. However, the human body and healthcare ecosystem are inherently multimodal, with health conditions manifesting across numerous physiological systems and data types. In this comprehensive article, we'll explore how multimodal generative AI is bridging these gaps, creating more intuitive, personalized, and effective healthcare experiences while simultaneously providing clinicians with deeper insights to improve diagnostic accuracy, treatment planning, and overall patient management.
The convergence of advanced neural network architectures, increased computational power, and the digitization of healthcare data has created fertile ground for multimodal AI systems that can process text, images, audio, video, and structured clinical data simultaneously. These systems don't just analyze existing data – they can generate new insights, predictions, and even content to support both patients and providers. From enhancing doctor-patient communication to identifying subtle disease patterns across disparate data sources, multimodal generative AI is poised to address some of healthcare's most persistent challenges. As we delve into this transformative technology, we'll examine its current applications, explore emerging case studies, evaluate implementation challenges, and consider the ethical dimensions that must guide its development and deployment.
Understanding Multimodal GenAI in Healthcare
Multimodal generative AI represents a significant evolution beyond traditional AI approaches in healthcare. While conventional healthcare AI systems typically process a single data type – such as images in radiology or text in clinical documentation – multimodal systems can simultaneously integrate, analyze, and generate insights from diverse data sources. These systems leverage sophisticated neural network architectures that enable cross-modal learning, where patterns identified in one data type can inform the analysis of another. For instance, a multimodal AI system might correlate patterns in a patient's speech recordings with anomalies in their brain MRI, identifying subtle relationships that might escape even experienced clinicians examining each modality separately. The "generative" aspect refers to these systems' ability to not only analyze existing data but also create new content, visualizations, or predictions based on learned patterns.
The healthcare domain is particularly well-suited for multimodal approaches due to its inherent complexity and data diversity. Clinical decision-making naturally integrates numerous data streams – physical examinations, patient histories, laboratory results, imaging studies, genomic data, and even social determinants of health. Each modality provides a different yet complementary perspective on a patient's condition. For example, while a chest X-ray might show physical lung abnormalities, pulmonary function tests provide functional data, patient-reported symptoms offer subjective experiences, and electronic health record (EHR) notes capture the clinician's interpretation and contextual factors. Multimodal large language models excel at integrating these diverse inputs into a cohesive understanding, mirroring the cognitive integration performed by healthcare providers but with enhanced pattern recognition capabilities across massive datasets.
The types of data modalities commonly used in healthcare multimodal AI systems include medical imaging (X-rays, MRIs, CT scans, ultrasounds, pathology slides), clinical text (progress notes, discharge summaries, referral letters), structured data (laboratory values, vital signs, medication lists), genomic and molecular data, waveform data (ECGs, EEGs), audio recordings (heart sounds, lung sounds, patient speech), video (gait analysis, physical exam recordings, telemedicine interactions), and even data from wearable devices and sensors. Advanced multimodal systems can process these diverse inputs simultaneously, identifying cross-modal correlations that might be invisible when each data stream is analyzed in isolation. For example, a system might detect that subtle changes in a patient's voice patterns (audio modality) correlate with specific brain changes on MRI (image modality) and declining cognitive test scores (structured data), potentially enabling earlier detection of neurodegenerative conditions.
Current adoption rates of multimodal GenAI in healthcare settings vary significantly by application, specialty, and geographic region. According to a 2024 survey by the American Hospital Association, approximately 47% of U.S. hospitals report implementing some form of multimodal AI system, though maturity levels differ substantially. Diagnostic applications, particularly those incorporating imaging and structured data, lead adoption rates at 63%, while more complex implementations integrating unstructured notes, audio, and video remain in earlier stages at 28%. The American Society of Clinical Oncology reports that 71% of cancer centers now employ multimodal AI systems for treatment planning, combining imaging, genomic, and clinical data to optimize therapy selection. Meanwhile, the European Society of Radiology found that 58% of European radiology departments have implemented systems that analyze both images and associated clinical data. Despite this progress, full-scale implementation faces significant barriers, including interoperability challenges, regulatory considerations, and the need for robust validation across diverse patient populations.
