Simplifying Complexity: Dimensionality Reduction Demystified
Unraveling the Mystery: How Dimensionality Reduction Can Simplify Your Data Analysis Game In today's fast-paced world of data-driven decision making, businesses are challenged with the task of uncovering gems of insight from vast amounts of intricate and multi-dimensional data.
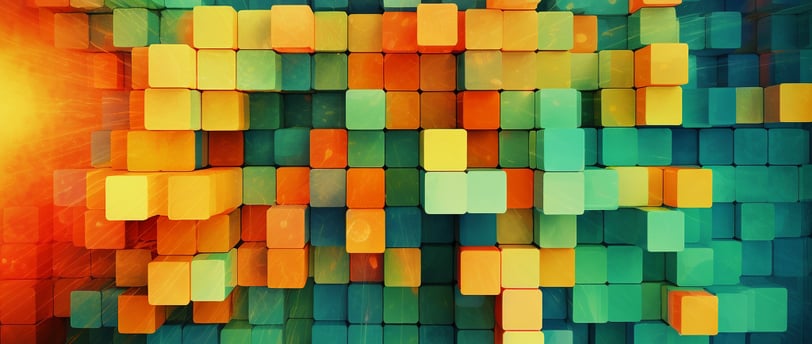
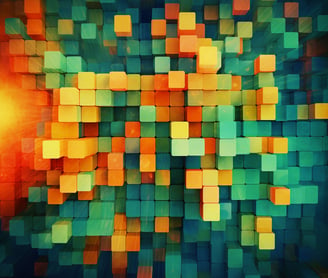
In the ever-evolving world of data-driven decision making, businesses are faced with the daunting task of extracting valuable insights from massive amounts of complex and high-dimensional data. This surge in data volume has led to a significant challenge known as the curse of dimensionality. Dealing with high-dimensional data can hinder businesses from efficiently processing, analyzing, and making meaningful conclusions from their data sets. However, the field of dimensionality reduction offers a solution to this problem, providing businesses with the tools to simplify complexity and extract essential information from their data.
In this article, we will delve deep into the concept of dimensionality reduction, discussing its key concerns, potential benefits for businesses, and insights crucial for the success of the target audience. We will also explore how Datasumi, a leading data analytics company, can help businesses navigate the intricacies of dimensionality reduction and unlock the full potential of their data.
Understanding Dimensionality Reduction
Dimensionality reduction refers to the process of reducing the number of variables or features in a data set while preserving its essential characteristics. The primary goal of dimensionality reduction is to simplify complex data by transforming it into a lower-dimensional space without losing critical information. By eliminating redundant and irrelevant features, dimensionality reduction enables businesses to overcome the challenges associated with high-dimensional data, such as computational inefficiency, overfitting, and difficulty in visualizing and interpreting the data.
Key Concerns in Dimensionality Reduction
While dimensionality reduction offers significant benefits, it is not without its challenges. Several key concerns must be addressed when applying dimensionality reduction techniques to business data:
1. Information Loss: One of the primary concerns in dimensionality reduction is the potential loss of information. When reducing the dimensionality of a data set, there is a risk of discarding valuable information that may be crucial for decision-making processes. It is essential to strike a balance between simplifying the data and preserving its meaningful features.
2. Algorithm Selection: Choosing the right dimensionality reduction algorithm is critical. Various techniques, such as Principal Component Analysis (PCA), t-distributed Stochastic Neighbor Embedding (t-SNE), and Linear Discriminant Analysis (LDA), offer different approaches to dimensionality reduction. Each algorithm has its strengths and limitations, and selecting the appropriate one depends on the specific characteristics of the data and the business's objectives.
3. Computational Complexity: High-dimensional data often requires significant computational resources to process and analyze. Dimensionality reduction techniques should be efficient and scalable to handle large data sets within reasonable timeframes. The computational complexity of the algorithms should be taken into consideration when choosing a dimensionality reduction approach.
Potential Benefits for Businesses
Implementing dimensionality reduction techniques in business processes can yield several benefits, enabling organizations to make better decisions, enhance performance, and improve efficiency. Some of the key advantages include:
1. Improved Data Visualization: High-dimensional data is challenging to visualize, making it difficult to identify patterns and relationships. Dimensionality reduction techniques transform complex data into a lower-dimensional space that can be effectively visualized, enabling businesses to gain insights through graphical representations and make more informed decisions.
