Role of AI Vendors in Deployment of Large Language Models (LLMs)
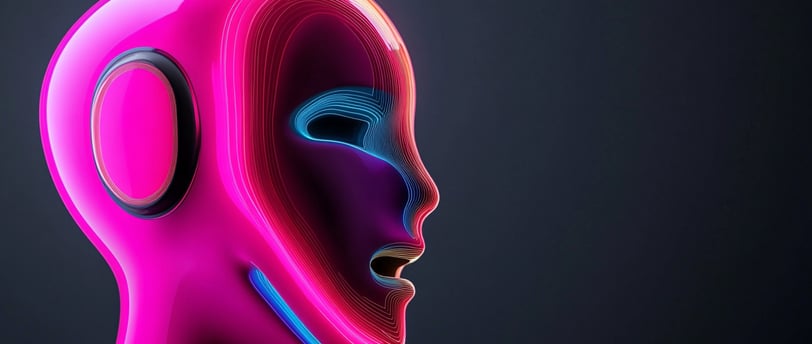
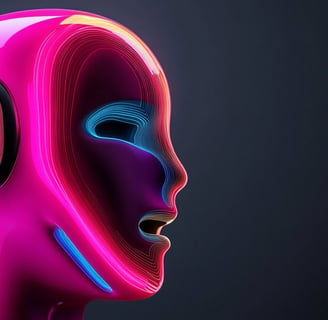
Large Language Models (LLMs) have emerged as a transformative force, revolutionizing how businesses operate and interact with data. These sophisticated models, capable of understanding and generating human-like text, are increasingly being deployed across various industries to enhance efficiency, improve customer experiences, and drive innovation. However, the successful deployment of LLMs is not a straightforward task. It requires meticulous planning, robust infrastructure, and, most crucially, the expertise of AI vendors. This article delves into the critical role AI vendors play in the deployment of LLMs, highlighting their contributions, the challenges they face, and the best practices for ensuring successful implementation.
Understanding Large Language Models (LLMs)
Large Language Models (LLMs) are a subset of artificial intelligence that specialize in natural language processing (NLP). These models are trained on vast amounts of text data to understand, generate, and interpret human language. LLMs have a wide range of applications, from chatbots and virtual assistants to content generation and data analysis. They are designed to mimic human-like conversations, providing coherent and contextually relevant responses. The underlying technology of LLMs is based on deep learning algorithms and transformer architectures, which enable them to process and analyze large datasets efficiently12.
Key Features of LLMs
Text Generation: LLMs can generate human-like text, making them ideal for content creation, such as writing articles, reports, and even creative writing.
Language Translation: These models can translate text from one language to another, breaking down language barriers and facilitating global communication.
Sentiment Analysis: LLMs can analyze text to determine the sentiment behind it, helping businesses understand customer feedback and improve their services.
Data Analysis: LLMs can sift through vast amounts of data to identify patterns, trends, and insights, providing valuable information for decision-making.
Applications of LLMs
LLMs are being deployed across various industries to streamline operations, enhance customer experiences, and drive innovation. Some of the key applications include:
Customer Service: LLMs power chatbots and virtual assistants, providing instant and personalized responses to customer queries.
Content Creation: These models can generate high-quality content, such as blog posts, articles, and reports, saving time and resources.
Data Analysis: LLMs can analyze large datasets to identify trends, patterns, and insights, helping businesses make data-driven decisions.
Healthcare: In the healthcare sector, LLMs assist in diagnosing diseases, developing treatment plans, and improving patient care.
Finance: LLMs are used for fraud detection, risk assessment, and providing personalized financial advice.
The Role of AI Vendors in LLM Deployment
AI vendors play a pivotal role in the successful deployment of LLMs. They provide the necessary infrastructure, expertise, and support to ensure that these models are integrated seamlessly into business operations. Here are some of the key contributions of AI vendors:
Infrastructure and Support
AI vendors offer robust infrastructure and technical support to deploy LLMs. This includes providing cloud-based platforms, scalable computing resources, and secure environments for training and deploying these models. For instance, Amazon Bedrock is a fully managed service that makes LLMs from Amazon and leading AI startups available through an API, allowing businesses to choose the model that best suits their needs1.
Expertise and Consultation
AI vendors bring invaluable expertise and consultation services to the table. They help businesses identify the right use cases for LLMs, develop customized solutions, and provide ongoing support to ensure optimal performance. For example, IBM’s industry-leading AI expertise helps businesses reinvent critical workflows and operations by adding AI to maximize experiences, real-time decision-making, and business value2.
Training and Customization
AI vendors assist in training and customizing LLMs to meet specific business requirements. This involves fine-tuning the models using proprietary data, ensuring that they align with the business’s goals and operational needs. For instance, SAP highlights the importance of training LLMs with industry-relevant and organization-specific data to optimize their performance and effectiveness3.
Compliance and Ethical Considerations
AI vendors ensure that the deployment of LLMs adheres to regulatory compliance and ethical standards. This includes addressing data privacy concerns, mitigating biases in the models, and ensuring transparency and accountability in their operations3.
Challenges Faced by AI Vendors
Despite their critical role, AI vendors face several challenges in the deployment of LLMs. Some of the key challenges include:
Data Privacy and Security
Ensuring the privacy and security of data is a significant challenge. LLMs process vast amounts of textual data, which can include sensitive or personal information. AI vendors must implement robust security measures to protect this data and prevent unauthorized access4.
Bias and Fairness
LLMs can inadvertently perpetuate biases present in the training data. AI vendors must work to mitigate these biases and ensure that the models generate fair and unbiased outputs. This involves continuous monitoring and updating of the models to address any emerging biases4.
Technical Complexity
Deploying LLMs requires substantial technical expertise and resources. AI vendors must navigate the complexities of training, fine-tuning, and maintaining these models, ensuring that they perform optimally and meet business requirements5.
Scalability and Cost
Scaling LLMs to handle large volumes of data and users can be challenging and costly. AI vendors must provide scalable solutions that are cost-effective and efficient, ensuring that businesses can leverage the full potential of these models without incurring excessive costs5.
Best Practices for Successful LLM Deployment
To ensure the successful deployment of LLMs, AI vendors and businesses should follow these best practices:
Clear Use Case Identification
Identifying clear and specific use cases for LLMs is crucial. This involves understanding the business needs and goals, and determining how LLMs can address these requirements effectively3.
Robust Data Management
Effective data management is essential for the successful deployment of LLMs. This includes collecting high-quality data, ensuring data privacy and security, and continuously updating the models with new data to improve their performance3.
Continuous Monitoring and Updating
LLMs require continuous monitoring and updating to ensure optimal performance. This involves regularly evaluating the models’ outputs, addressing any biases or inaccuracies, and fine-tuning the models to meet evolving business needs3.
Collaboration and Partnership
Collaboration between AI vendors and businesses is key to successful LLM deployment. This involves open communication, sharing of expertise, and working together to overcome challenges and achieve common goals6.