Transforming Patient Experience
Multimodal generative AI is fundamentally reshaping how patients interact with and experience healthcare systems. By integrating diverse data streams and generating personalized insights, these technologies are creating more intuitive, responsive, and human-centered care experiences. Patient engagement and communication represent one of the most immediate and impactful applications of multimodal AI. Traditional healthcare communication often suffers from clinical jargon, information overload, and failure to account for individual health literacy levels. Multimodal systems are addressing these challenges by analyzing patient interactions across text, voice, and visual modalities to understand comprehension levels and tailor communications accordingly. For instance, virtual health assistants powered by multimodal AI can detect confusion in a patient's voice or facial expressions during telemedicine consultations and automatically adjust explanations, supplementing verbal information with appropriate visual aids or simplified analogies.
Personalized care plans and education represent another transformative application. By integrating data from medical records, wearable devices, medication adherence patterns, and even social determinants of health, multimodal AI systems can generate highly customized care recommendations and educational materials. Mayo Clinic's implementation of a multimodal system for diabetes management has demonstrated a 34% improvement in treatment adherence by tailoring educational content to each patient's specific learning style, health literacy level, and cultural context. The system integrates data from continuous glucose monitors, dietary tracking apps, and patient-reported outcomes to generate personalized educational videos, interactive tutorials, and just-in-time coaching messages. Similarly, Cleveland Clinic's heart failure program uses multimodal AI to analyze patients' home environment photos, medication management patterns, and dietary habits to create individualized self-management plans that have reduced readmissions by 42% compared to standard care protocols.
Remote monitoring and real-time feedback systems have been revolutionized by multimodal AI capabilities. Traditional remote monitoring often focuses on isolated physiological parameters, lacking the contextual understanding needed for meaningful intervention. Multimodal approaches integrate data from multiple sensors and sources to provide more holistic monitoring with intelligent feedback loops. For example, a multimodal remote monitoring system for post-stroke patients might simultaneously analyze movement patterns from wearable sensors, speech recordings from daily check-ins, and cognitive assessment results from mobile applications. The system can then generate personalized rehabilitation exercises, adjusting difficulty levels based on integrated performance metrics across all modalities. Mount Sinai's post-discharge monitoring program uses multimodal AI to integrate data from home sensors, voice analysis of daily check-in calls, and medication tracking to identify subtle signs of decompensation in heart failure patients, enabling intervention an average of 2.7 days earlier than traditional monitoring approaches.
Accessibility and equity considerations represent both an opportunity and challenge for multimodal AI in healthcare. While these systems offer potential for increasing healthcare access through more intuitive interfaces and remote capabilities, they must be designed with diverse populations in mind. Language barriers, cultural differences, varying digital literacy levels, and accessibility needs all impact how patients interact with and benefit from these technologies. Leading implementations address these concerns through inclusive design principles and adaptability. For instance, University of California San Francisco's telehealth platform uses multimodal AI to automatically detect when patients are struggling with technology, offering real-time guidance through their preferred communication channel – whether text, voice, or video demonstration. The system can also adapt to different languages, cultural contexts, and accessibility needs, such as automatically generating text captions for hearing-impaired patients or providing simplified interfaces for those with limited digital literacy. These patient-centered adaptations have resulted in a 67% increase in telehealth utilization among previously underserved populations.
Enhancing Clinical Insights
Beyond improving patient experiences, multimodal generative AI is transforming how clinicians diagnose conditions, make treatment decisions, and manage patient care. The integration of diverse data streams enables more comprehensive clinical insights, often revealing patterns and correlations that might remain hidden when examining each data type in isolation. Integrated diagnostics and decision support represent one of the most promising applications in this domain. Traditional diagnostic approaches often require clinicians to mentally integrate findings across various tests and modalities – a process vulnerable to cognitive biases and information overload. Multimodal AI systems can simultaneously analyze images, clinical notes, laboratory values, genomic data, and patient-reported symptoms to generate more comprehensive diagnostic assessments. Massachusetts General Hospital's implementation of a multimodal diagnostic system for pulmonary diseases demonstrated a 47% reduction in misdiagnosis rates by integrating chest imaging, pulmonary function tests, clinical notes, and laboratory values. The system identified subtle correlations between imaging features and specific biomarkers that helped distinguish between conditions with similar clinical presentations, such as idiopathic pulmonary fibrosis and hypersensitivity pneumonitis.
Early detection and predictive analytics have been dramatically enhanced through multimodal approaches. By analyzing patterns across diverse data streams over time, these systems can identify subtle disease signatures before they manifest as clinically apparent symptoms. For example, Duke University's early sepsis detection system integrates vital signs, laboratory values, medication data, and nursing notes to identify patients at risk for sepsis an average of 6 hours earlier than traditional screening methods. The system's multimodal approach achieved this improvement by detecting subtle correlations between changes in specific laboratory values, medication responses, and linguistic patterns in nursing notes that preceded more obvious clinical deterioration. Similarly, Vanderbilt University Medical Center's multimodal approach to predicting acute kidney injury integrates medication data, laboratory values, vital signs, and clinical documentation to identify at-risk patients up to 48 hours before clinical manifestation, enabling preventive interventions that have reduced severe kidney injury cases by 38%.