2. Enhanced Predictive Models: Dimensionality reduction can help improve the performance of machine learning models by reducing overfitting. By eliminating irrelevant and redundant features, the models become more robust and generalizable, leading to better predictions and more accurate decision-making.
3. Faster Processing and Analysis: Dimensionality reduction reduces the computational burden associated with high-dimensional data. By simplifying the data, businesses can process and analyze their data more efficiently, saving valuable time and resources.
4. Feature Selection and Engineering: Dimensionality reduction techniques aid in identifying the most relevant features in a data set. By focusing on the most informative variables, businesses can streamline their feature selection and engineering processes, leading to more effective models and better business outcomes.
5. Noise Reduction: High-dimensional data often contains noisy or irrelevant features that can hinder analysis and decision-making. Dimensionality reduction techniques help filter out this noise, improving the quality of the data and enabling businesses to focus on the most meaningful patterns and relationships.
Insights Crucial for Success
To successfully apply dimensionality reduction techniques, businesses need to consider several insights that can significantly impact their outcomes:
1. Data Understanding: Before applying dimensionality reduction, businesses must thoroughly understand their data. It is essential to have a clear understanding of the data's structure, relationships, and potential biases. This understanding will guide the selection of appropriate dimensionality reduction techniques and ensure that valuable information is not lost during the process.
2. Evaluation Metrics: The effectiveness of dimensionality reduction techniques should be evaluated using appropriate metrics. Metrics such as explained variance, reconstruction error, and visualization quality can help assess the performance of different algorithms and guide the selection process.
3. Interpretability: While dimensionality reduction simplifies data, it is crucial to retain interpretability. Businesses should aim to ensure that the reduced representation still captures meaningful patterns and relationships that can be easily understood and interpreted by domain experts and decision-makers.
4. Iterative Approach: Dimensionality reduction is not a one-time process. As businesses evolve and new data becomes available, it is essential to periodically re-evaluate and update the dimensionality reduction techniques to capture emerging patterns and changes in the data.
How Datasumi Can Help
Datasumi, a renowned data analytics company, offers a comprehensive suite of solutions to assist businesses in simplifying complexity through dimensionality reduction. Leveraging their expertise in advanced analytics, machine learning, and data visualization, Datasumi provides the following services:
1. Consultation and Assessment: Datasumi's team of experts works closely with businesses to understand their specific data challenges and objectives. They assess the data landscape, identify potential dimensionality reduction opportunities, and develop tailored strategies to simplify complexity and extract valuable insights.
2. Algorithm Selection and Implementation: Datasumi guides businesses in selecting the most appropriate dimensionality reduction algorithms based on their data characteristics and goals. They have a deep understanding of various techniques and their applications, ensuring the optimal algorithm is chosen for each unique scenario. Datasumi's technical team also implements the selected algorithms and integrates them seamlessly into the existing data analytics pipeline.
3. Performance Evaluation: Datasumi provides comprehensive evaluation of the dimensionality reduction techniques applied to the business data. Through rigorous testing and analysis, they assess the effectiveness of the chosen algorithms, measure the impact on data quality and interpretability, and provide actionable recommendations for improvement.
4. Continuous Support and Monitoring: Datasumi understands that dimensionality reduction is an ongoing process. They offer continuous support and monitoring, ensuring that businesses can adapt to evolving data landscapes and make informed decisions based on the latest insights. Datasumi's experts work closely with businesses to refine and optimize dimensionality reduction strategies over time, ensuring maximum value extraction from their data.
Conclusion
In the era of big data, dimensionality reduction has emerged as a crucial tool for simplifying complexity and extracting valuable insights. By reducing the dimensionality of data, businesses can overcome the challenges associated with high-dimensional data sets and make more informed decisions. However, to leverage the full potential of dimensionality reduction, businesses must carefully address the key concerns, understand the potential benefits, and adopt crucial insights for success.
Datasumi, with its expertise in data analytics and dimensionality reduction, can be a valuable partner for businesses seeking to unlock the power of their data. With their consultation, algorithm selection, implementation, and continuous support, Datasumi empowers businesses to navigate the complexities of dimensionality reduction and gain a competitive advantage in today's data-driven landscape.