What is Automated Machine Learning (AutoML)?
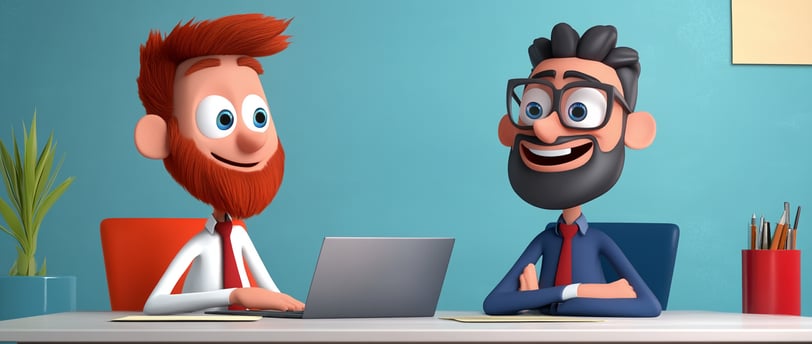
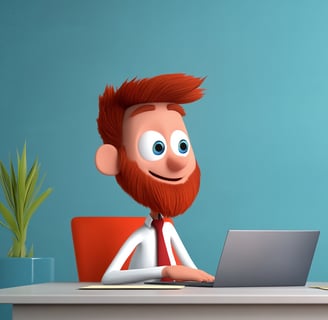
Imagine a world where developing cutting-edge machine learning models doesn't require a Ph.D. in data science. Welcome to the realm of Automated Machine Learning (AutoML), a transformative approach that democratizes machine learning by automating the complex and iterative tasks involved in model development. Whether you're a seasoned data scientist or a novice, AutoML makes it possible to build high-performing models with ease, efficiency, and accuracy. In this article, we'll explore the fundamentals of AutoML, its applications, benefits, and how it's reshaping industries across the board.
Understanding AutoML
AutoML is the process of automating the end-to-end development of machine learning models. It encompasses everything from data preprocessing and feature engineering to model selection, hyperparameter tuning, and deployment. Traditional machine learning often requires manual intervention at each of these stages, making it time-consuming and resource-intensive. AutoML, on the other hand, streamlines these processes, allowing for faster and more efficient model development.
Benefits of AutoML
One of the primary advantages of AutoML is its accessibility. It enables non-experts to create and implement artificial intelligence (AI) systems, making machine learning more approachable for a broader audience. For data scientists and developers, AutoML simplifies workflows and reduces manual labor, allowing them to focus on more strategic tasks. This democratization of machine learning has significant implications for various industries, from healthcare and finance to retail and manufacturing. AutoML tools construct numerous machine learning pipelines to handle the intended task, then identify the optimal choice. Model evaluation and model selection are automated as part of the iterative process of choosing the best model for the job1.
Key Components of AutoML
AutoML systems typically include several key components that work together to automate the machine learning process. These components include:
Data Preprocessing: Automating the cleaning and transformation of raw data into a format suitable for machine learning algorithms.
Feature Engineering: Automatically creating new features from raw data to improve the performance of machine learning models.
Model Selection: Automatically choosing the most appropriate machine learning algorithm for a given task.
Hyperparameter Tuning: Optimizing the parameters of machine learning models to improve their performance.
Model Evaluation: Automatically assessing the performance of machine learning models and selecting the best one.
Applications of AutoML
AutoML has a wide range of applications across various industries. For example, in healthcare, AutoML can be used to develop predictive models for disease diagnosis and treatment outcomes. In finance, it can be employed for fraud detection and risk assessment. In retail, AutoML can help optimize inventory management and customer segmentation. The possibilities are endless, and the impact of AutoML is only beginning to be realized.
Case Studies
Let's look at a couple of case studies to illustrate the power of AutoML:
Healthcare: A hospital uses AutoML to develop a predictive model for patient readmission. By automating the data preprocessing, feature engineering, and model selection processes, the hospital is able to build a highly accurate model that predicts which patients are most likely to be readmitted within 30 days of discharge. This allows the hospital to intervene early and reduce readmission rates, improving patient outcomes and reducing costs.
Finance: A bank employs AutoML to create a fraud detection system. The AutoML system automatically selects the best model and tunes its hyperparameters, resulting in a highly accurate fraud detection model that can identify suspicious transactions in real-time. This helps the bank prevent fraudulent activities and protect its customers' assets.
The Future of AutoML
The future of AutoML is bright, with ongoing research and development focused on improving its capabilities and expanding its applications. As AutoML continues to evolve, we can expect to see even more powerful and accessible tools that make machine learning available to an even wider audience. This will drive innovation and enable businesses to solve complex problems more efficiently.
Conclusion
AutoML is a game-changer in the world of machine learning, making it possible for anyone to build high-performing models with ease. By automating the complex and iterative tasks involved in model development, AutoML democratizes machine learning and opens up new possibilities for innovation. Whether you're a data scientist, a developer, or a business leader, AutoML has the potential to transform the way you approach machine learning and drive meaningful results. So, are you ready to embrace the future of machine learning with AutoML?
FAQ Section
What is AutoML? AutoML, or Automated Machine Learning, is the process of automating the end-to-end development of machine learning models. It includes data preprocessing, feature engineering, model selection, hyperparameter tuning, and model evaluation.
Who can benefit from AutoML? AutoML benefits both non-experts and experienced data scientists. It makes machine learning accessible to those with limited expertise while streamlining workflows for professionals.
What are the key components of AutoML? The key components of AutoML include data preprocessing, feature engineering, model selection, hyperparameter tuning, and model evaluation.
How does AutoML differ from traditional machine learning? AutoML automates the time-consuming and iterative tasks involved in model development, making it faster and more efficient compared to traditional machine learning, which often requires manual intervention at each stage.
What are some applications of AutoML? AutoML has applications in healthcare, finance, retail, manufacturing, and more. It can be used for predictive modeling, fraud detection, risk assessment, inventory management, and customer segmentation.
Can AutoML replace data scientists? While AutoML automates many tasks, it does not replace the need for data scientists. Instead, it complements their work by allowing them to focus on more strategic tasks and innovative projects.
What are the benefits of using AutoML? Benefits include increased accessibility, efficiency, and accuracy in model development. AutoML enables faster deployment of machine learning models and allows organizations to leverage their existing talent without hiring additional data scientists.
How does AutoML impact different industries? AutoML impacts various industries by making machine learning more accessible and efficient. It enables organizations to solve complex problems, drive innovation, and improve decision-making processes.
What is the future of AutoML? The future of AutoML is promising, with ongoing research focused on improving its capabilities and expanding its applications. As AutoML continues to evolve, we can expect to see more powerful and accessible tools that drive innovation and efficiency.
How can I get started with AutoML? To get started with AutoML, you can explore various tools and platforms that offer automated machine learning capabilities. Many of these tools provide user-friendly interfaces and resources to help you build and deploy machine learning models with ease.
Additional Resources
AutoML Overview: For a comprehensive overview of AutoML, you can refer to the AutoML Wikipedia page 2.
AutoML Tools: To learn more about specific AutoML tools and platforms, you can visit the AutoML.org website 3.