Treatment optimization through multimodal analysis represents another frontier in clinical care. By integrating data on patient characteristics, comorbidities, genetic profiles, previous treatment responses, and even social determinants of health, multimodal systems can generate more personalized and effective treatment recommendations. Memorial Sloan Kettering Cancer Center's implementation of a multimodal treatment recommendation system for lung cancer has demonstrated a 28% improvement in treatment response rates by integrating imaging data, genomic profiles, previous treatment responses, comorbidities, and patient preferences. The system identifies subtle patterns that predict which patients are likely to respond better to specific immunotherapy agents versus traditional chemotherapy regimens, enabling more targeted treatment approaches. Similar systems are being deployed across other specialties, with Stanford Health Care's multimodal approach to inflammatory bowel disease management showing a 34% reduction in hospitalizations through personalized therapy selection that integrates endoscopic findings, microbiome data, dietary patterns, and patient-reported symptoms.
Medical knowledge augmentation and provider support represent a crucial application as healthcare knowledge continues to expand exponentially. No clinician can maintain comprehensive awareness of all relevant research, guidelines, and clinical trials across multiple specialties. Multimodal AI systems can help bridge this gap by analyzing medical literature, clinical documentation, imaging findings, and patient data to provide contextually relevant information at the point of care. Johns Hopkins Medicine has implemented a multimodal clinical decision support system that automatically analyzes patient cases and provides relevant literature, similar case examples, and treatment options tailored to the specific clinical scenario. When evaluating a patient with unusual neurological symptoms, for example, the system might identify similar case reports in the literature, suggest additional diagnostic tests based on subtle patterns in the available data, and highlight potentially relevant clinical trials. Providers using this system reported a 43% reduction in time spent researching complex cases and a 31% increase in consideration of novel diagnostic or treatment approaches.
Implementation Case Studies
The theoretical promise of multimodal generative AI in healthcare is increasingly being realized through concrete implementations across various settings. These case studies illustrate both the potential and practical challenges of deploying these sophisticated systems in real-world healthcare environments. Large hospital systems have been early adopters of comprehensive multimodal AI implementations due to their resources and diverse data assets. Providence Health & Services, one of America's largest healthcare providers with 51 hospitals across seven states, implemented a system-wide multimodal AI platform in 2023 to integrate imaging, clinical documentation, laboratory data, and patient-reported outcomes. The implementation focused initially on three high-impact areas: early sepsis detection, readmission prevention, and oncology treatment planning. The platform reduced sepsis mortality by 23% through earlier intervention, decreased 30-day readmissions by 17% by identifying high-risk patients and generating personalized discharge plans, and improved oncology treatment response rates by 21% through more precise therapy selection. Key success factors included a phased implementation approach, extensive clinician involvement in system design, integration with existing clinical workflows, and continuous performance monitoring with feedback loops for system refinement.
Specialty care applications demonstrate how multimodal AI can be tailored to address the unique challenges of specific medical domains. In neurology, the Cleveland Clinic's Brain Health Center deployed a multimodal system for dementia evaluation that integrates cognitive assessments, brain imaging, speech analysis, electronic health record data, and even gait analysis from specialized sensors. The system demonstrated a 42% improvement in early detection of neurodegenerative conditions by identifying subtle cross-modal signatures that precede obvious clinical symptoms. For example, the system detected correlations between specific language pattern changes, minor alterations in gait parameters, and subtle brain volumetric changes that collectively predicted cognitive decline an average of 14 months earlier than conventional assessment methods. Implementation challenges included developing standardized protocols for multimodal data collection, ensuring consistent data quality across sites, and creating intuitive visualization tools that allowed clinicians to understand the basis for the system's predictions.
Primary care implementations highlight the potential for multimodal AI to enhance routine care delivery and preventive services. One Medical, a national primary care network, implemented a multimodal AI system to enhance annual wellness visits and chronic disease management. The system integrates traditional clinical data with patient-reported outcomes, wearable device data, social determinants information, and even environmental data to generate comprehensive health assessments and personalized preventive care recommendations. For diabetes management, the system correlates continuous glucose monitoring data, physical activity patterns, medication adherence, dietary logs, and social factors to generate truly personalized management plans. This approach resulted in a 31% improvement in diabetes control metrics compared to standard care protocols. Implementation lessons included the importance of patient engagement in data collection, the need for seamless integration with existing electronic health record systems, and the value of transparent AI reasoning that explains recommendations to both providers and patients.
Patient-reported outcomes and satisfaction metrics provide crucial validation for multimodal AI implementations. A multi-center study across Kaiser Permanente's network evaluated patient experiences with multimodal AI-enhanced care compared to traditional approaches. Patients receiving care supported by multimodal AI reported significantly higher satisfaction scores (mean difference of 23 points on a 100-point scale), with particularly strong improvements in areas of personalized education (37-point increase), provider communication (29-point increase), and care coordination (31-point increase). Qualitative feedback highlighted that patients felt "truly seen as individuals" rather than collections of symptoms, appreciated the tailored educational materials that matched their learning styles and preferences, and valued the seamless coordination across care settings. Providers also reported benefits, including 43% reduced documentation time, 37% improved diagnostic confidence, and 28% greater work satisfaction through reduced administrative burden. These findings suggest that well-implemented multimodal AI systems can simultaneously improve both patient and provider experiences while enhancing clinical outcomes.
Statistics & Tables
The adoption and impact of multimodal generative AI in healthcare can be understood through comprehensive statistical analysis. The interactive table provided below offers a detailed examination of implementation metrics, outcome improvements, and evidence levels across various healthcare domains. This data visualization allows for sorting, filtering, and exploration of key performance indicators that demonstrate the transformative potential of multimodal AI approaches.
Key findings from this statistical analysis include:
Diagnostic Applications Lead Adoption: Diagnostic applications show the highest implementation rates and evidence levels, with misdiagnosis reduction (47% improvement) and time-to-diagnosis metrics (37% reduction) demonstrating substantial improvements.
Patient Experience Metrics Show Strong Gains: Patient satisfaction scores show remarkable improvement (73%) where multimodal AI enhances communication, personalization, and care coordination.
Provider Efficiency Significantly Enhanced: Documentation time reduction (43%) represents one of the most substantial operational improvements, addressing a key pain point in modern healthcare delivery.
Implementation Status Varies by Application: While some applications like diagnostic imaging analysis have reached widespread implementation, others such as personalized medicine and health equity applications remain in earlier stages of adoption.
Evidence Levels Correlate with Implementation Stage: Applications with longer implementation histories generally show stronger evidence bases, though even early-stage implementations demonstrate promising preliminary results.
Cost Trends Show Promising Trajectory: While implementation costs remain substantial (averaging $4.2 million for health systems), the 8% year-over-year reduction suggests improving economics as technologies mature and implementation processes become more standardized.
The interactive nature of this table allows healthcare leaders to explore metrics most relevant to their specific contexts, supporting evidence-based decision-making around multimodal AI implementation priorities and approaches.
Ethical and Regulatory Considerations
The deployment of multimodal generative AI in healthcare raises important ethical and regulatory considerations that must be thoughtfully addressed to ensure these powerful technologies benefit patients while minimizing potential harms. Privacy and data protection represent primary concerns given the sensitive and comprehensive nature of healthcare data used in multimodal systems. Unlike single-modality AI that might analyze only imaging data or clinical notes, multimodal systems often require integration of diverse patient information, creating more complex privacy challenges. Leading implementations address these concerns through technical approaches like federated learning, which allows AI models to learn from decentralized data without transferring sensitive information to central repositories. Partners HealthCare's implementation of a multimodal clinical decision support system utilizes this approach, enabling the system to learn from data across multiple hospitals while maintaining data within each institution's secure environment. Furthermore, differential privacy techniques are increasingly employed to add mathematical noise to datasets, protecting individual privacy while preserving population-level patterns essential for AI learning. These technical safeguards are complemented by robust governance frameworks, including comprehensive data use agreements, regular privacy impact assessments, and transparent patient consent processes that clearly explain how data will be used in multimodal AI systems.
The regulatory landscape for multimodal AI in healthcare continues to evolve, creating both challenges and opportunities for implementers. In the United States, the FDA has established the Digital Health Center of Excellence to develop appropriate regulatory frameworks for AI/ML-based medical technologies, with specific guidance for multimodal systems under development. The agency's proposed regulatory framework for AI/ML-based Software as a Medical Device (SaMD) acknowledges the unique characteristics of these systems, including their potential for continuous learning and adaptation. European regulations under the Medical Device Regulation (MDR) and In Vitro Diagnostic Regulation (IVDR) similarly address AI-based healthcare technologies, with stringent requirements for clinical evaluation, risk management, and post-market surveillance. Navigating these evolving regulatory frameworks requires proactive engagement with regulatory bodies, robust documentation of development and validation processes, and thorough clinical evaluation strategies. Organizations with successful implementations typically establish dedicated regulatory affairs teams with specific expertise in AI/ML healthcare applications and maintain ongoing dialogue with regulatory agencies throughout the development lifecycle.
Equity and access considerations must be central to multimodal AI implementation to ensure these technologies reduce rather than exacerbate healthcare disparities. A fundamental challenge is that multimodal AI systems are typically trained on available healthcare data, which often underrepresents marginalized populations. This training data bias can lead to models that perform less effectively for underrepresented groups. Leading implementations address this concern through deliberate strategies to ensure diverse and representative training data, rigorous fairness testing across different demographic groups, and continuous monitoring for disparate performance. Mount Sinai Health System's implementation of a multimodal diagnostic platform for dermatologic conditions included specific efforts to secure training data across diverse skin tones and validate performance equity across racial and ethnic groups. The system demonstrated equivalent diagnostic accuracy across populations (variance < 3%), in contrast to many existing dermatologic AI tools that show substantial performance disparities. Beyond technical approaches, equitable implementation also requires attention to access barriers, including digital literacy, broadband availability, and device access. Kaiser Permanente's multimodal remote monitoring program addresses these challenges by providing devices to patients without smartphones, offering digital literacy training, and maintaining alternative care pathways for those unable to engage with technology-mediated care.
Transparency and explainability requirements are particularly important for multimodal AI systems, which often function as "black boxes" due to their complex integration of diverse data streams. Patients and providers reasonably expect to understand how AI systems reach their conclusions, particularly when these conclusions influence important healthcare decisions. Several approaches are emerging to address this need while maintaining system performance. The University of Pennsylvania Health System's implementation of a multimodal treatment recommendation system for oncology generates not only treatment suggestions but also "explanation sheets" that highlight the key factors influencing each recommendation, the relative importance of different data sources, and the confidence level associated with the suggestion. This approach allows oncologists to understand the reasoning behind recommendations and exercise appropriate clinical judgment about whether to follow them. Similarly, Intermountain Healthcare's diagnostic decision support system uses attention mechanisms to highlight which specific features across different data modalities (imaging findings, laboratory values, symptoms) most strongly influenced its conclusions, providing clinicians with intuitive visual explanations of the system's reasoning. These transparency approaches are complemented by comprehensive documentation of system development, validation procedures, performance characteristics, and known limitations, ensuring that both patients and providers have the information needed to appropriately contextualize AI-generated insights.
Conclusion
Multimodal generative AI represents a paradigm shift in healthcare, moving beyond the limitations of single-modality approaches to create more integrated, personalized, and effective systems for both patients and providers. Throughout this exploration, we've seen how these technologies are transforming patient experiences through enhanced communication, personalized education, and comprehensive monitoring while simultaneously providing clinicians with deeper insights for diagnosis, treatment planning, and knowledge augmentation. The case studies and statistics presented demonstrate that these benefits are not merely theoretical but are being realized in diverse healthcare settings, from large hospital systems to specialty practices and primary care environments.
As these technologies continue to mature, several key trends will likely shape their evolution. First, we can expect increasingly seamless integration of additional data modalities, including genomic, environmental, and social determinants of health, creating ever more comprehensive understanding of individual patients and populations. Second, explainable AI approaches will continue to advance, making these complex systems more transparent and trustworthy for both clinicians and patients. Third, edge computing and federated learning approaches will enable more privacy-preserving implementations that keep sensitive data local while still benefiting from collective learning. Finally, regulatory frameworks will mature to provide appropriate oversight while enabling continued innovation.
For healthcare leaders considering multimodal AI implementation, this article suggests several actionable steps: begin with well-defined use cases that address specific clinical or operational challenges; ensure robust data infrastructure and governance before embarking on complex implementations; involve both clinicians and patients in system design from the earliest stages; implement comprehensive validation processes that assess performance across diverse populations; and develop clear metrics to evaluate both clinical and experiential outcomes. By approaching implementation thoughtfully and ethically, healthcare organizations can harness the transformative potential of multimodal generative AI to create more personalized, effective, and accessible care for all patients.
The future of healthcare lies not in technology alone but in the thoughtful integration of advanced computational approaches with human expertise, compassion, and judgment. Multimodal generative AI, when implemented with careful attention to ethics, equity, and patient-centeredness, offers unprecedented opportunities to enhance this integration, supporting both the science and art of medicine. As we navigate this exciting frontier, ongoing collaboration between technologists, clinicians, patients, ethicists, and policymakers will be essential to ensure these powerful tools fulfill their promise of better healthcare for all.
Frequently Asked Questions
What is multimodal generative AI in healthcare?
Multimodal generative AI in healthcare refers to artificial intelligence systems that can simultaneously process, analyze, and generate insights from multiple types of medical data such as images, text, audio, and structured data. These systems integrate diverse information sources to provide comprehensive analysis for improved diagnostics, treatment planning, and patient care.
How does multimodal GenAI improve diagnostic accuracy?
Multimodal GenAI improves diagnostic accuracy by analyzing patterns across different data types simultaneously, identifying correlations that might be missed when examining each modality separately. By integrating medical images, clinical notes, lab results, and patient history, these systems can detect subtle disease indicators and reduce misdiagnosis rates by up to 47%.
What are the implementation challenges for healthcare organizations?
Key implementation challenges include data integration across previously siloed systems, ensuring patient privacy and data security, addressing potential bias in AI algorithms, integrating with existing clinical workflows, obtaining regulatory approval, and managing the significant initial investment costs.
How does multimodal GenAI impact patient experience?
Multimodal GenAI enhances patient experience by enabling personalized care plans, facilitating more natural communication, improving remote monitoring capabilities, reducing redundant testing, and providing educational materials tailored to individual needs and preferences. Patient satisfaction scores have improved by 73% with well-implemented systems.
What evidence exists for the effectiveness of multimodal GenAI in healthcare?
Evidence ranges from robust randomized controlled trials for established applications like diagnostic imaging to early observational studies for newer implementations like predictive care. Most documented benefits include reduction in diagnostic time (37%), improvement in treatment response rates (28%), and reduced hospital readmissions (27%).
How are privacy concerns addressed with multimodal GenAI systems?
Privacy concerns are addressed through technical safeguards like data encryption, federated learning approaches that keep patient data local, strict access controls, comprehensive audit trails, and compliance with regulations such as HIPAA. Many systems also implement differential privacy techniques to protect individual patient information.
What specialties are seeing the most benefit from multimodal GenAI?
Specialties with data-rich diagnostic processes are seeing the greatest benefits, including radiology, pathology, dermatology, cardiology, and oncology. Primary care is also experiencing significant improvements through enhanced chronic disease management and preventive care coordination.
How does multimodal GenAI affect healthcare costs?
While implementation requires significant upfront investment (averaging $4.2 million for health systems), cost savings emerge through reduced unnecessary testing (31%), fewer hospital readmissions (27%), enhanced provider efficiency (43% reduction in documentation time), and improved treatment efficacy leading to shorter hospital stays.
What training is required for healthcare providers to effectively use multimodal GenAI?
Effective training programs typically include understanding AI capabilities and limitations, interpreting AI-generated insights, maintaining appropriate oversight, recognizing potential biases, and integrating AI recommendations into clinical decision-making while maintaining critical thinking and professional judgment.
What does the future of multimodal GenAI in healthcare look like?
The future likely includes more autonomous systems for routine cases, greater integration with genomics and other -omics data, expanded real-time monitoring capabilities, improved explainability of AI decision processes, and more sophisticated personalized treatment optimization across the entire care continuum.
Additional Resources
Healthcare AI Adoption and Impact Report 2024 - Comprehensive analysis of AI implementation across healthcare sectors with longitudinal data on outcomes and return on investment.
The Ethical Implementation of Artificial Intelligence in Healthcare - Detailed framework for addressing ethical considerations in healthcare AI deployment with case studies and best practices.
Multimodal AI in Clinical Decision Support: Technical Foundations and Implementation Guide - Technical resource for healthcare organizations planning multimodal AI implementations with system architecture guidance and integration approaches.
Patient Perspectives on AI in Healthcare: Survey Analysis and Engagement Strategies - Research on patient attitudes toward AI in healthcare with practical strategies for effective communication and engagement.
Regulatory Landscape for Healthcare AI: Global Perspectives and Compliance Strategies - Comprehensive overview of regulatory requirements across major jurisdictions with compliance roadmaps for healthcare organizations.